Supervised deep learning for the moving morphable components topology optimization framework
Engineering Applications of Artificial Intelligence(2023)
摘要
In the context of mechanical engineering design, the field of machine learning accelerated topology optimization is dominated by image-based models trained in a supervised manner on datasets with a limited diversity of boundary conditions. State-of-the-art methods show poor generalization capabilities and are strongly coupled to finite element mesh resolution, hindering scalability. In this paper, we leverage the explicit topology parameterization of the moving morphable components (MMC) framework to train a deep learning model that directly generates geometric design variables using a model architecture that is independent of the finite element mesh used for structural analysis. The developed model is trained on a large dataset of boundary conditions. Despite achieving state-of-the-art regression loss, evaluations reveal that direct-design approaches generate topologies with poor mechanical performance. Specifically, the model-generated topologies have, on average, a stiffness 11.48% lower than conventional MMC designs, as evidenced by in-distribution and out-of-distribution test samples. We demonstrate that this is due to the incompatibility between the regression loss function typically used in literature and the topology optimization objective of compliance minimization. To address this issue, we propose a novel acceleration approach that leverages the trained model to generate improved initial designs for conventional optimization. Specifically, the deep learning model is used to generate an initial design, which is then refined by conventional optimization to arrive at a final, optimal design. This approach shows a computation time-saving of 36.84% without sacrificing the final mechanical performance of the optimal topology compared to conventional optimization starting from a uniform initial layout.
更多查看译文
关键词
Topology optimization,Moving morphable components,Deep learning,Artificial intelligence
AI 理解论文
溯源树
样例
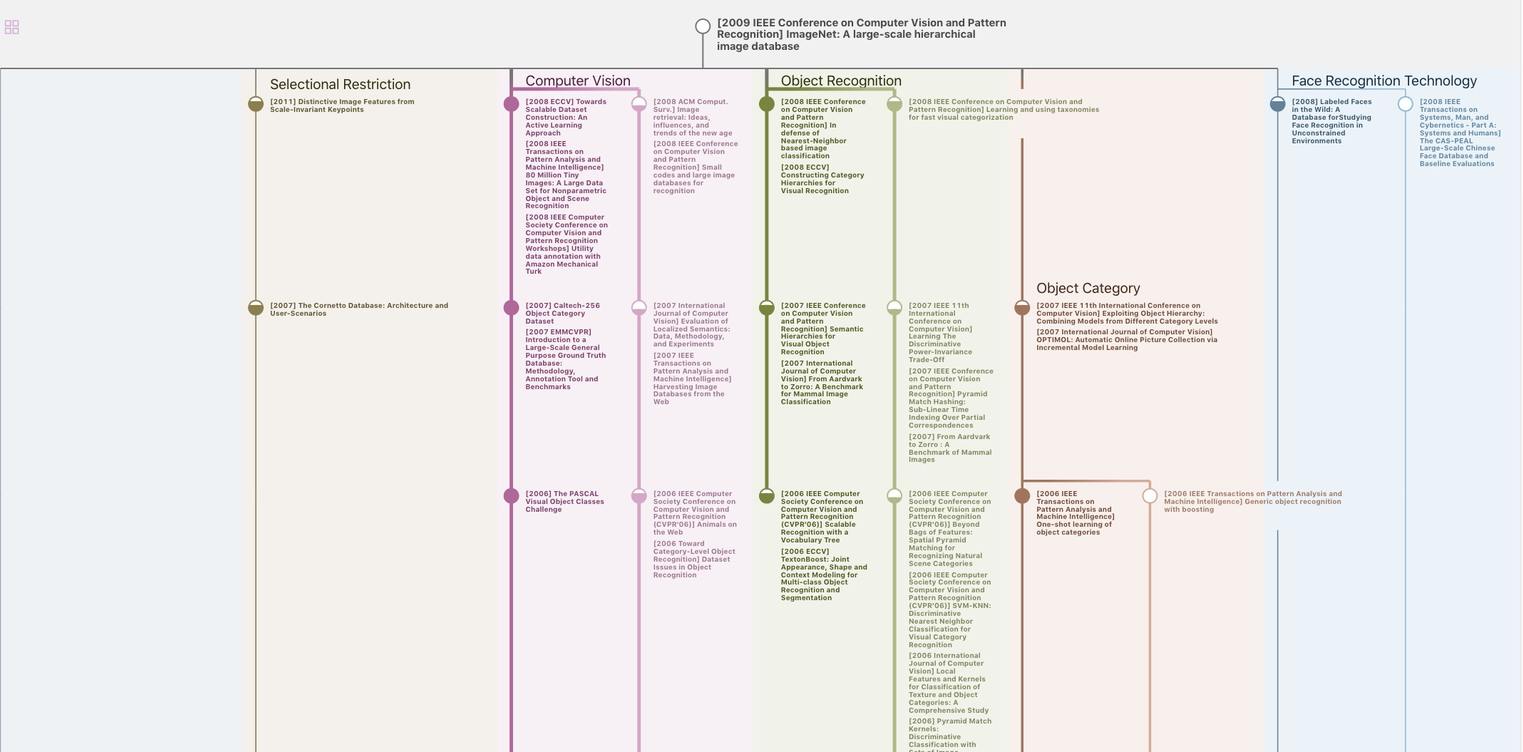
生成溯源树,研究论文发展脉络
Chat Paper
正在生成论文摘要