Embedded real-time objects’ hardness classification for robotic grippers
Future Generation Computer Systems(2023)
摘要
Robotic grippers can be equipped with tactile sensing systems to extract information from a manipulated object. The real-time classification of the physical properties of a grasped object on resource-constrained devices requires efficient and effective pre-processing techniques and machine-learning (ML) algorithms. In this paper, we propose a tactile sensing system mounted on the Baxter robot for the hardness classification of objects. In particular, we pre-processed the raw data with low computational cost techniques, and we designed three ML algorithms to provide real-time, energy-efficient, and low-memory impact classification on a resource-constrained microcontroller. Results show that convolutional neural networks (CNNs) achieve the best accuracy (>98%), while the support vector machine (SVM) presents the lowest memory occupation (1576 bytes), inference time (<0.077ms), and energy consumption (<5.74μJ).
更多查看译文
关键词
Resource-constrained devices, Machine learning, Tactile sensing, Robotic grippers, Hardness classification
AI 理解论文
溯源树
样例
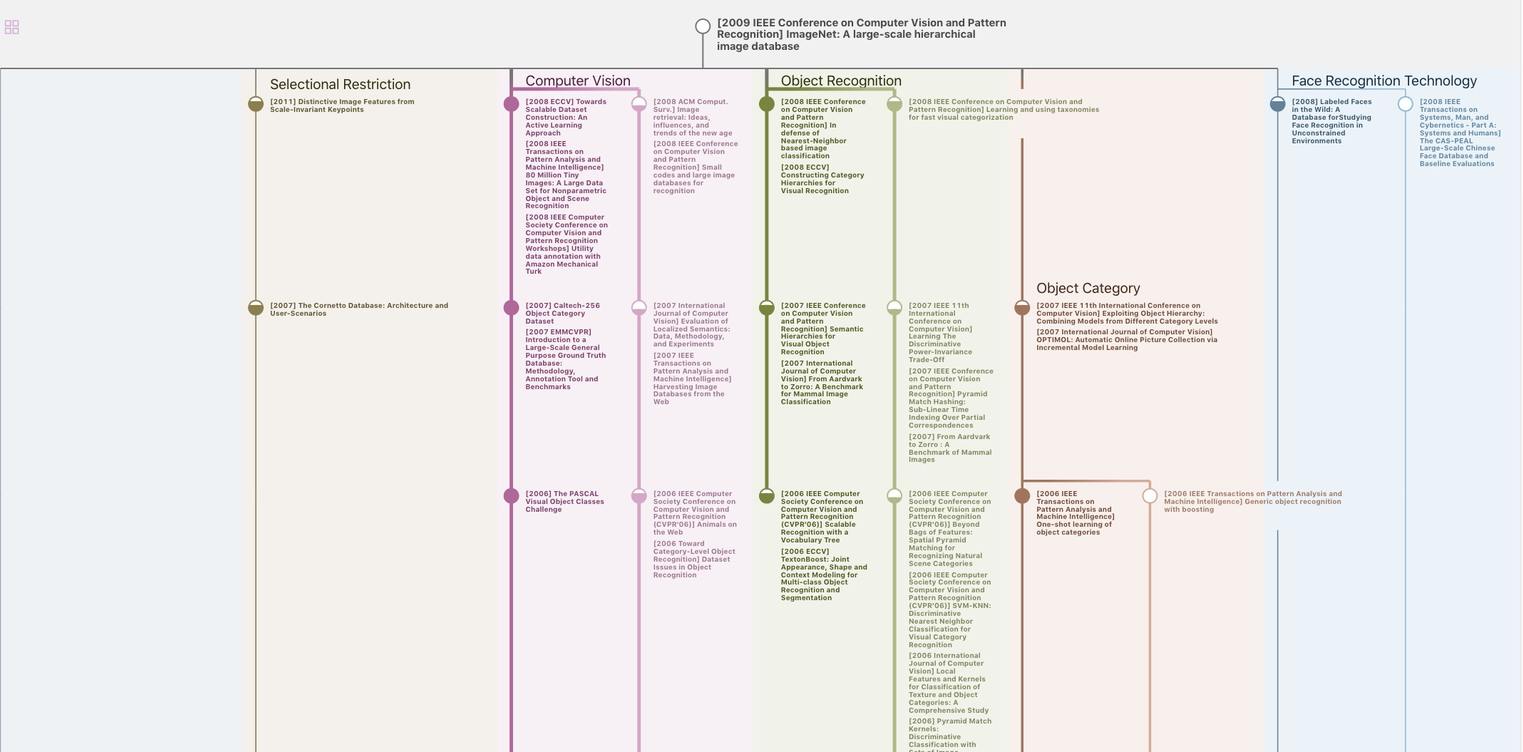
生成溯源树,研究论文发展脉络
Chat Paper
正在生成论文摘要