Machine learning prediction of compressive strength of concrete with resistivity modification
Materials Today Communications(2023)
摘要
Machine learning techniques can predict the compressive strength of cement-based materials with good accuracy and learning capacity. Traditional compressive strength prediction according to machine learning techniques such as the support vector machine (SVM), decision tree, and Gaussian regression are normally based on the mix proportion of concrete compositions. Resistivity can realize the long-term, real-time and in-situ monitoring of compressive strength of the concrete structures. Therefore, electrical resistivity is regarded as a key nondestructive testing parameter to improve the accuracy of the compressive strength prediction model according to machine learning techniques in this study. When the resistivity was taken into consideration as an input variable accounting for 0.166, the fitting degree of the compressive strength in the decision trees model is increased from 0.77 to 0.79. In the SVM model, the fitting degree remains 0.79, the RMSE decreases from 8.490 to 8.335, which indicates the reliability is improved. The fitting degree in the Gaussian model model is increased from 0.81 to 0.82. As a new parameter variable, the accuracy of the compressive strength prediction model modified with electrical resistivity can be significantly increased. Therefore, the nondestructive testing method can be combined with machine learning techniques to promote the development of civil engineering building structure monitoring, diagnosis and facilitate the development of intelligent buildings through data-driven approaches.
更多查看译文
关键词
Compressive strength,Machine learning,Cement-based materials,Electrical resistivity
AI 理解论文
溯源树
样例
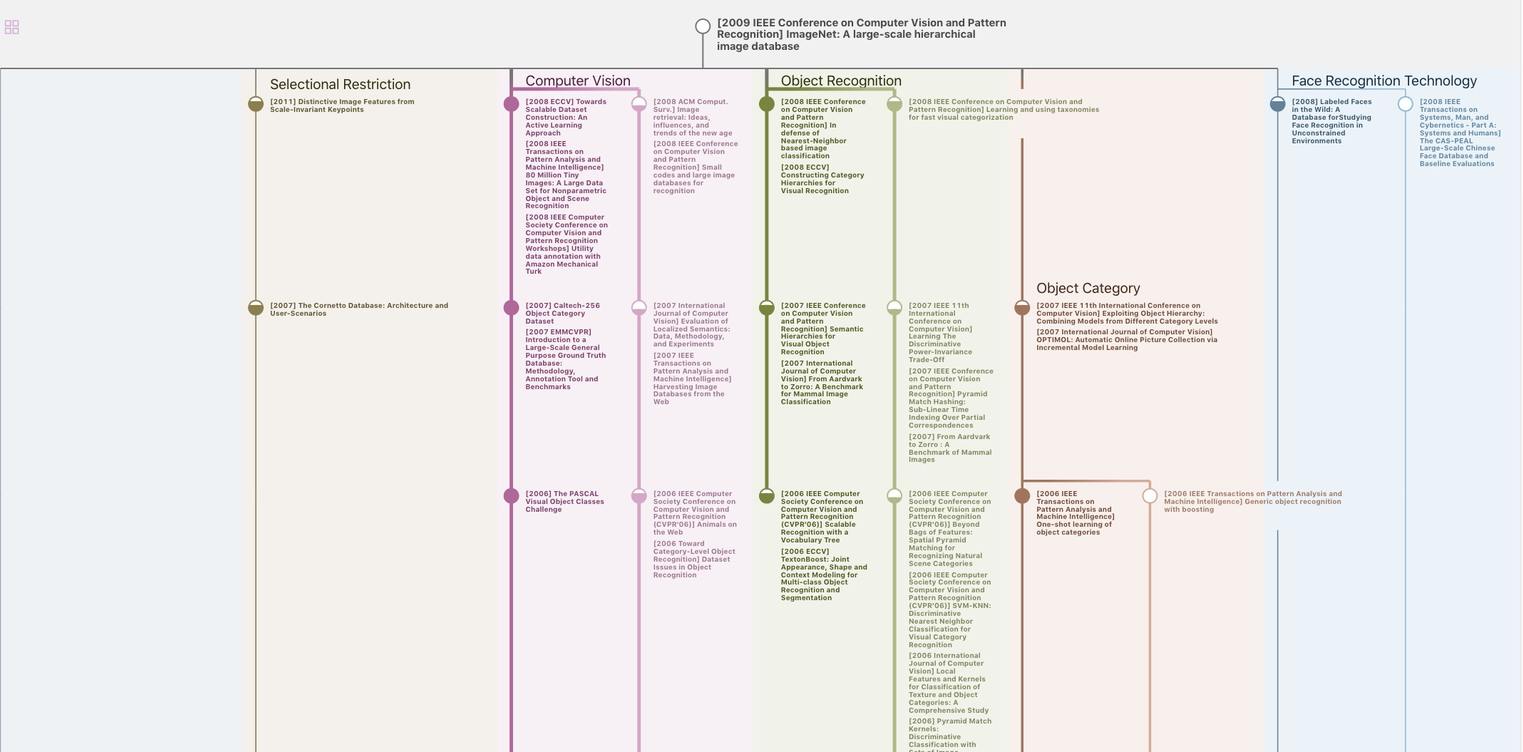
生成溯源树,研究论文发展脉络
Chat Paper
正在生成论文摘要