Interpretable federated learning for machine condition monitoring: Interpretable average global model as a fault feature library
Engineering Applications of Artificial Intelligence(2023)
摘要
Federated learning (FL) is an emerging technique used to prevent the two contradictory problems of data silos and data privacy. Different from centralized learning, FL makes it possible to learn a global model while private data are stored locally. Nevertheless, statistical heterogeneity is a major challenge that has not been well addressed in literature and the interpretability of the model is always ignored. In this paper, an interpretable FL framework is constructed for machine condition monitoring and fault diagnosis. Since fault characteristic frequencies (FCFs) and their harmonics are closely connected with specific machine fault types, an interpretable local client model is designed to identify the FCFs and their harmonics of different clients. Theoretical investigation on the additivity of local model parameters in this paper prove that learned local parameters from the frequency domain are actually FCFs and their harmonics and their additivity is capable of constructing a fault feature library, which is beneficial to providing different fault information and quickly diagnosing fault types. The effectiveness of the method is demonstrated by experiments with two independent bearing run-to-failure datasets.
更多查看译文
关键词
Federated learning, Fault diagnosis, Fault feature library, Fault characteristic frequency, Additivity of local model parameters
AI 理解论文
溯源树
样例
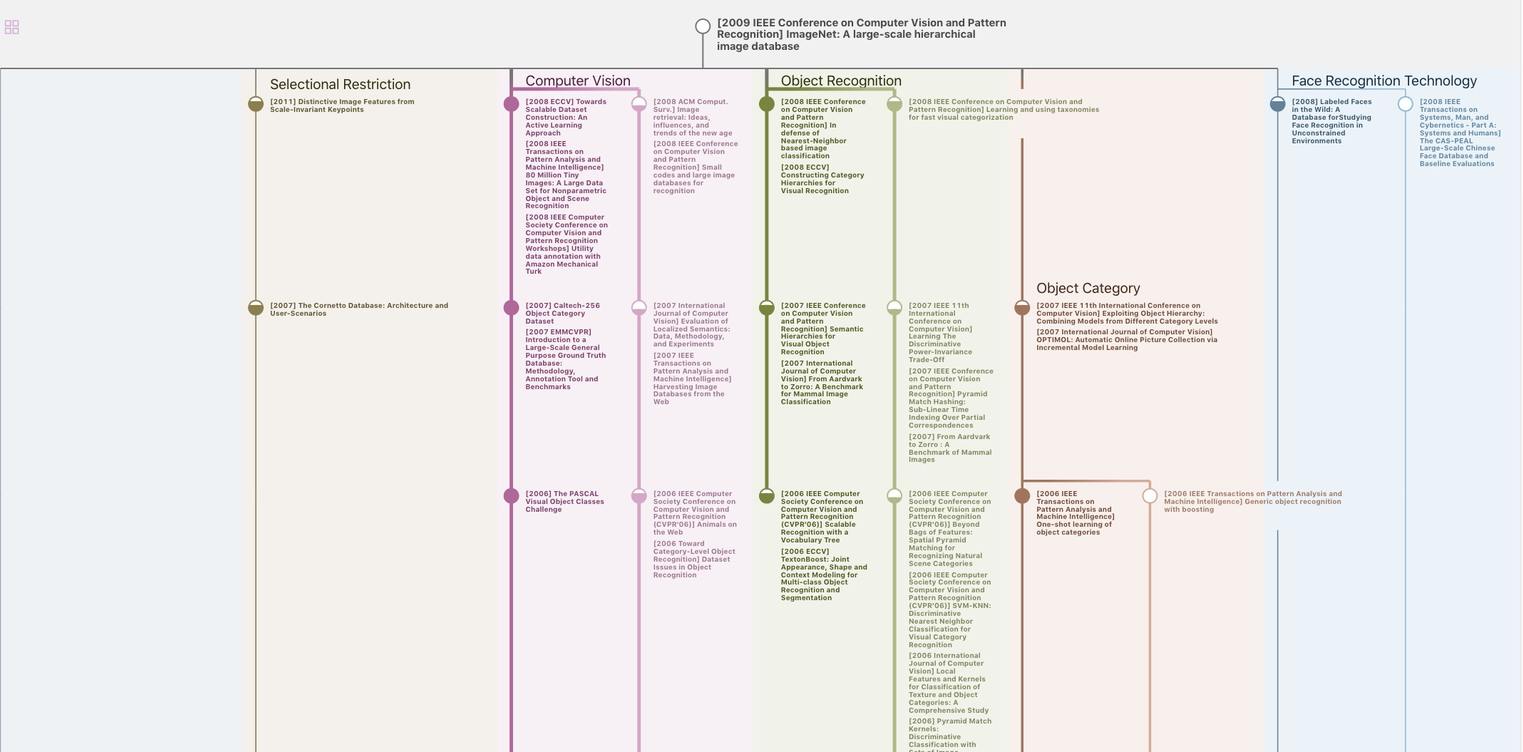
生成溯源树,研究论文发展脉络
Chat Paper
正在生成论文摘要