Study on strategies for reducing training samples for accurate estimation of wind-induced structural response of LSTM networks
Journal of Wind Engineering and Industrial Aerodynamics(2023)
摘要
Long short-term memory (LSTM) neural network is an efficient method to analyze the nonlinear dynamical response of structures. However, once it is applied to a long-duration response prediction task, the demand on the computational resources will become very severe. In order to address this limitation, various strategies based on sample truncation and down-sampling were proposed in this study to reduce the sample size for predicting wind-induced structural response, i.e., to intercept representative short-duration samples from long-duration samples or down sample the long-duration sample to train the LSTM neural network. These novel strategies can achieve the goal of reducing computational burdens without loss of accuracy compared to the long-duration training samples. Based on a single-degree-of-freedom (SDOF) system, the advantages in terms of the time efficiency of the proposed methods on the training phase of LSTM neural networks have been studied, and the accuracy of the predicted wind-induced structural response was investigated. It showed that the Duhamel integral-based approach (DI-BA) of sample truncation presented the best comprehensive performance in time and accuracy. Furthermore, the DI-BA was applied to a four-degree-of-freedom (4DOF) system with different structural parameters to demonstrate its good performance in more general applications involving multiple degrees of freedom.
更多查看译文
关键词
Deep learning,Long short-term memory neural network,Surrogate model,Wind-induced structural response,Dynamic analysis
AI 理解论文
溯源树
样例
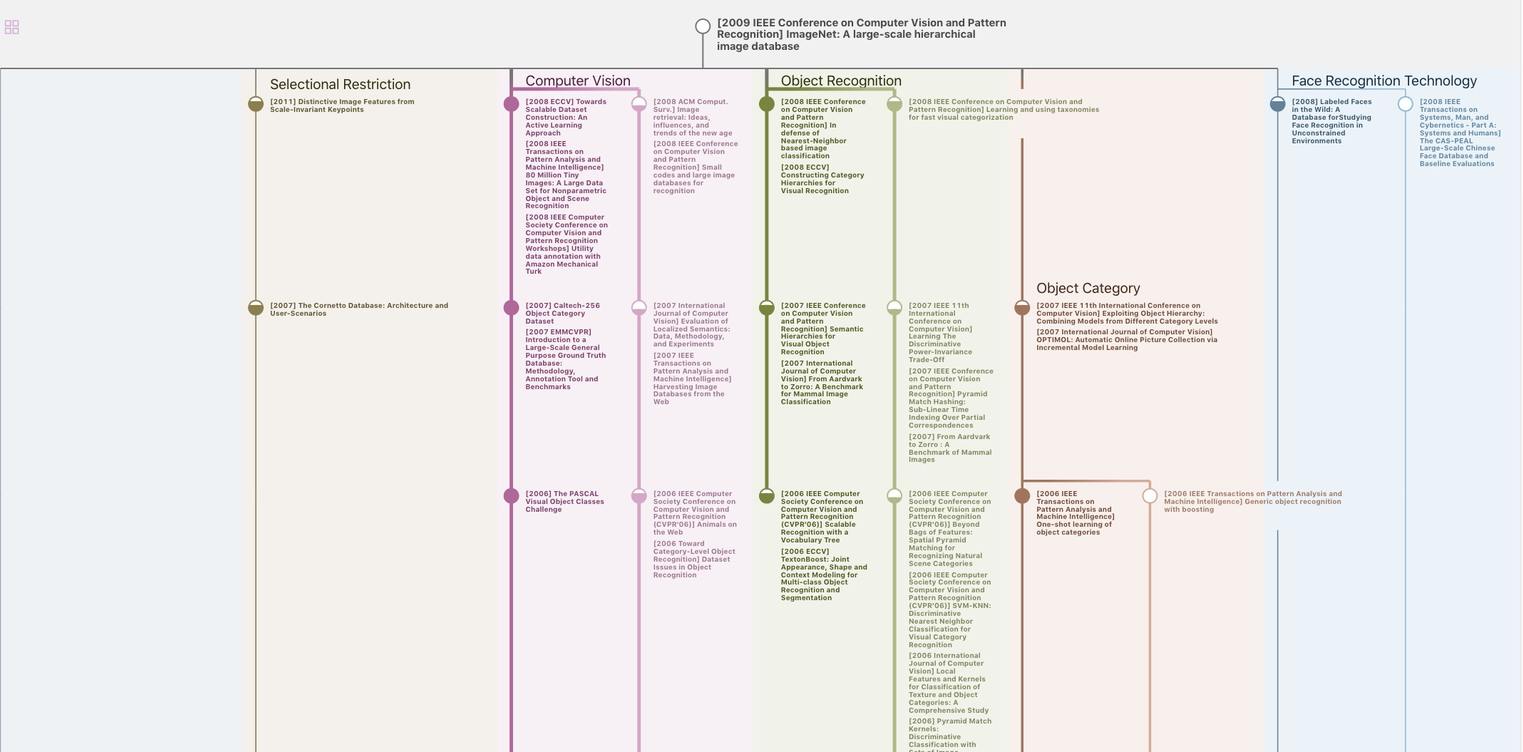
生成溯源树,研究论文发展脉络
Chat Paper
正在生成论文摘要