Leveraging hierarchical language models for aspect-based sentiment analysis on financial data
Information Processing & Management(2023)
摘要
Every day millions of news articles and (micro)blogs that contain financial information are posted online. These documents often include insightful financial aspects with associated sentiments. In this paper, we predict financial aspect classes and their corresponding polarities (sentiment) within sentences. We use data from the Financial Question & Answering (FiQA) challenge, more precisely the aspect-based financial sentiment analysis task. We incorporate the hierarchical structure of the data by using the parent aspect class predictions to improve the child aspect class prediction (two-step model). Furthermore, we incorporate model output from the child aspect class prediction when predicting the polarity. We improve the F1 score by 7.6% using the two-step model for aspect classification over direct aspect classification in the test set. Furthermore, we improve the state-of-the-art test F1 score of the original aspect classification challenge from 0.46 to 0.70. The model that incorporates output from the child aspect classification performs up to par in polarity classification with our plain RoBERTa model. In addition, our plain RoBERTa model outperforms all the state-of-the-art models, lowering the MSE score by at least 28% and 33% for the cross-validation set and the test set, respectively.
更多查看译文
关键词
Text data,Financial aspect classes,Polarity,Hierarchical structure of data
AI 理解论文
溯源树
样例
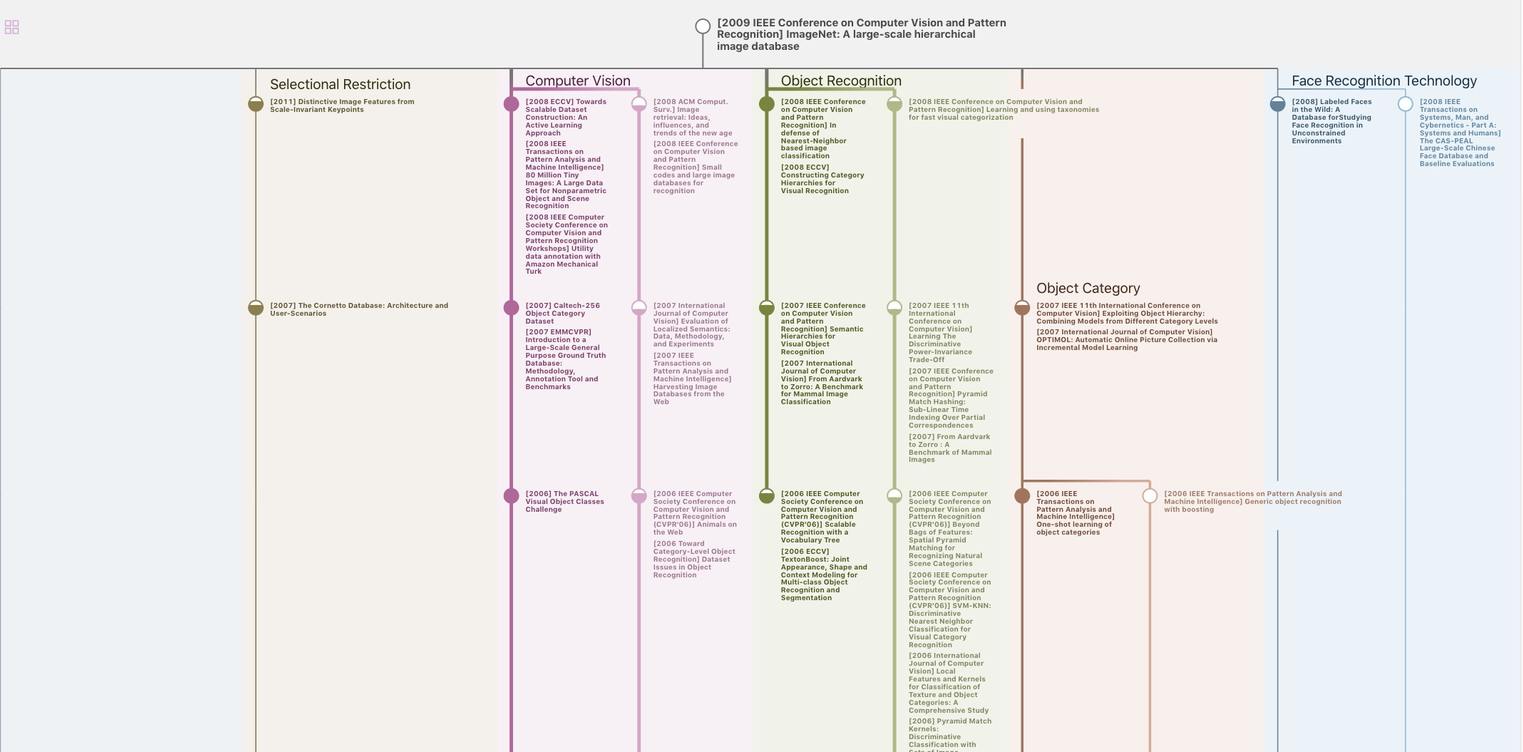
生成溯源树,研究论文发展脉络
Chat Paper
正在生成论文摘要