Spatiotemporal analysis of bidimensional wind speed forecasting: Development and thorough assessment of LSTM and ensemble graph neural networks on the Dutch database
Energy(2023)
摘要
The increasing concern about climate change and global warming led to the necessity to develop new energy sources. Within this scenario, wind power has become a major player due to its global availability and competitive prices. However, the intermittency and stochastic characteristics of the wind ultimately damper its efficient usage, increasing its operational costs. To mitigate this barrier, this work investigates the spatiotemporal phenomena underpinning wind speed and their usefulness when it comes to wind forecasting. We propose a new ensemble model based on Graph Attention Network (GAT) and GraphSAGE to predict wind speed in a bi-dimensional approach. The model was trained and validated using the Dutch dataset and considered several time horizons, timelags, and the impact of weather stations distributed across the country. To benchmark the results, the proposed model was compared against the persistence and LSTM models, as well as state-of-the-art paradigms such as LSSTM, GNN-GAT, and GNN-SAGE. The results showed that the ensemble SAGE-GAT was equivalent to or outperformed all benchmarking models and had lesser error values than those found in reference literature. The results also showed that the longer the horizon is to predict wind speed, the more relevant the spatial information passed from the stations is.
更多查看译文
关键词
Renewable energy, Wind energy, Wind speed, Forecasting, Machine learning, Graph neural networks
AI 理解论文
溯源树
样例
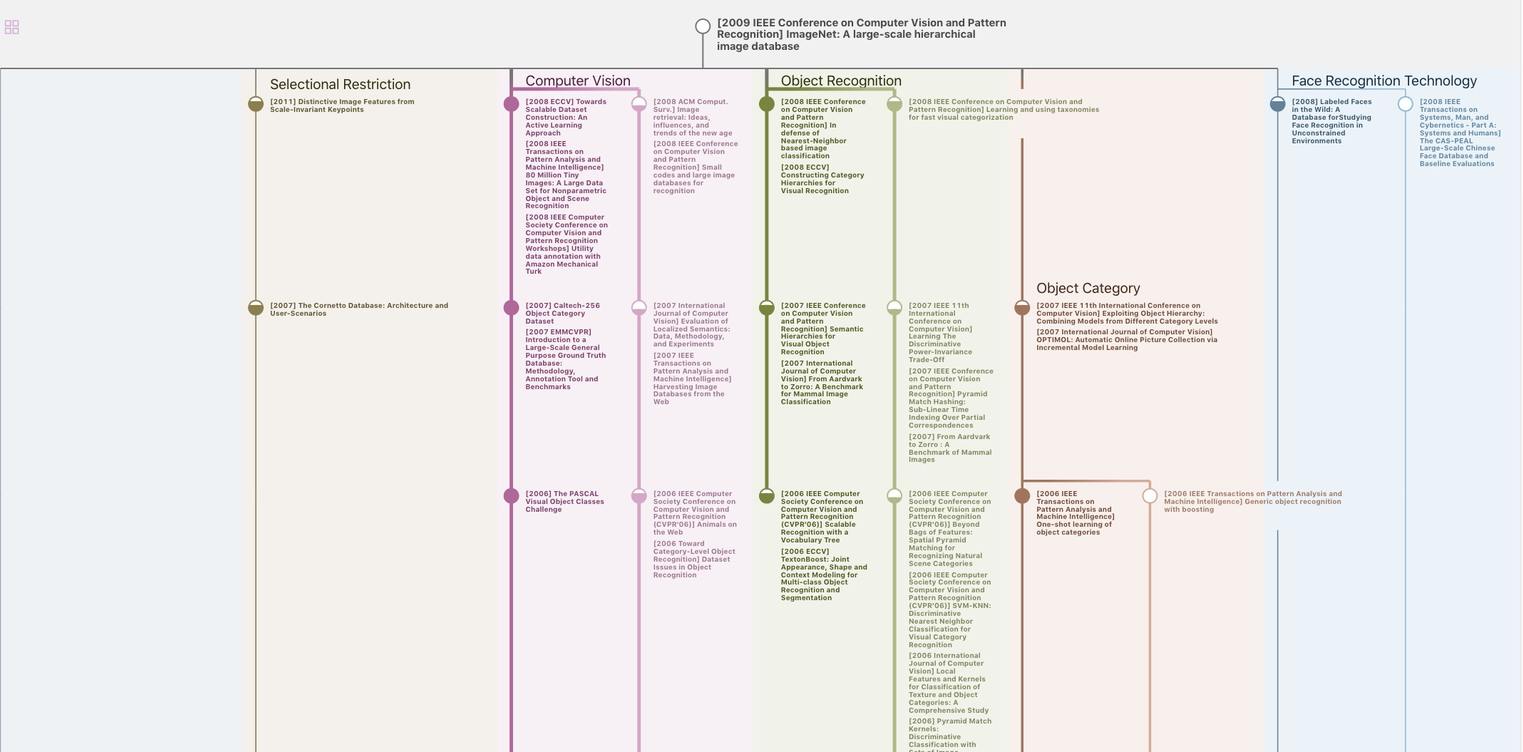
生成溯源树,研究论文发展脉络
Chat Paper
正在生成论文摘要