Perspectives on Health Disparities and Inequities in Radiation Oncology and Opportunities for Medical Physicists: From Awareness to Action
International Journal of Radiation Oncology*Biology*Physics(2023)
摘要
Health disparities in outcomes and inequities in access to radiation therapy (RT) across races in the United States have been well documented, particularly in cancer. 1 Wheeler SB Reeder-Hayes KE Carey LA. Disparities in breast cancer treatment and outcomes: Biological, social, and health system determinants and opportunities for research. Oncologist. 2013; 18: 986-993 Crossref PubMed Scopus (173) Google Scholar The sources of these disparities are multifactorial and include complex social, political, and historical interpersonal, structural, and systemic factors. 2 Purnell TS Calhoun EA Golden SH et al. Achieving health equity: Closing the gaps in health care disparities, interventions, and research. Health Affairs. 2016; 35: 1410-1415 Crossref PubMed Scopus (172) Google Scholar Some of these patient-level factors may be more obviously within the immediate day-to-day sphere of influence of medical physicists, while others, such as socioeconomic status and other social determinants of health, may require larger scale strategies to effectively impact them over time. Nevertheless, the first step to effecting change begins with understanding the existing factors, particularly those within our sphere of influence. The next step is to appreciate the ways in which all members who contribute to the health care system may have affected such disparities. As an example, medical physicists who do not typically provide direct clinical care may believe they do not influence health disparities. However, recent work has shown that artificial intelligence (AI) models may perpetuate bias that is inherent to real-world data used for training. 3 Dankwa-Mullan I Weeraratne D. Artificial intelligence and machine learning technologies in cancer care: Addressing disparities, bias, and data diversity. Cancer Discov. 2022; 12: 1423-1427 Crossref PubMed Scopus (5) Google Scholar This is also true of predictive models or treatment regimens developed from clinical trials, which typically do not include representative proportions of ethnic and racial populations. 4 Aldrighetti CM Niemierko A Van Allen E et al. Racial and ethnic disparities among participants in precision oncology clinical studies. JAMA Netw Open. 2021; 4:e2133205 Crossref PubMed Scopus (41) Google Scholar This bias in the real-world data and skewed study populations is, in fact, propagated into the final algorithms and clinical recommendations. An example outside of health care is Amazon's AI algorithm, which was intended to help select talented candidates for highly paid positions but was ultimately biased to select male candidates. 5 Goodman R. Why Amazon's automated hiring tool discriminated against women. Available at:https://www.aclu.org/news/womens-rights/why-amazons-automated-hiring-tool-discriminated-against. Accessed October 1, 2022. Google Scholar In the health care setting, Obermeyer et al 6 Obermeyer Z Powers B Vogeli C et al. Dissecting racial bias in an algorithm used to manage the health of populations. Science. 2019; 366: 447-453 Crossref PubMed Scopus (1442) Google Scholar reported on the use of a widely available algorithm intended to aid clinicians in identifying patients with complex health needs who warrant additional interventions and resources. They discovered that the algorithm used health costs as a proxy, which was biased against Black patients compared with White patients because health care expenditure for White patients is higher than Black patients with the same comorbidities. Obermeyer et al state that such bias is “pernicious because it can arise from reasonable choices.” 6 Obermeyer Z Powers B Vogeli C et al. Dissecting racial bias in an algorithm used to manage the health of populations. Science. 2019; 366: 447-453 Crossref PubMed Scopus (1442) Google Scholar Physicists who are developing algorithms for AI should be aware of this unintended pitfall and use tools to assess bias, generalizability, and other measures of structural racism in their algorithms. 7 Bellamy RKE Dey K Hind M et al. AI fairness 360: An extensible toolkit for detecting and mitigating algorithmic bias. IBM J Res & Dev. 2019; 63 (1-4:15): 4 Crossref Scopus (183) Google Scholar The Medical Imaging Data Resource Center is a repository of COVID-19 images that also serves to provide sequestered data for use by AI researchers. 8 Baughan N Whitney H Drukker K et al. Sequestration of imaging studies in MIDRC: A multi-institutional data commons. in: Mello-Thoms CR Taylor-Phillips S Medical Imaging 2022: Image Perception, Observer Performance, and Technology Assessment. SPIE, San Diego, CA2022: 18 Crossref Scopus (2) Google Scholar Due to the racial disparities encountered during the COVID-19 pandemic, the scientists developing this resource were forward-thinking and have incorporated race as a means for researchers to assess bias in AI algorithms using these data.
