Lifetime prediction of electronic devices based on the P-stacking machine learning model
Microelectronics Reliability(2023)
摘要
Nowadays the data-driven artificial intelligence (AI) and machine learning (ML) provide novel approaches for the effective lifetime prediction of the electronic devices with complicated mechanisms and multiple controlling factors. However, the existing ML prediction models cannot process both high accuracy and high efficiency at the meantime. To address this problem, this paper proposes the new P-Stacking ML algorithm model, which combines the Pearson correlation analysis and the Stacking multi-model fusion. The Pearson correlation analysis is first applied to select the multiple base learner models with weak correlations for the following Stacking multi-model fusion. Two neutral network layers are built to perform the Stacking algorithm. In this work, two distinct types of electronic devices are utilized to verify the effectiveness of the P-Stacking ML model, which are the IGBT devices and the lithium-ion batteries respectively. For the IGBT lifetime prediction, the results have shown that compared with the long-term and short-term memory neural network (LSTM) model in the previous literature, the mean square error (MSE) of the P-Stacking ML model is improved by 6 %, and the average model training time is reduced by 90 %. Moreover, for the lithium batteries, the lifetime prediction accuracy of the P-Stacking model is increased by 65 %, and the training time is decreased by 90 %. The results demonstrated that the P-Stacking ML model can significantly improve both the prediction accuracy and efficiency simultaneously.
更多查看译文
关键词
Stacking algorithm, Pearson correlation analysis, Machine learning, IGBT device, Lithium -ion battery, Lifetime prediction
AI 理解论文
溯源树
样例
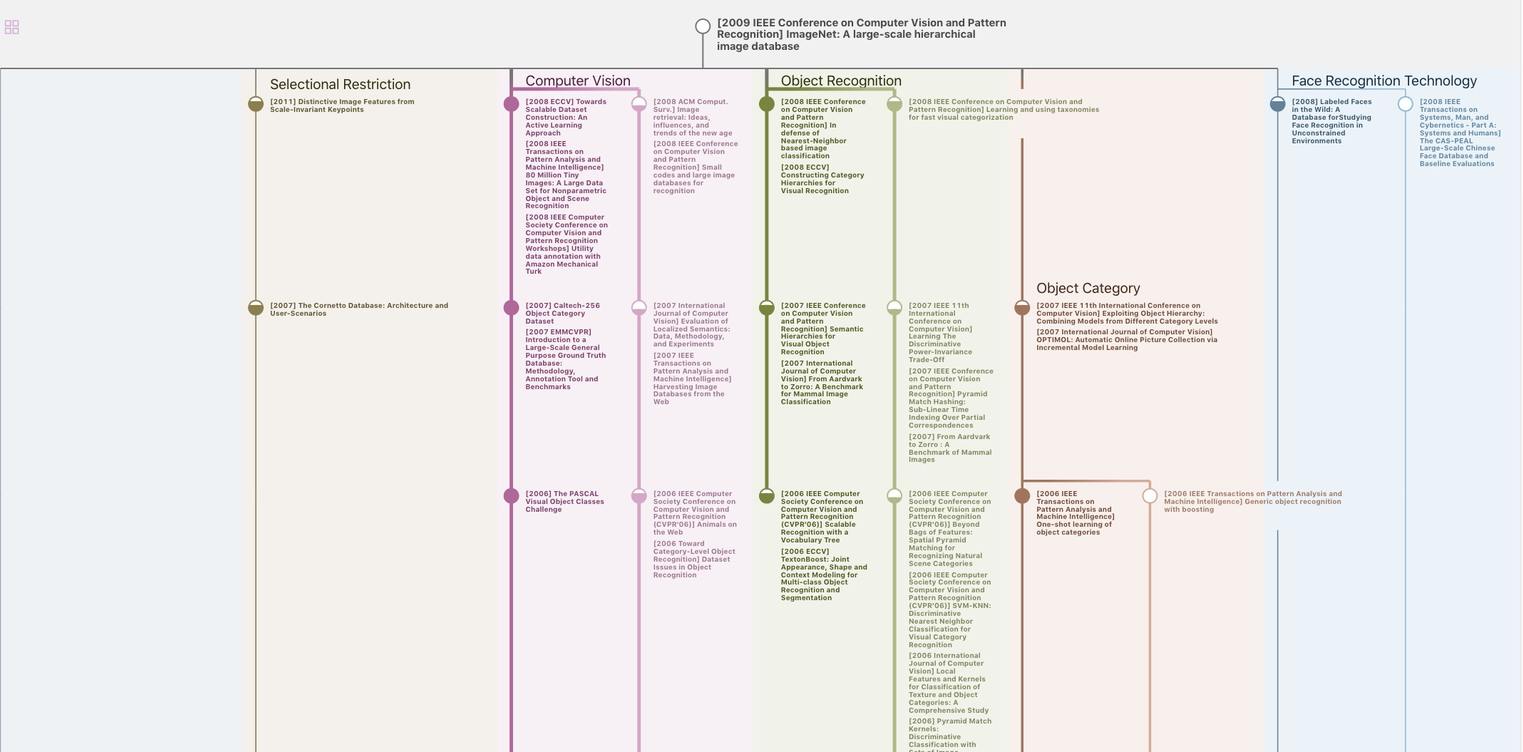
生成溯源树,研究论文发展脉络
Chat Paper
正在生成论文摘要