Fast flow field prediction of hydrofoils based on deep learning
Ocean Engineering(2023)
摘要
Conventionally, the flow field over the hydrofoil is solved by computational fluid dynamics (CFD), which is a computationally expensive task. As an alternative, deep learning (DL) approximates the flow field without solving the complicated Navier-Stokes (NS) equations. Consequently, this paper proposes a data-driven methodology using Convolutional Neural Networks (CNN) for hydrofoils performance prediction, which automatically detects fundamental features and accurately predicts the flow field of the hydrofoil. The CFD simulation is used to generate the dataset for neural network training and testing. The dataset containing hydrofoil geometry and operating conditions is fed into the CNN to approximate the model for predicting the flow field. Furthermore, we use novel attention gates (AGs) that concentrate on hydrofoils of different shapes and sizes. Models trained with AGs not only learn to restrain unrelated features of the input information, but also emphasize significant features useful for flow field prediction. In addition, the effects of various hyperparameters of models on convergence have been extensively investigated. The experimental results demonstrate that the flow field over a hydrofoil can be obtained within 0.5 s, with a mean relative error of less than 1.5%.
更多查看译文
关键词
fast flow field prediction,hydrofoils,learning
AI 理解论文
溯源树
样例
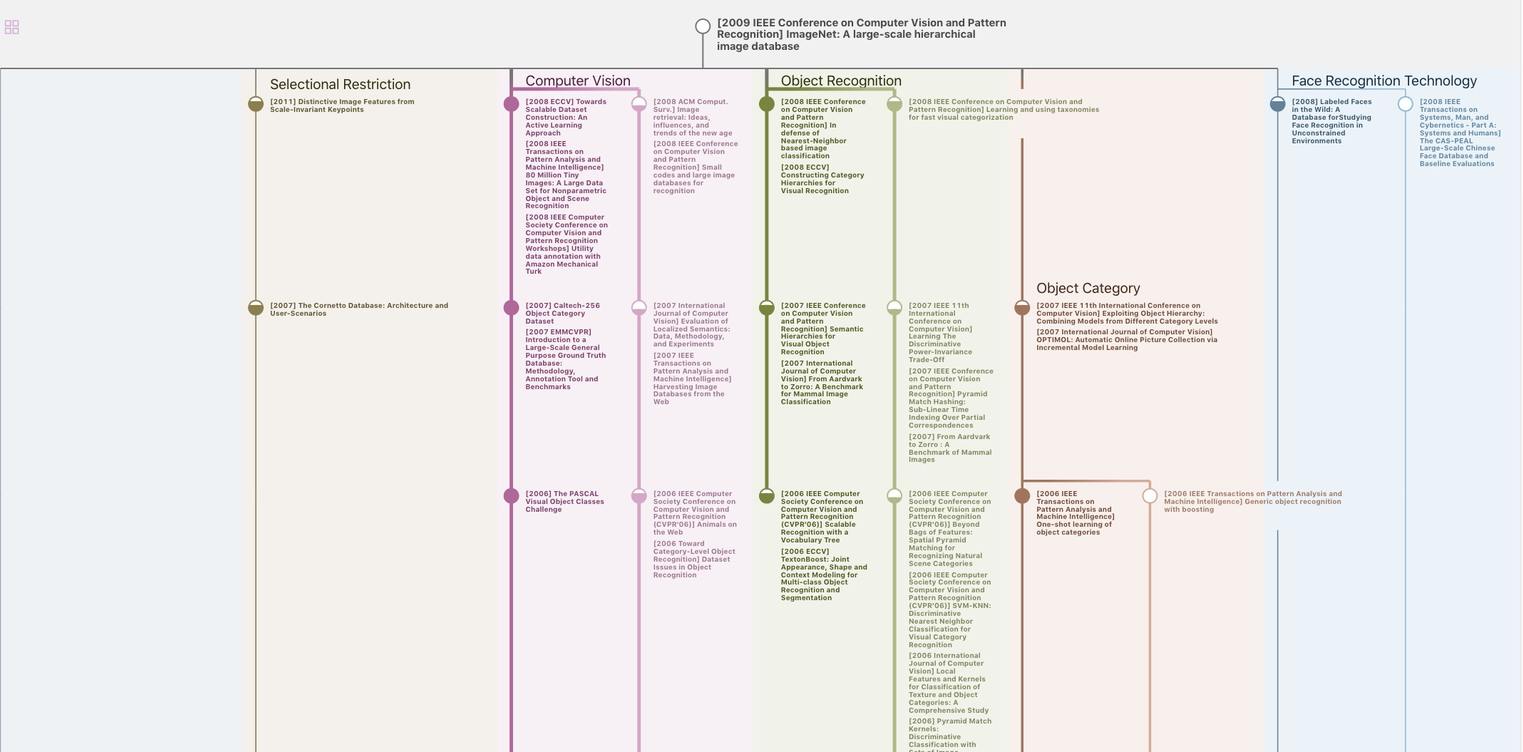
生成溯源树,研究论文发展脉络
Chat Paper
正在生成论文摘要