Joint UAV Trajectory Planning, DAG Task Scheduling, and Service Function Deployment Based on DRL in UAV-Empowered Edge Computing
IEEE Internet of Things Journal(2023)
摘要
Unmanned aerial vehicle (UAV)-empowered edge computing has been widely investigated in obstacle-free scenarios, where a moving UAV is in charge of handling offloaded singleton tasks from mobile devices on the ground. However, little attention has been paid to the scenario, in which the UAV serves a complex area with multiple obstacles and dependent tasks. A dependent task can be formulated as a directed acyclic graph (DAG) that contains a number of subtasks; and each subtask can be executed by a corresponding service function (SF) deployed on the UAV. In this backdrop, the joint UAV trajectory planning, DAG task scheduling, and SF deployment is formulated as an optimization problem in this article. Afterwards, a deep reinforcement learning (DRL)-based algorithm is presented to tackle the NP-hard problem. The state space, action space, and the reward function of the agent, i.e., the UAV, are defined, respectively, under the DRL framework. To evaluate the effectiveness of the proposal, a series of experiments is conducted with different parameter settings. Results show that the DRL-based algorithm performs much better than three heuristic algorithms in success rate of trajectory planning, the number of executed tasks, and the average task response latency.
更多查看译文
关键词
Deep reinforcement learning (DRL),edge computing,service function (SF),unmanned aerial vehicle (UAV)
AI 理解论文
溯源树
样例
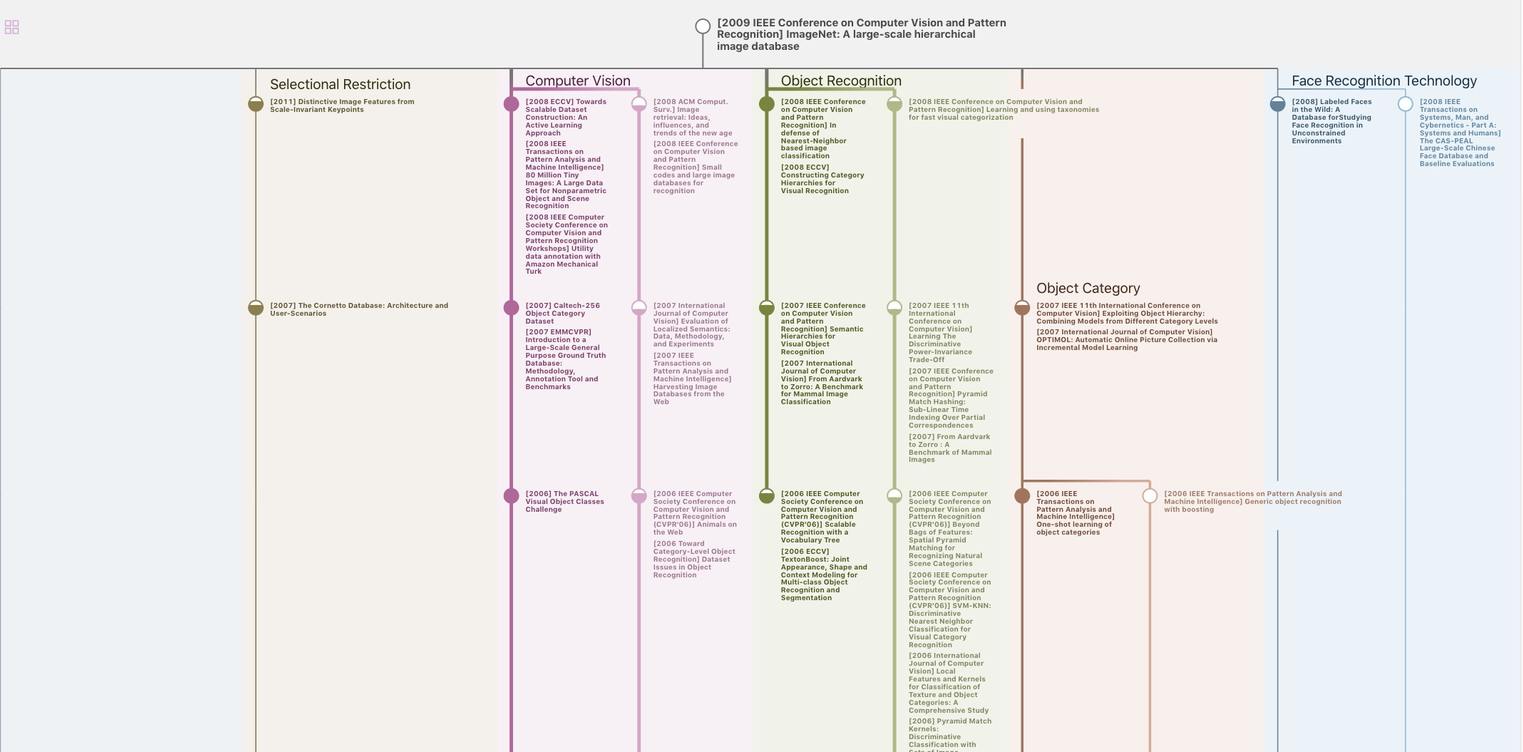
生成溯源树,研究论文发展脉络
Chat Paper
正在生成论文摘要