Reconstruction of structured illumination microscopy with an untrained neural network
OPTICS COMMUNICATIONS(2023)
摘要
Structured illumination microscopy (SIM) is one of super-resolution optical microscopic techniques, and it has been widely used in biological research. In this paper, a physics-driven deep image prior framework for super-resolution reconstruction of SIM (entitled DIP-SIM) is proposed. DIP-SIM does not rely on a large number of labeled data, and the output becomes more interpretable due to the intrinsic constraint of a physical model. Both the simulation and experiment verify that DIP-SIM can reconstruct a super-resolution image with a quality comparable to conventional SIM. Of note, it allows for super-resolution reconstruction from three raw images for two-orientation SIM and four raw images for three-orientation SIM, and hence it has a much faster imaging speed and lower photobleaching compared with the traditional SIM. We can envisage that the proposed method can be applied to chemistry and biomedical fields, etc.
更多查看译文
关键词
Structured illumination microscopy,Deep learning,Neural network,Super-resolution,Image reconstruction
AI 理解论文
溯源树
样例
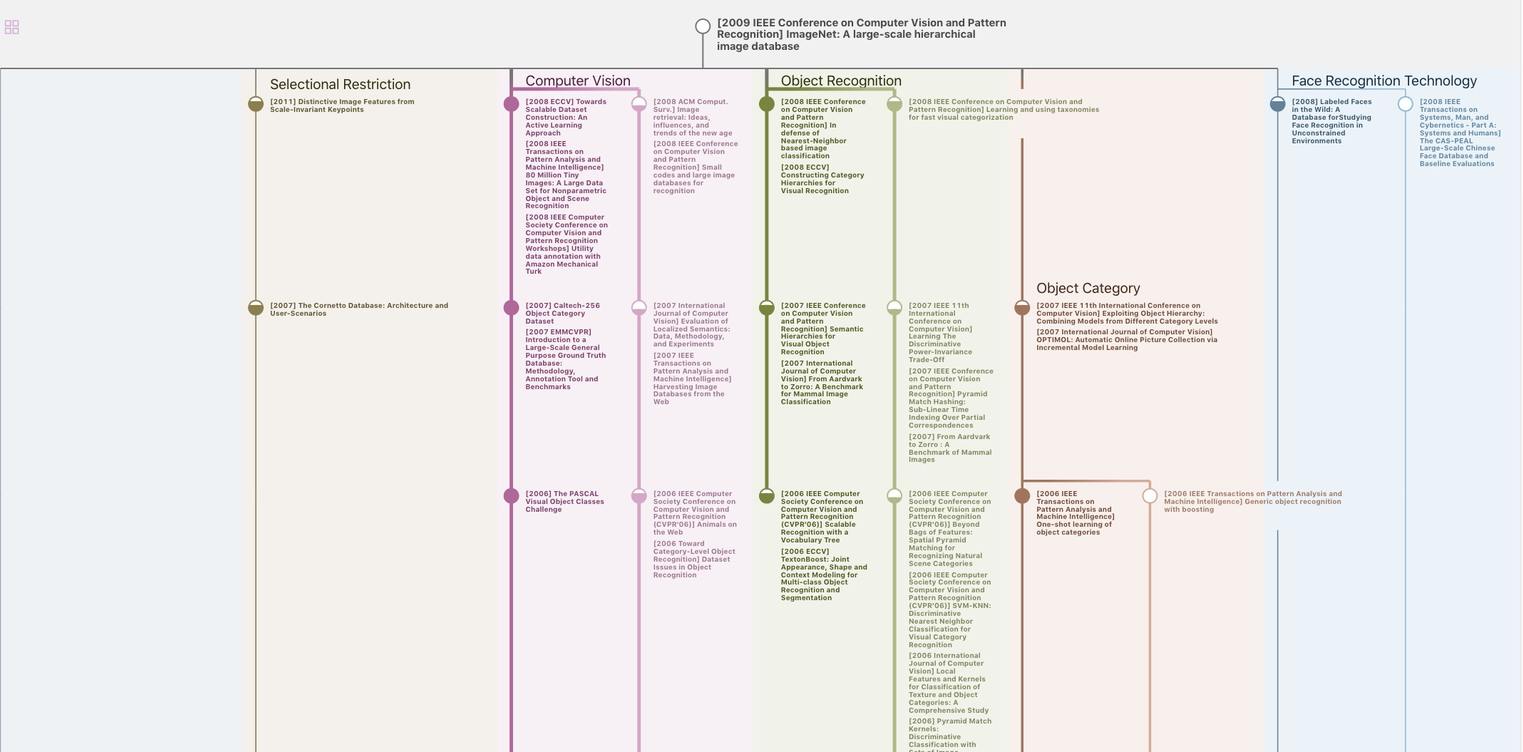
生成溯源树,研究论文发展脉络
Chat Paper
正在生成论文摘要