Reverse gamma correction based GARCH model for underwater image dehazing and detail exposure
Expert Systems with Applications(2023)
摘要
Underwater imaging poses significant challenges as water alters the behavior of light in comparison to air or vacuum. Therefore, it is crucial to effectively utilize the unique characteristics of unclear edges in hazy underwater images to achieve high-performance results in real-time applications. In this paper, we exploit such features as edges and visual perception in underwater haze images. To achieve this, we estimate the true transmission of the image by enhancing the visibility of discontinuous edges using the reverse gamma correction based on the Generalized Autoregressive Conditional Heteroscedasticity (GARCH) model. The parameters of the GARCH model are defined by the local and global pixel dynamics in adjacent neighborhoods, which improves the color orientation of the image while preserving image details. Additionally, we perform deseasoning to separate pixels while maintaining the natural contours of interactions between them. By considering the volatility of the RGB color channels, we predict the variance of the pixels based on the difference of the deseasoned pixels, which improves pixel intensity and allows for scene depth estimation. While estimating gamma correction and global ambient light, we deseasonalize image pixels based on their colors, enhancing the color of the final dehazed images. Using the greedy algorithm with a Convolutional Neural Network (CNN), our proposed method outperforms commonly used state-of-the-art methods.
更多查看译文
关键词
Underwater image dehazing, RGB color wavelength, Deseason, Ambient global underwater light, Scene depth
AI 理解论文
溯源树
样例
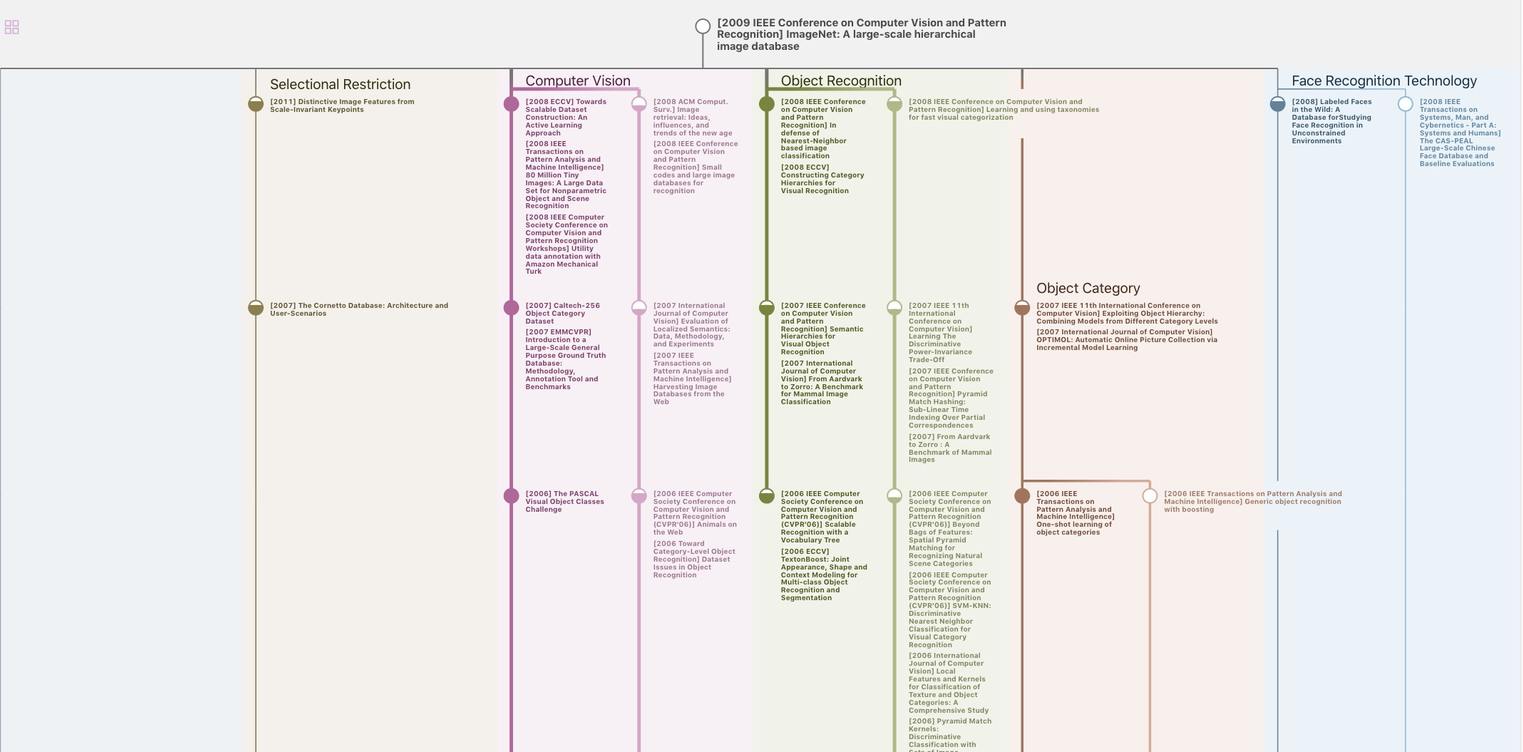
生成溯源树,研究论文发展脉络
Chat Paper
正在生成论文摘要