Adversarial Attack and Defense on Graph Data: A Survey
IEEE Transactions on Knowledge and Data Engineering(2023)
摘要
Deep neural networks (DNNs) have been widely applied to various applications, including image classification, text generation, audio recognition, and graph data analysis. However, recent studies have shown that DNNs are vulnerable to adversarial attacks. Though there are several works about adversarial attack and defense strategies on domains such as images and natural language processing, it is still difficult to directly transfer the learned knowledge to graph data due to its representation structure. Given the importance of graph analysis, an increasing number of studies over the past few years have attempted to analyze the robustness of machine learning models on graph data. Nevertheless, existing research considering adversarial behaviors on graph data often focuses on specific types of attacks with certain assumptions. In addition, each work proposes its own mathematical formulation, which makes the comparison among different methods difficult. Therefore, this review is intended to provide an overall landscape of more than 100 papers on adversarial attack and defense strategies for graph data, and establish a unified formulation encompassing most graph adversarial learning models. Moreover, we also compare different graph attacks and defenses along with their contributions and limitations, as well as summarize the evaluation metrics, datasets and future trends. We hope this survey can help fill the gap in the literature and facilitate further development of this promising new field
1
.
更多查看译文
关键词
Adversarial attack,adversarial defense,adversarial learning,graph data,graph neural networks
AI 理解论文
溯源树
样例
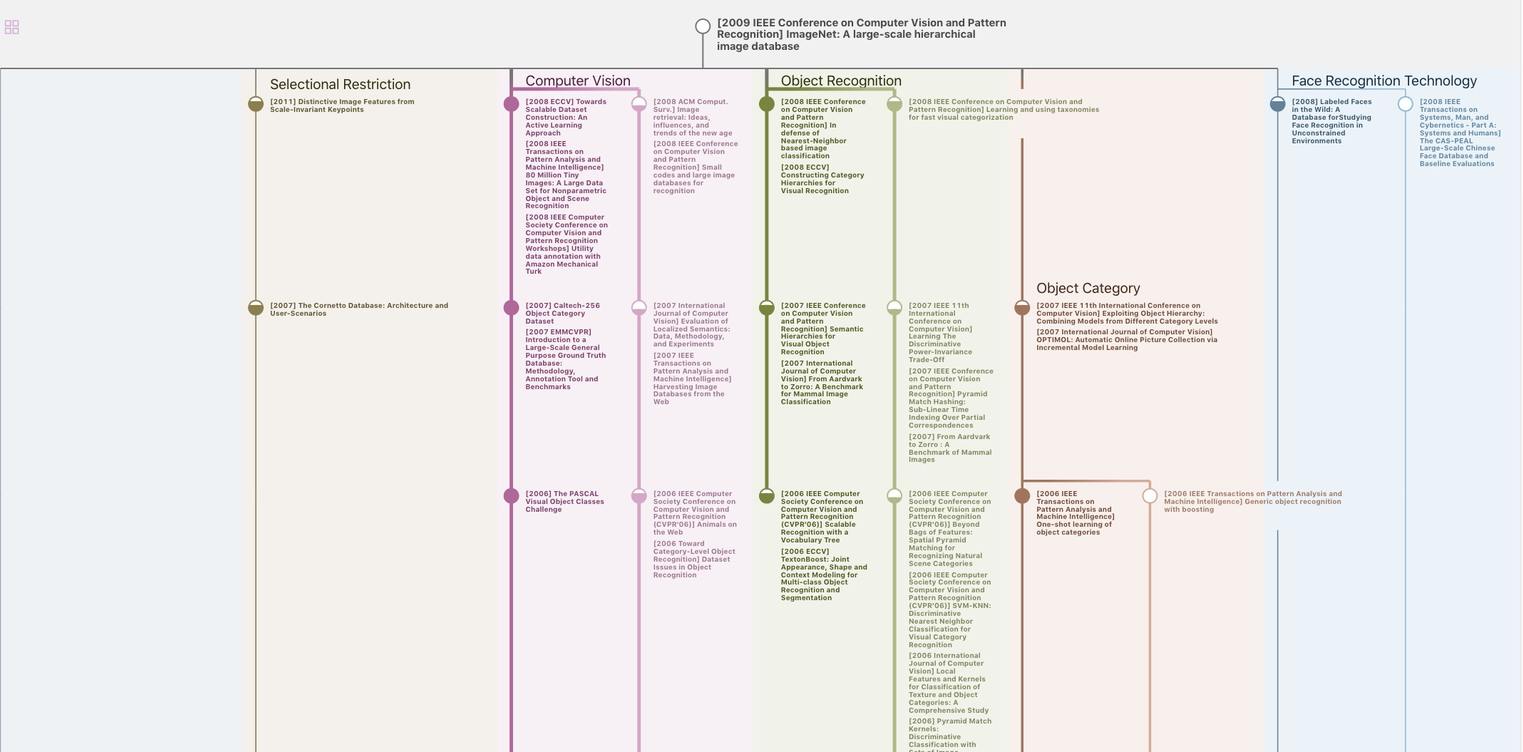
生成溯源树,研究论文发展脉络
Chat Paper
正在生成论文摘要