Interpreting the effects of shale rock properties on seismic anisotropy by statistical and machine learning methods
Geoenergy Science and Engineering(2023)
摘要
The elastic anisotropy of unconventional shale reservoirs plays a crucial role in their economic hydrocarbon production. The strong anisotropy of organic-rich shale rocks, characterized by clay platelets, elongated pores, and organic material, presents challenges for traditional reservoir characterization methods, which are designed for isotropic or weakly anisotropic conventional sandstone reservoirs. To effectively describe the geomechanical behaviors of organic-rich shales and estimate changes in stress caused by hydraulic fracturing, it is necessary to develop practical methods for predicting anisotropy parameters from conventional rock property logs. This study proposes a statistical approach for predicting independent elastic stiffness coefficients for vertically transverse isotropic (VTI) shale from three shale rock properties: clay volume fraction, kerogen volume fraction, and total porosity. The results of this study show that the stiffness coefficients, C11 and C33, are related to P-wave anisotropy and porosity has a greater impact on P-wave anisotropy than clay and kerogen volume fractions. In contrast, changes in clay volume have a more sensitive effect on Co and C66, providing S-wave anisotropy in-formation that can help detect lithology changes. Furthermore, kerogen variation has a greater influence on both C12 and C13 than the effects of porosity and clay variations. From these relationships, we develop exponential nonlinear regression (ENLR) models for predicting independent elastic stiffness coefficients from three shale rock properties. These models are validated with the Midland Basin log data, and we improve the accuracy of the C13 estimation model using the deep feedforward neural network (DFNN) method. The results show that the sta-tistical approach can help understand the effect of shale rock components on seismic anisotropy variations and better predict the variations in lithology, fluid, and organic contents in shales from anisotropic seismic data. Furthermore, the methodology can provide robust estimates of the stress changes from hydraulic fracturing, supporting the efficient and economically viable production of shale hydrocarbons.
更多查看译文
关键词
Anisotropy,Elastic stiffness,Machine learning,Shapley value,Shale,Nonlinear regression
AI 理解论文
溯源树
样例
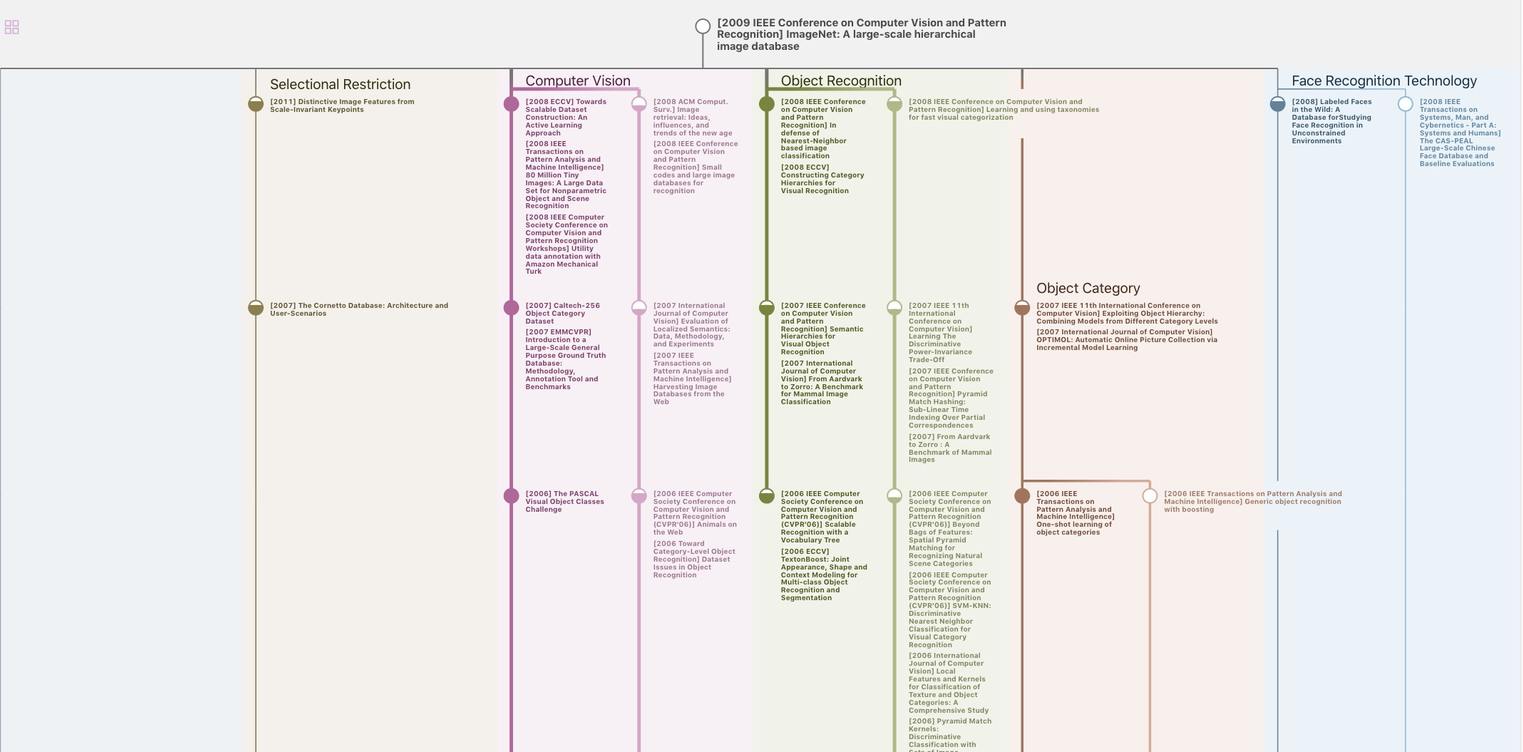
生成溯源树,研究论文发展脉络
Chat Paper
正在生成论文摘要