更多查看译文
关键词
radiation oncology,health disparities,medical physicists
AI 理解论文
溯源树
样例
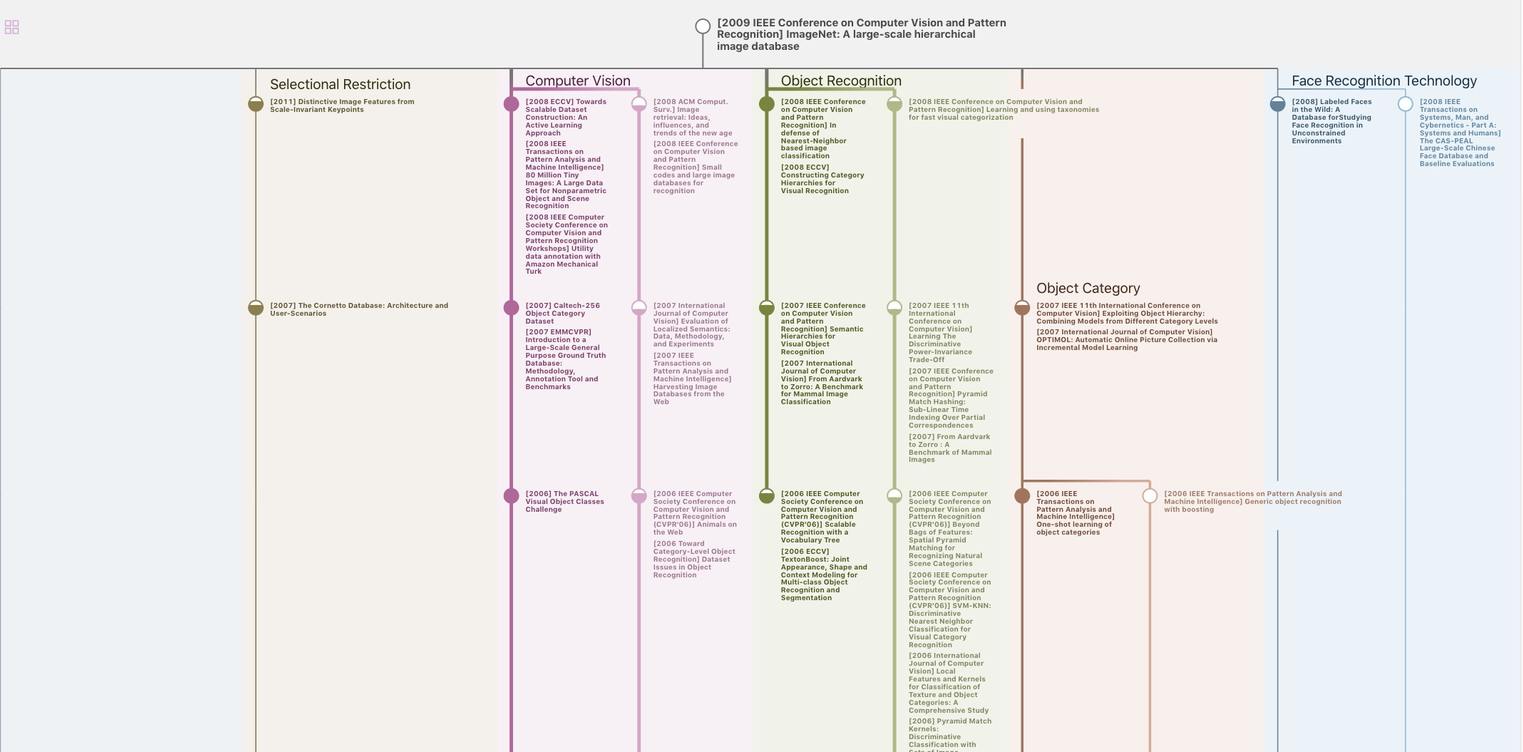
生成溯源树,研究论文发展脉络
Chat Paper
正在生成论文摘要