Low-level liquid cloud properties during ORACLES retrieved using airborne polarimetric measurements and a neural network algorithm
crossref(2019)
摘要
Abstract. In this study we developed a neural network (NN) that can be used to relate a large dataset of multi-angular and multi-spectral polarimetric remote sensing observations to retrievals of cloud microphysical properties. This effort builds upon our previous work, which explored the sensitivity of neural network input, architecture, and other design requirements for this type of remote sensing problem. In particular this work introduces a framework for appropriately weighting total and polarized reflectances, which have vastly different magnitudes and measurement uncertainties. The NN is trained using an artificial training set and applied to Research Scanning Polarimeter (RSP) data obtained during the ORACLES field campaign (Observations of Aerosols above Clouds and their Interactions). The polarimetric RSP observations are unique in that they observe the same cloud from a very large number of angles within a variety of spectral bands resulting in a large dataset that can be explored rapidly with a NN approach. The usefulness applying a NN to a dataset such as this one stems from the possibility of rapidly obtaining a retrieval that could be subsequently applied as a first-guess for slower but more rigorous physical-based retrieval algorithms. This approach could be particularly advantageous for more complicated atmospheric retrievals – such as when an aerosol layer lies above clouds like in ORACLES. For the ORACLES 2016 dataset comparisons of the NN and standard parametric polarimetric (PP) cloud retrieval give reasonable results for droplet effective radius (re : R = 0.756, RMSE = 1.74 μm) and cloud optical thickness (τ : R = 0.950, RMSE = 1.82). This level of statistical agreement is shown to be similar to comparisons between the two most well-established cloud retrievals, namely the the polarimetric cloud retrieval and the bispectral total reflectance cloud retrieval. The NN retrievals from the ORACLES 2017 dataset result in retrievals of re (R = 0.54, RMSE = 4.77 μm) and τ (R = 0.785, RMSE = 5.61) that behave much more poorly. In particular we found that our NN retrieval approach does not perform well for thin (τ <3), inhomogeneous, or broken clouds. We also found that correction for above-cloud atmospheric absorption improved the NN retrievals moderately – but retrievals without this correction still behaved similarly to existing cloud retrievals with a slight systematic offset.
更多查看译文
AI 理解论文
溯源树
样例
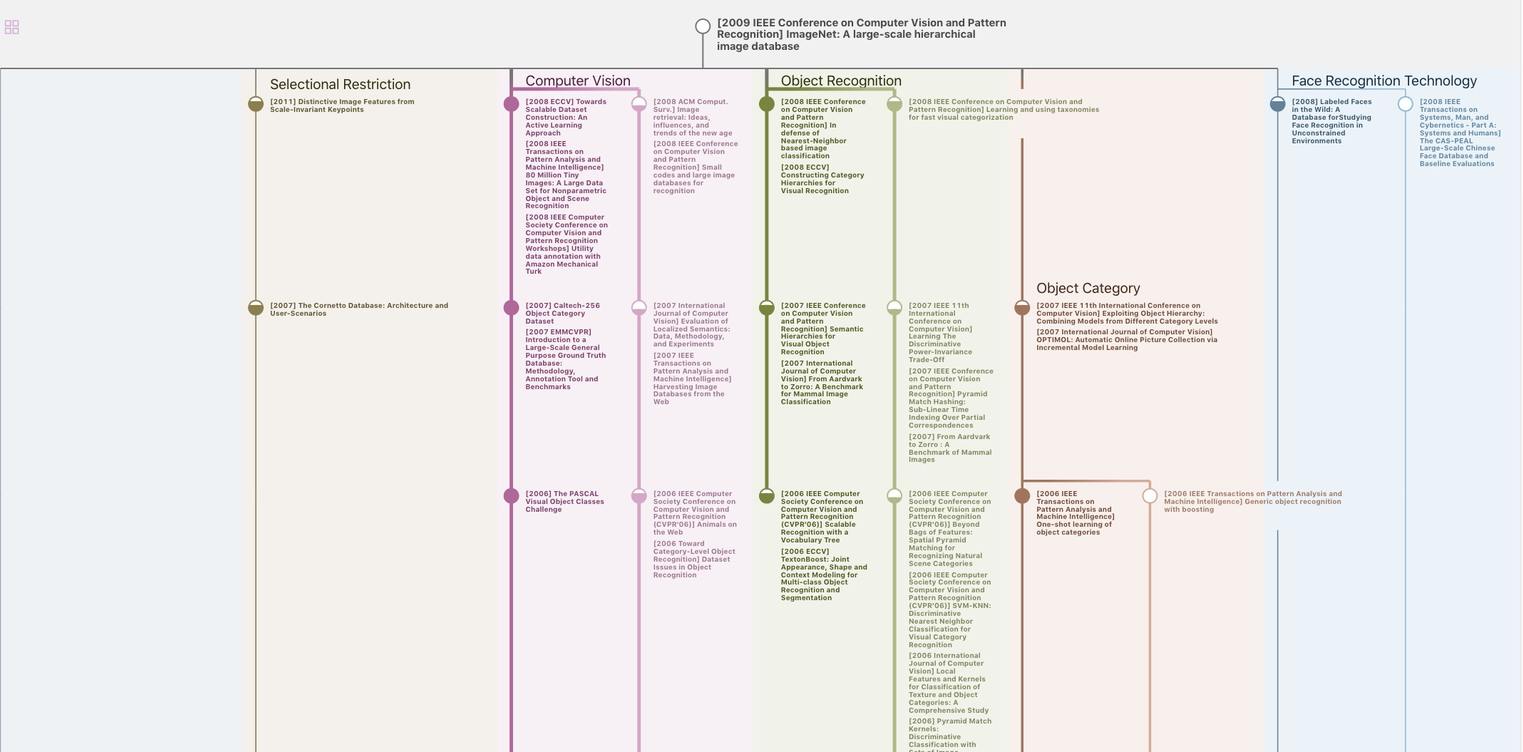
生成溯源树,研究论文发展脉络
Chat Paper
正在生成论文摘要