Bridge damage identification under varying environmental and operational conditions combining Deep Learning and numerical simulations
Mechanical Systems and Signal Processing(2023)
摘要
This work proposes a novel supervised learning approach to identify damage in operating bridge structures. We propose a method to introduce the effect of environmental and operational conditions into the synthetic damage scenarios employed for training a Deep Neural Network, which is applicable to large-scale complex structures. We apply a clustering technique based on Gaussian Mixtures to effectively select Q representative measurements from a long-term monitoring dataset. We employ these measurements as the target response to solve various Finite Element Model Updating problems before generating different damage scenarios. The synthetic and experimental measurements feed two Deep Neural Networks that assess the structural health condition in terms of damage severity and location. We demonstrate the applicability of the proposed method with a real full-scale case study: the Infante Dom Henrique bridge in Porto. A comparative study reveals that neglecting different environmental and operational conditions during training detracts the damage identification task. By contrast, our method provides successful results during a synthetic validation.
更多查看译文
关键词
Structural Health Monitoring,Damage identification,Deep Learning,Varying environmental and operational conditions
AI 理解论文
溯源树
样例
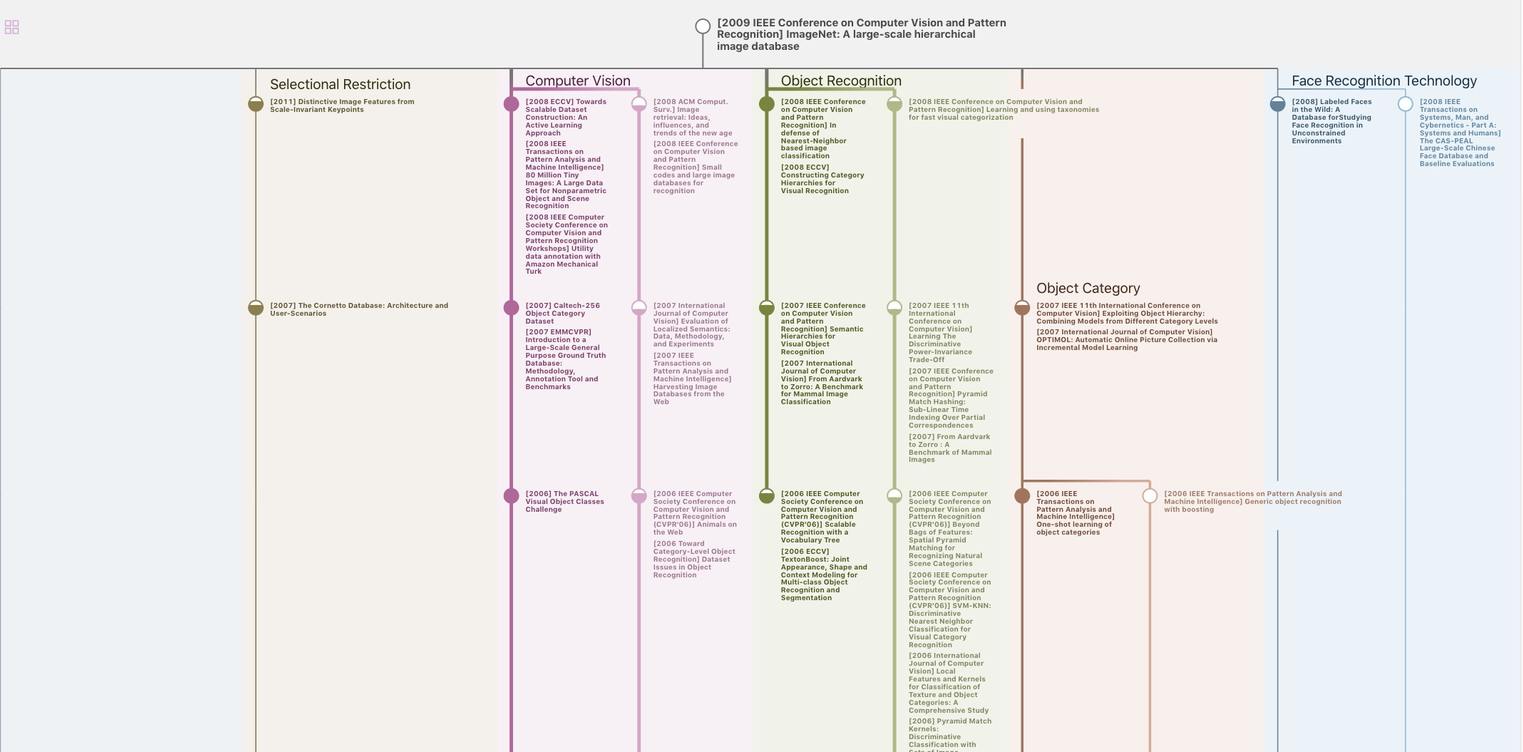
生成溯源树,研究论文发展脉络
Chat Paper
正在生成论文摘要