Offline economic dispatch for multi-area power system via hierarchical reinforcement learning
International Journal of Electrical Power & Energy Systems(2023)
摘要
In this paper, a novel hierarchical reinforcement learning (HRL) architecture is put forward to resolve the multi-area economic dispatch (MAED) problem in a fully off-line optimization manner, while the focus of MAED is to minimize the total generation cost of the entire multi-area power system by determining output power within area and the power transfer among areas. The original MAED problem is transformed into an augmented sub-MAED problem by exploring the relationship between power balance constraint and tie line capacity constraint in each area. This architecture first calculates the scheduled area load in the top layer through the primal–dual consensus-based distributed Q-learning, and then calculates load-power table assembled by the optimal output power within single area according to the multi-stage decision algorithm in the bottom layer. Acting as an underlying framework, the assigned load-power table is implemented with the combination of off-line calculation and on-line inquiry. It is capable of parallel and asynchronous optimization in each area while satisfying various constraints. Furthermore, a power-triggered algorithm is proposed to satisfy the power balance among areas, and its convergence is theoretically analyzed, which illustrates that the area power error will converge to zero in the limited iteration. Finally, the proposed approach is validated in three different cases: 20-unit, 40-unit, and 120-unit. The comparative results confirmed the superiority of the proposed HRL architecture with high real-time performance and less computation burden. Specifically, the HRL architecture reduces the fuel cost in the range of 0.01656%–0.19173% and accelerates the speed more than 10 times than others.
更多查看译文
关键词
offline economic dispatch,economic dispatch,multi-area
AI 理解论文
溯源树
样例
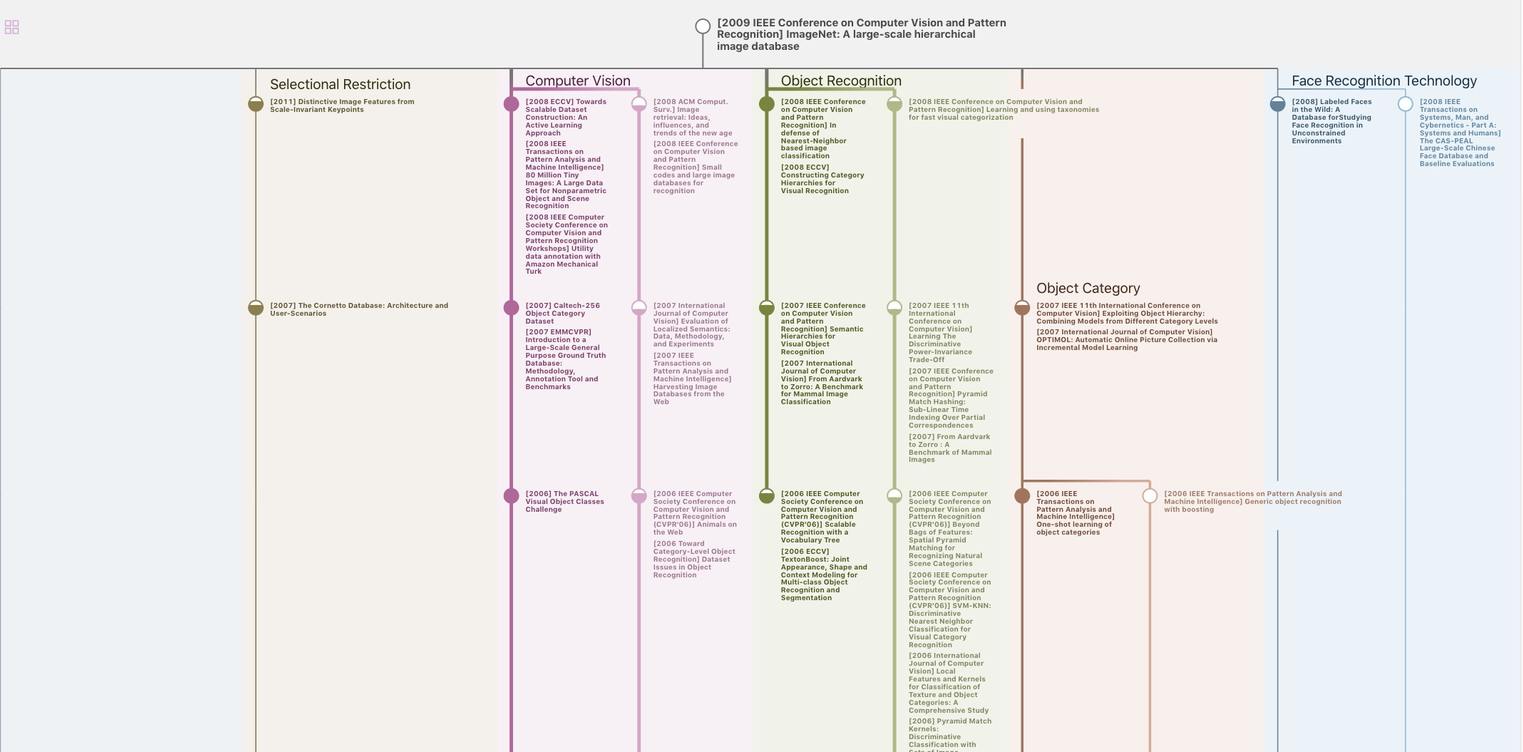
生成溯源树,研究论文发展脉络
Chat Paper
正在生成论文摘要