A multivariate monitoring method based on kernel principal component analysis and dual control chart
Journal of Process Control(2023)
摘要
The kernel principal component analysis (KPCA) method has been widely applied in process monitoring. However, the KPCA method still has two challenging problems that need to be solved: the principal component selection problem and the problem of determining Gaussian kernel width without fault data. The principal component selection plays an important role in the performance of the KPCA method, which is determined by the cumulative contribution rate, cross-validation, average eigenvalue, etc. However, these selection methods ignore the principal components corresponding to small eigenvalues, which are still very important for fault detection. Therefore, this paper proposes a multivariate monitoring method based on the KPCA and Dual Control Chart (KPCA-DCC), which adopts a scheme of monitoring all principal components and solves the selection problem. The Gaussian kernel width is usually determined by training an appropriate coefficient to times the variable number, which may not be possible to train the coefficient in the absence of fault data while the industrial process works at the initial stage of its operation. Furthermore, different systems may have the same number of variables, and their Gaussian kernel widths should vary because of different variables’ features. Therefore, this paper proposes an empirical equation for estimating Gaussian kernel width according to data characteristics, which makes the Gaussian kernel width can be estimated without fault data. In addition, this paper also gives the detection theory of the DCC method from the probability perspective, which is not presented in the previous work. The Tennessee Eastman (TE) process and blast furnace ironmaking process are adopted to verify the effectiveness of the proposed methods.
更多查看译文
关键词
Kernel principal component analysis, Dual control chart (DCC), KPCA-DCC, Kernel width, (KPCA)
AI 理解论文
溯源树
样例
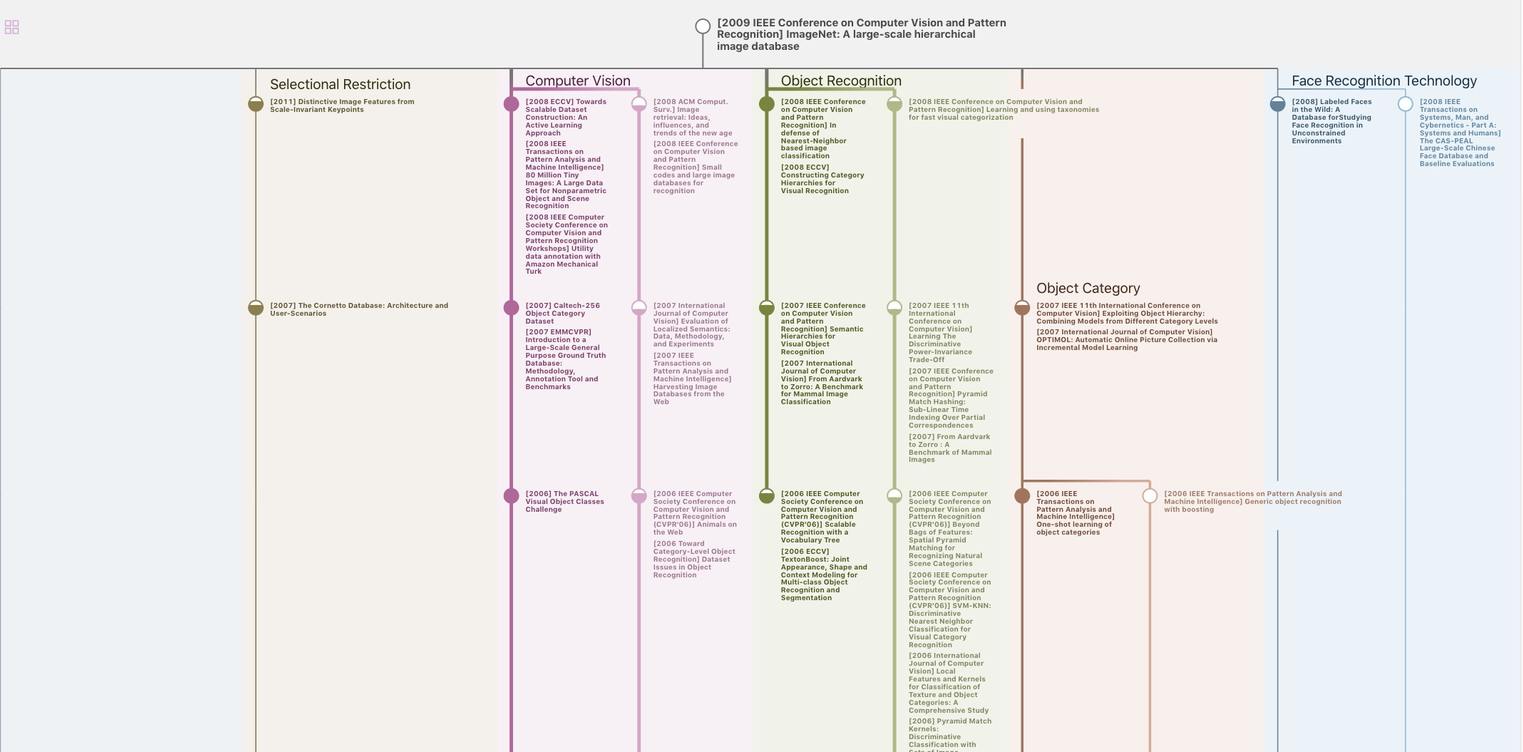
生成溯源树,研究论文发展脉络
Chat Paper
正在生成论文摘要