Rooted Mahalanobis distance based Gustafson-Kessel fuzzy C-means
Information Sciences(2023)
摘要
Fuzzy c-means (FCM) is a classic unsupervised clustering algorithm in machine learning fields. Euclidean distance is a frequently used distance metric in FCM, but it is only suitable for data with spherical clusters. Therefore, Mahalanobis distance was introduced into Gustafson-Kessel Fuzzy C-Means (GK-FCM) to help improve the performance on data with ellipsoidal clusters. However, GK-FCM and existing Mahalanobis distance based algorithms only focus on squared Mahalanobis distance, because squared Mahalanobis distance based problems are usually convex and easily solvable. But squared Mahalanobis distance is not a perfect metric, because it tends to exaggerate the influence of outliers and lead to unsatisfying results. In this paper, we propose a rooted Mahalanobis distance based GK-FCM model, which has better clustering performance and superior robustness than traditional GK-FCM. Moreover, owing to the introduction of rooted Mahalanobis distance, the optimization of the proposed model becomes non-trivial and it is not realistic to obtain a closed-form solution as that of traditional GK-FCM. In this paper, by making reference to the re-weighted method, we develop a novel iterative converging algorithm to optimize the proposed model. Finally, extensive experiments are conducted on both synthetic and real-world data sets to manifest the superiority of the proposed model.
更多查看译文
关键词
Mahalanobis distance,Gustafson Kessel,Unsupervised,Fuzzy C-means,Clustering
AI 理解论文
溯源树
样例
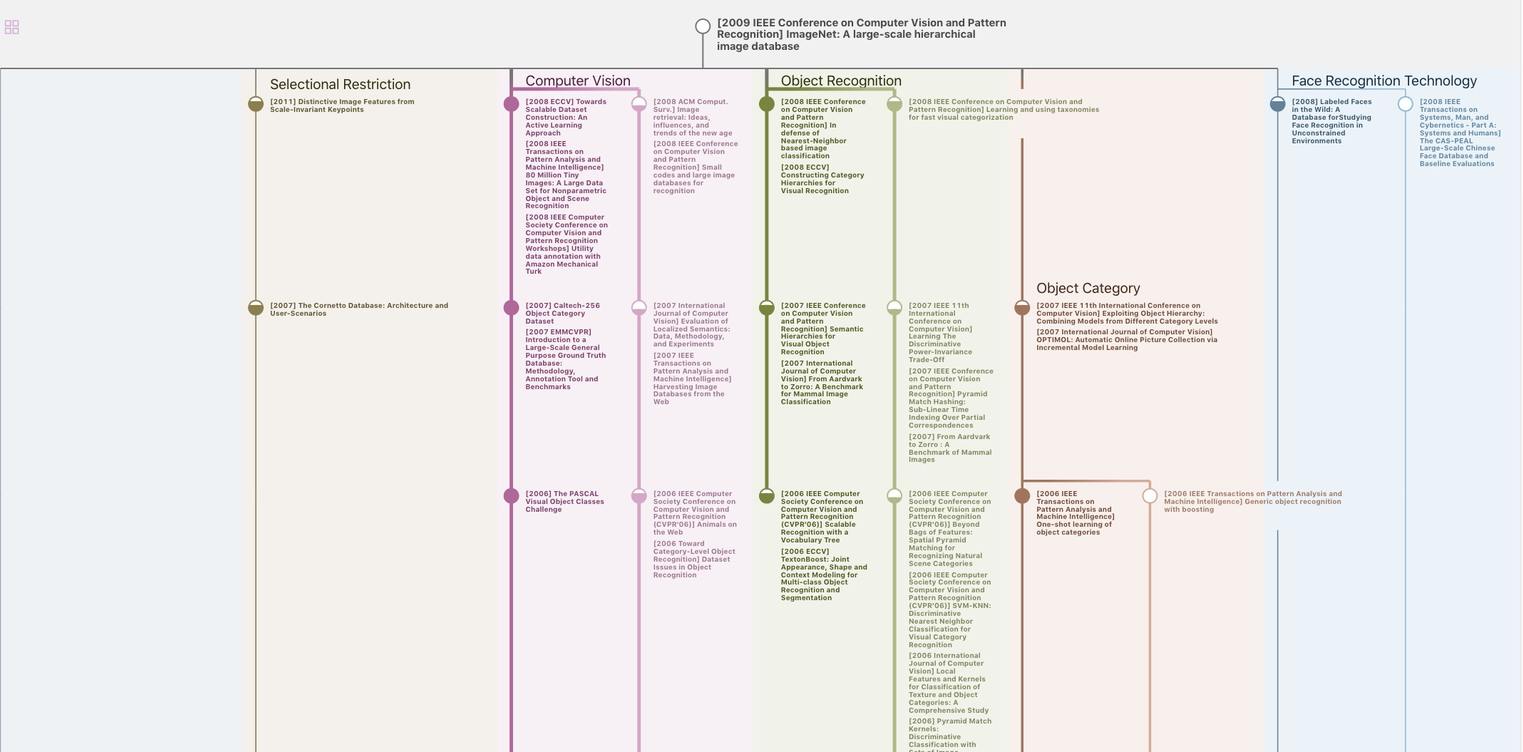
生成溯源树,研究论文发展脉络
Chat Paper
正在生成论文摘要