Multi-view imputation and cross-attention network based on incomplete longitudinal and multimodal data for conversion prediction of mild cognitive impairment
Expert Systems with Applications(2023)
摘要
Predicting whether subjects with mild cognitive impairment (MCI) can convert to Alzheimer's disease is significant for personalized treatment development and disease progression delay. Longitudinal and multimodal data have been recognized for their ability to capture longitudinal variations and provide complementary information for MCI conversion prediction. However, incomplete or missing data pose a persistent challenge in effectively utilizing such valuable information. Additionally, early-stage conversion prediction, particularly at baseline visit (BL), is crucial in clinical practice. Therefore, longitudinal data must only be incorporated during training to capture disease progression information. To address these challenges, we propose a multi-view imputation and cross-attention network (MCNet) to integrate data imputation and MCI conversion prediction in a unified framework. First, a multi-view imputation method combined with adversarial learning is presented to handle various missing data scenarios and reduce imputation errors. Second, two cross-attention blocks are introduced to exploit the potential associations in longitudinal and multimodal data. Finally, a multi-task learning model is established for data imputation, longitudinal classification, and conversion prediction. By appropriately training the model, disease progression information learned from longitudinal data improves the MCI conversion prediction that only uses BL data. To verify its effectiveness and flexibility in such MCI conversion prediction, we test MCNet on independent testing sets and single-modal BL data. Results show that MCNet outperforms competitive methods with an area under the receiver operating characteristic curve value of 86.0%. Furthermore, the interpretability of MCNet is demonstrated, indicating its potential as a valuable tool for incomplete data analysis in MCI conversion prediction.
更多查看译文
关键词
Adversarial learning,Cross-attention,Conversion prediction,Longitudinal and multimodal data,Mild cognitive impairment,Multi-view imputation
AI 理解论文
溯源树
样例
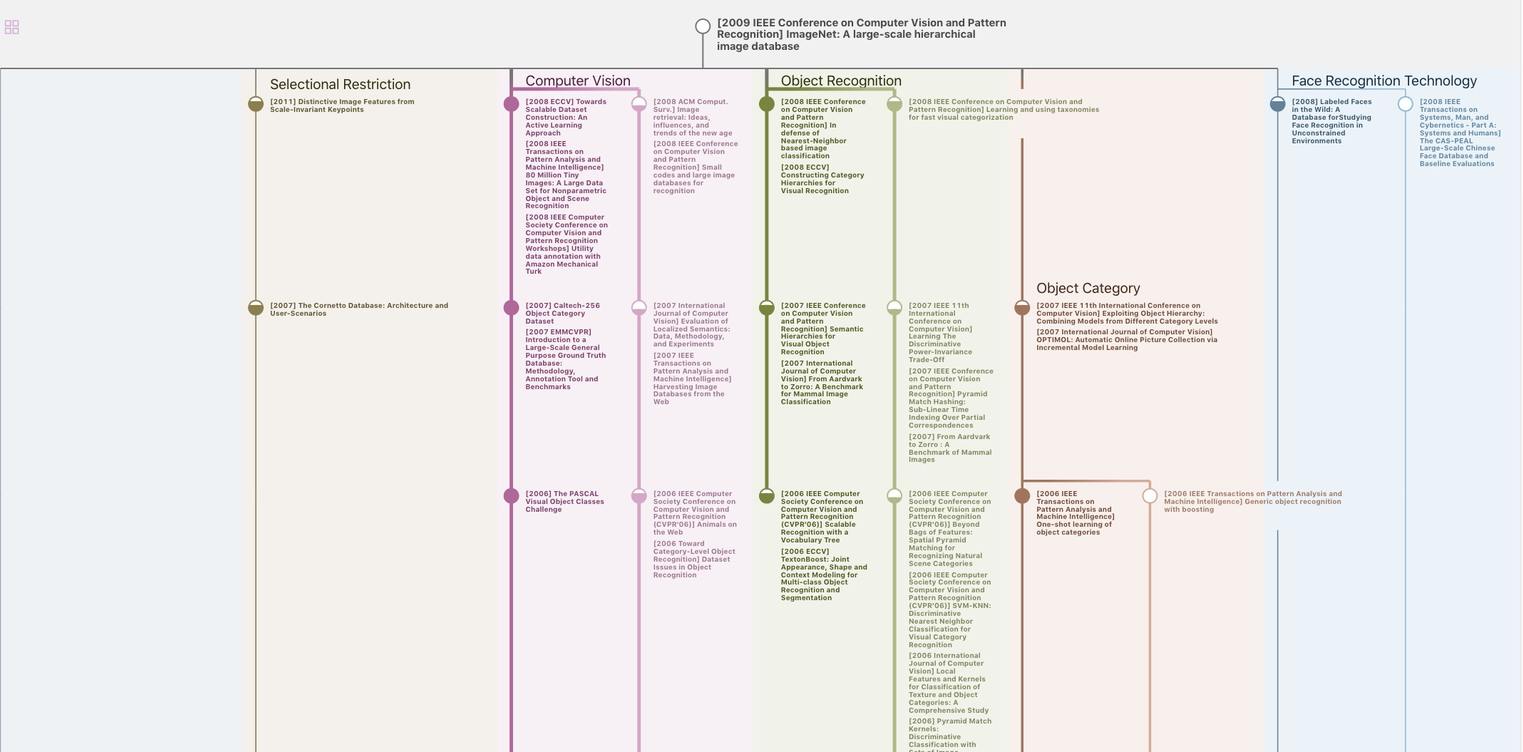
生成溯源树,研究论文发展脉络
Chat Paper
正在生成论文摘要