MCANet: Hierarchical cross-fusion lightweight transformer based on multi-ConvHead attention for object detection
Image and Vision Computing(2023)
摘要
The visual Transformer model based on self-attention has achieved better performance than convolutional neural networks in object detection tasks. However, existing visual Transformer models are typically heavy-weight to extract global features. In contrast, CNNs can extract features with fewer parameters and computational costs. To combine the advantages of convolutional processing at the local level with the advantages of the Transformer's global interaction, this paper proposes MCANet, a Hierarchical Cross-Fusion Lightweight Transformer Based on Multi-ConvHead Attention for Object Detection. To bi-directionally fuse local and global features, MCANet adds two improved transformers (MCA-Former) for global interaction and two novel feature fusion modules MCA-CSP. MCA-Former uses a novel self-attention computation method named Multi-ConvHead Attention(MCA) based on multi-scale depth-separable convolution, which reduces the computational cost by 2/3. Meanwhile, the number of model parameters is reduced to 9.49 M by using channel segmentation and multi-layer cross- fusion strategies. On the Pascal VOC and COCO datasets, the proposed model outperforms YOLOv4-Tiny in terms of AP by 2.43% and 1.8%, respectively. Additionally, MCANet is also superior to many latest lightweight object detection models. Results of various ablation experiments also verify the effectiveness of the proposed method.
更多查看译文
关键词
object detection,transformer,attention,cross-fusion,multi-convhead
AI 理解论文
溯源树
样例
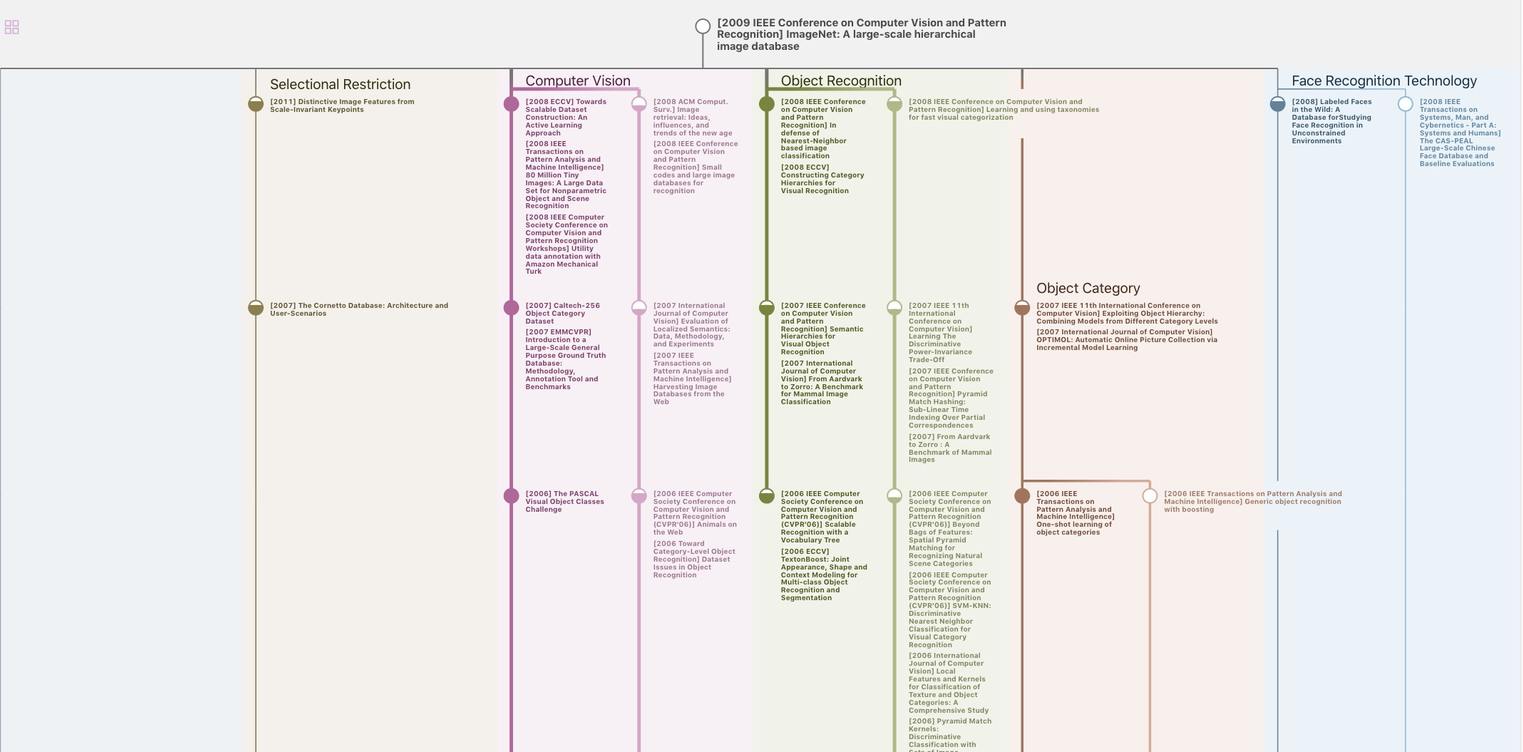
生成溯源树,研究论文发展脉络
Chat Paper
正在生成论文摘要