CWAN: Self-supervised learning for deep grape disease image composition
Engineering Applications of Artificial Intelligence(2023)
摘要
Grape leaf diseases seriously affect grape yield and directly lead to the loss of the industrial economy. An accurate diagnosis is crucial for effective spraying and control. Presently, the combination of image blending and a deep recognition model is the most effective method for accurately identifying grape leaf diseases. Existing methods of image blending depend primarily on single-channel and multi-scale channel blending, computing and optimizing the Poisson equation, and the iterative optimization of learning-based methods for simple composite results. Although these methods have had some success, they are not able to simultaneously address lesion and leaf gap size, color bleeding, and edge smoothness. In this paper, we propose a novel image blending method named cascade wavelet attention network (CWAN). Specifically, CWAN consists of a cascade wavelet attention (CWA) and a blending autoencoder. First, we build a CWA to decompose the image into a multi-level and multi-scale frequency domain image. Then, the detailed features of the source and target domains are extracted, and the edges are smoothed. Following this, a shared decoder is designed to fuse these features in the corresponding frequency-domain layers, and the result serves as the attention feature. Finally, we design a blending autoencoder with a feature fusion mechanism to seamlessly blend lesions and leaves enhanced by CWA. We also construct a weighted blended loss function to avoid leaf color infiltration into the lesion in the blended image. Comprehensive experiments on two grape leaf disease datasets. Qualitative and quantitative results demonstrate the superiority and effectiveness of our model.
更多查看译文
关键词
Grape leaf diseases,Data enhancement,Image blending,Self-supervised learning
AI 理解论文
溯源树
样例
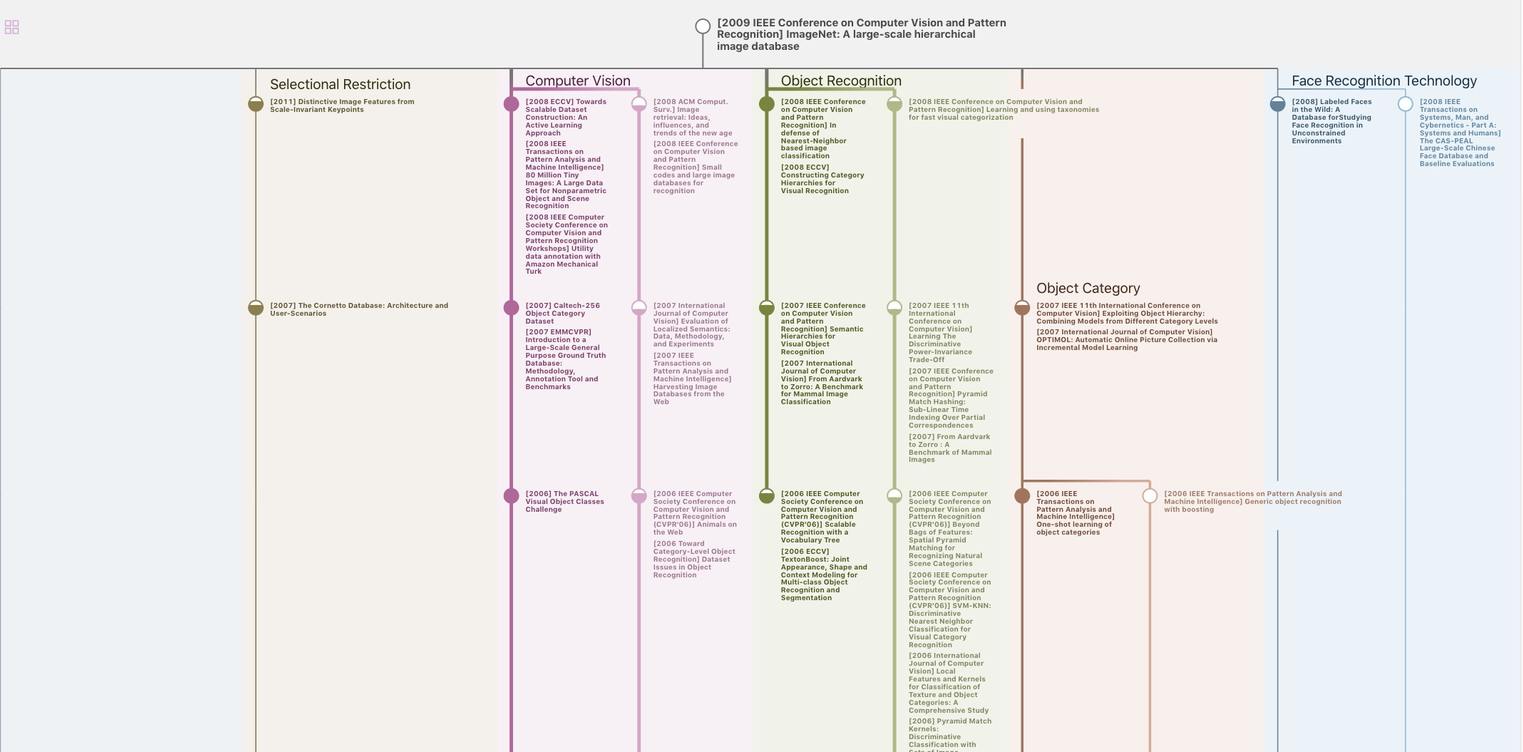
生成溯源树,研究论文发展脉络
Chat Paper
正在生成论文摘要