Fuzzy adaptive terminal sliding mode control based on recurrent neural network compensation for a maglev system
Engineering Applications of Artificial Intelligence(2023)
摘要
Reluctance motor has high force density while strong uncertainties. This paper concentrates on the research of the intelligent control method for a reluctance-motor maglev system (RMMS) with limited system information. To this end, we propose a new fuzzy adaptive terminal sliding mode control (FATSMC) method based on a novel full-regulated recurrent neural network (RNN) compensator. The RNN approximates the lumped uncertainty of the RMMS, and the controller handles the residual error and external disturbances. The presented increasing-decreasing fuzzy adaptive law, without the prior knowledge of uncertain upper bound, adjusts the switching gain by the dynamic information of the system. And the overestimation of the switching gain can be reduced. Moreover, the proposed method only uses the nominal parameters and model of the RMMS, thus avoiding complex modeling. This study provides strong support for the application of RMMS in high -precision situations. By constructing a Lyapunov function, the stability of the proposed method is analyzed, which is uniformly and ultimately bounded (UUB). In the experiment, the proposed method is compared with three existing methods in four cases. The results demonstrate that the presented method achieves nanoscale suspension accuracy, suppresses chattering significantly, and has strong robustness.
更多查看译文
关键词
Recurrent neural network (RNN),Fuzzy logic system (FLS),Adaptive terminal sliding mode control,Reluctance-motor maglev system
AI 理解论文
溯源树
样例
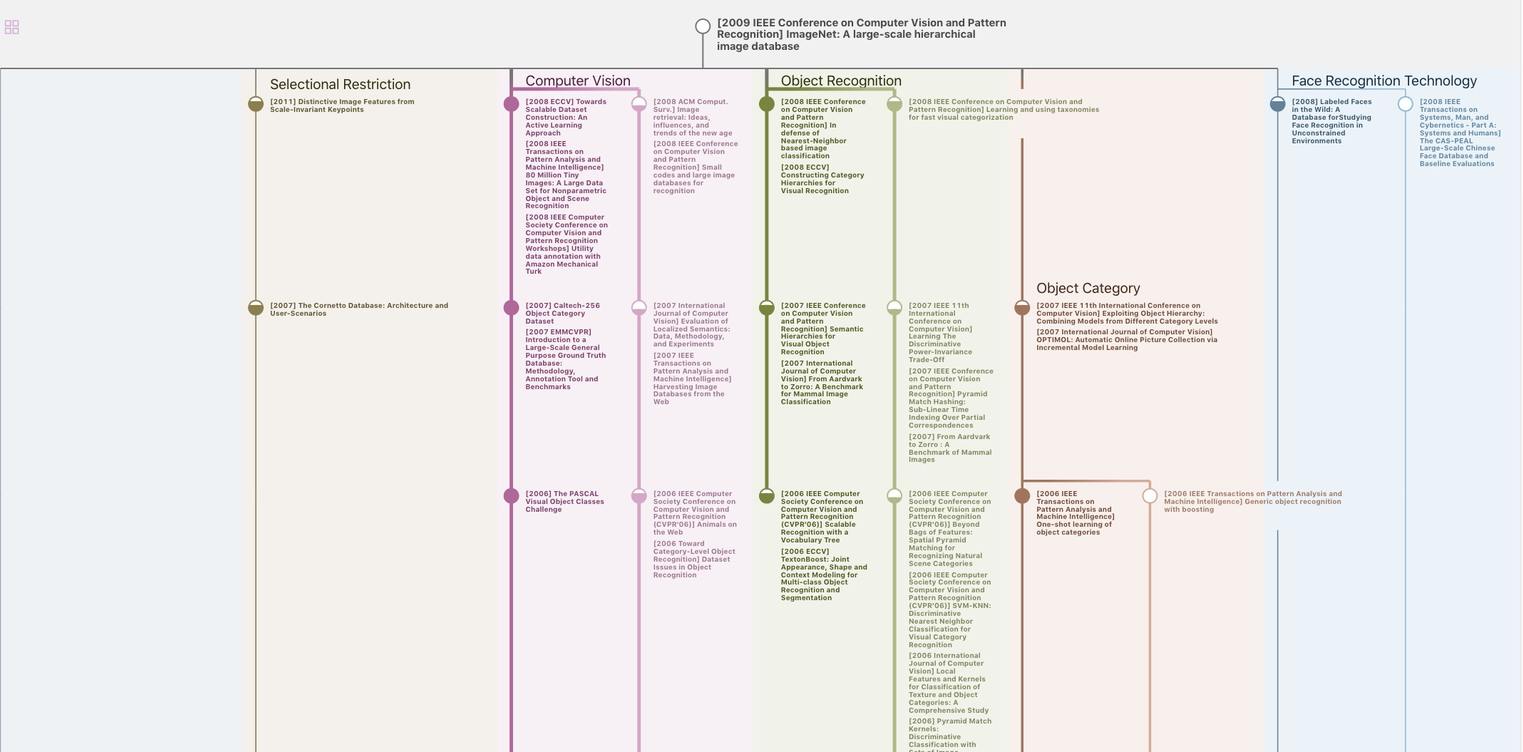
生成溯源树,研究论文发展脉络
Chat Paper
正在生成论文摘要