Predicting Graft Thrombosis in Patients with Peripheral Artery Disease Using a Support Vector Machine Learning Model
Journal of Vascular Surgery(2023)
摘要
The number of revascularizations performed for peripheral artery disease (PAD) has nearly doubled over the past decade. Subacute graft/stent thrombosis (≤6 months) occurs in up to 20% of revascularizations and is associated with amputation and subsequent death. Predicting patients that are at risk of graft/stent thrombosis is an important first step in tailoring thromboprophylaxis to potentially mitigate graft/stent loss. Objective coagulation assays such as thromboelastography with platelet mapping (TEG-PM) can provide a point of care assessment of a patient's coagulation status. The aim of this prospective study was to develop a computational model to predict graft/stent thrombosis following lower extremity revascularization in patients with PAD using machine learning techniques with viscoelastic and clinical data input. All patients undergoing lower extremity revascularization between December 2020 and December 2022 were prospectively enrolled. Clinical, anatomic, and operative details were collected. Whole blood TEG-PM samples were analyzed for up to 10 time points over 6 months. In machine learning, support vector machines are supervised learning models with associated learning algorithms that analyze data for classification and regression analysis. Missing values were first imputed using the average of 10 multiple-imputation simulations. Inferential analysis was then conducted across 31 variables comparing the thrombotic group to nonevents. Mathematical simulations were conducted to develop a support vector machine learning model to predict subacute thrombosis. The support vector machine learning model included data from 179 patients, totaling 5,549 input values (Table I). Forty-three patients (24.0%) experienced subacute graft/stent thrombosis. Support vector machines were trained on approximately 80% (n = 140 patients) to classify the endpoint of thrombosis. The remaining 20% (n = 39) of patient data was used to test the model. Observations were randomly assigned into the training and testing sets. All variables contained within Table I were included in our model, with the viscoelastic inputs of platelet aggregation/inhibition (P < .001) and diabetes (P = .032) having the most statistically significant contributions. The overall percent agreement was 96.1%, with a kappa of 0.89, indicating excellent agreement. All predicted thrombotic events were in fact events (100% specificity). Of the 43 thrombosis events, 36 were correctly identified (84% sensitivity) (Table II). Using prospectively collected, real-world clinical and viscoelastic data from patients with PAD, we developed a novel and highly sensitive and specific method of predicting subacute graft and stent thrombosis through machine learning. Such computational vectors may provide an approach to potentially mitigate limb loss via tailored postoperative thromboprophylaxis management based in objective data.Table IDescriptive characteristics of characteristics by thrombotic event status among n = 179 patientsThrombosisTotalNoYesNo.%No.%P-valueNo.%Current smoker3122.8716.3.3623821.2Former smoker8260.32865.1.57111061.5Ever smoker11383.13479.1.54914782.1Hypertension11886.83990.7.49415787.7Diabetes mellitus6346.32865.1.0329150.8Coronary artery disease6850.02148.8.8948949.7Myocardial infarction2619.11023.3.5553620.1HL9670.62967.4.69512569.8Chronic kidney disease4936.01125.6.2066033.5Prior intervention6245.62251.2.5238446.9Endovascular6044.12251.2.4198245.8Combined2619.1716.3.6763318.4Open5036.81432.6.6166435.8American Society of Anesthesiologists score10073.53274.4.90813273.7Plavix4936.01432.6.6786335.2Mono antiplatelet therapy7857.42660.5.71810458.1Dual antiplatelet therapy3626.51023.3.6744625.7Anticoagulants4633.81841.9.3386435.8Direct oral anticoagulants3122.81432.6.1984525.1Male9368.42967.4.90812268.2White11786.03479.1.27315184.4MeanSDMeanSDP-valueMeanSDPlatelet aggregation56.527.474.623.8<.00160.927.6Platelet inhibition43.227.525.823.7<.00139.027.6R time7.43.08.64.2.06062.09.9K time1.91.31.71.3.45062.09.9Angle68.910.167.512.8.45368.510.8CRT MA62.09.963.88.2.30562.59.5Body mass index27.36.127.34.6.94627.35.7International normalized ratio1.40.71.41.3.7731.40.9Prothrombin time16.56.116.910.3.84316.67.4Partial prothrombin time49.528.054.525.8.48951.027.3SD, Standard deviation.To determine P-values, Pearson χ2 tests were used for dichotomous variables, and t tests were used for continuous variables. Open table in a new tab Table IIPredictive agreement between a support vector machine model and observed valuesPredicted thrombosisObserved thrombosisTotalNoYesNo136 (100.0)7 (16.3)143 (79.9)Yes0 (0.0)36 (83.7)36 (20.1)Total136 (100.0)43 (100.0)179 (100.0)Data are presented as number (%). Open table in a new tab
更多查看译文
关键词
support vector machine,graft thrombosis,peripheral artery disease,machine learning
AI 理解论文
溯源树
样例
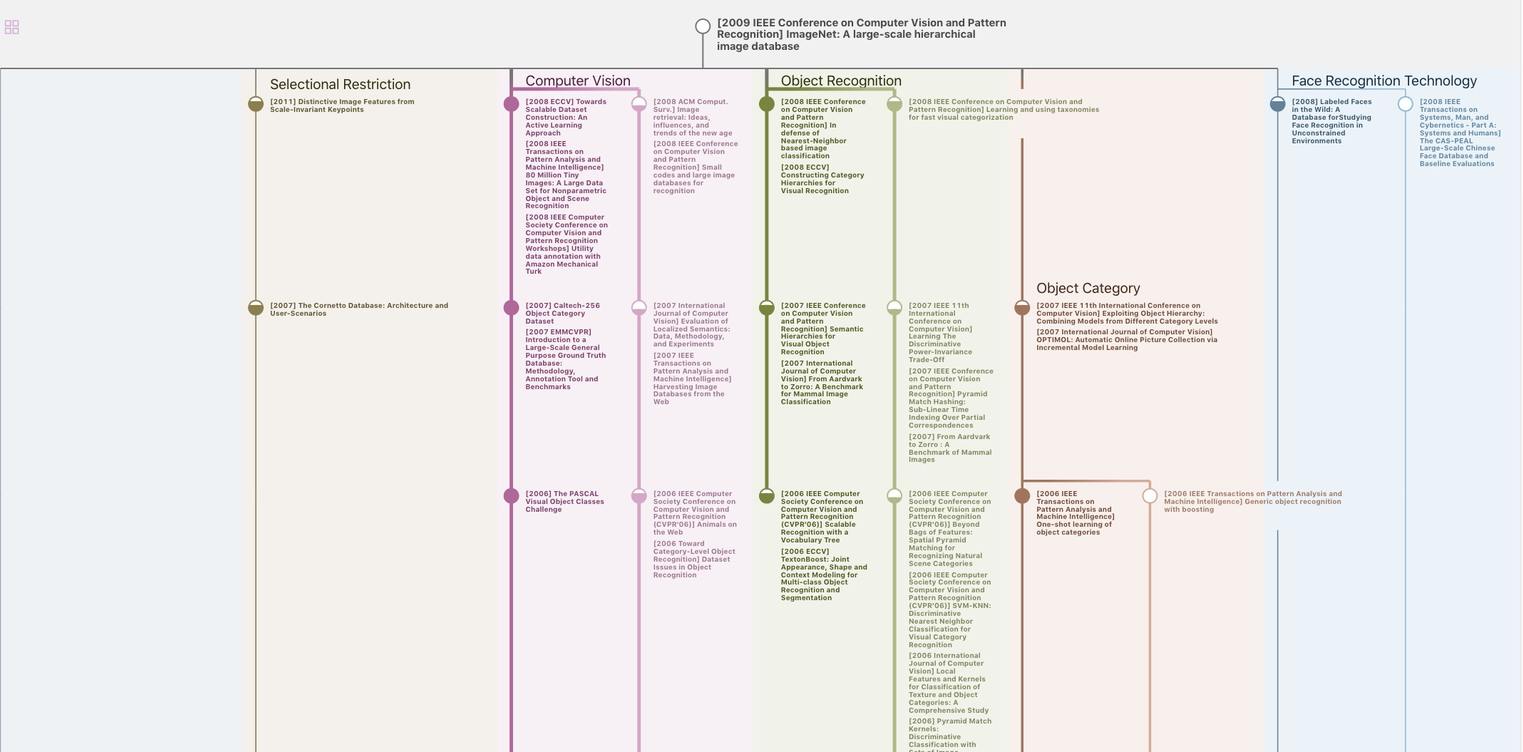
生成溯源树,研究论文发展脉络
Chat Paper
正在生成论文摘要