Sequential Bayesian inference for agent-based models with application to the Chinese business cycle
Economic Modelling(2023)
摘要
Agent-based models (ABMs), which model the evolving system through the interactive behavior of agents, are often challenged by inaccurate estimates. However, estimates provided by traditional approaches are sensitive to initial values due to irregular and multimodal likelihood functions. In this paper, we extend the Sequential Monte Carlo (SMC) algorithm for Bayesian posterior inference to ABMs, and used the kernel density estimation (KDE) to approximate the likelihood function. Our simulation results demonstrate that this method delivers advantages for parameters estimation in addressing multimodal likelihood, especially for large samples. Applying this approach to China’s macroeconomic data, we find that exogenous shocks in the agent-based model play a small role in explaining China’s business cycle. The SMC combined with KDE is straightforward to implement in empirical studies and yields estimates that align with the intuitions of ABMs, by mitigating estimation errors and reducing efficiency losses.
更多查看译文
关键词
C11,C13,C15
AI 理解论文
溯源树
样例
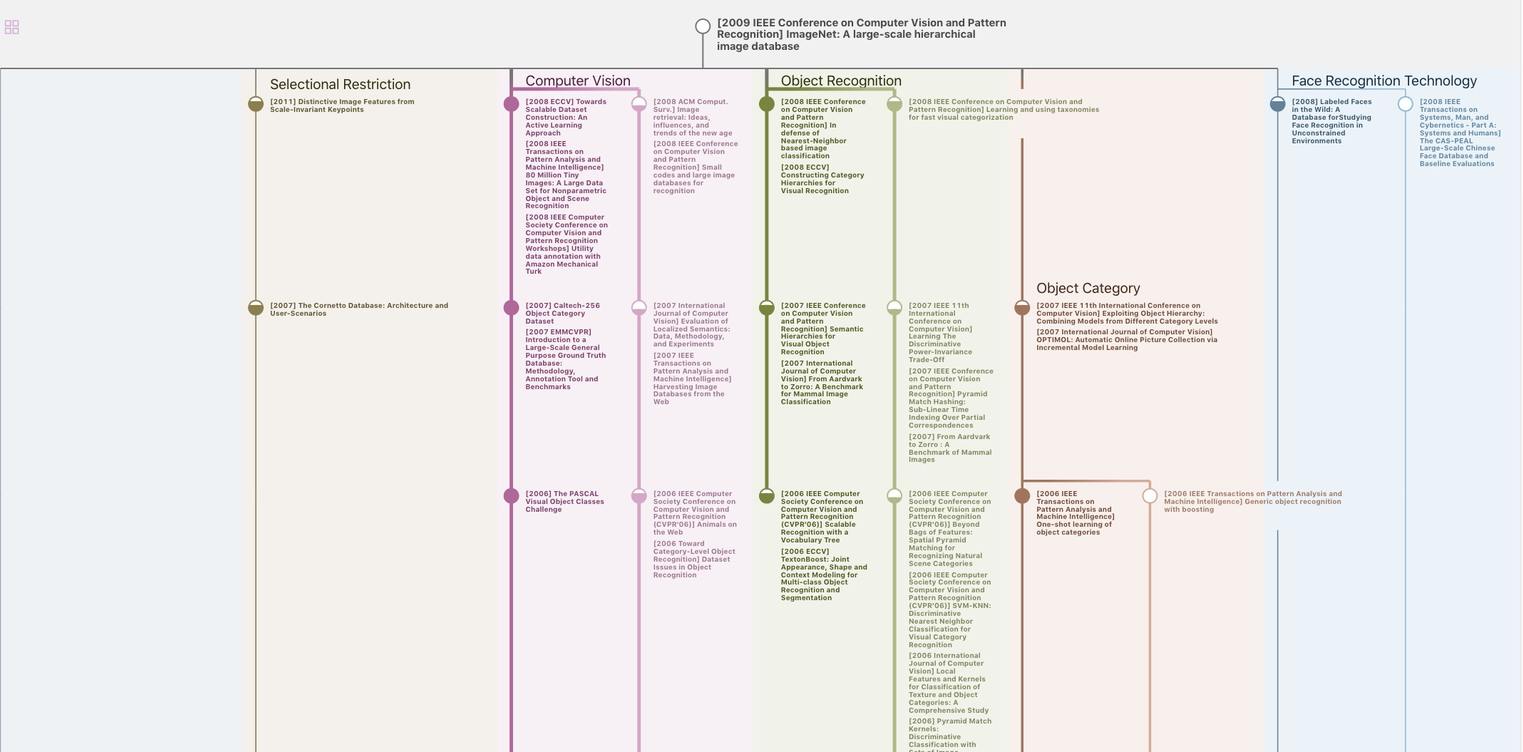
生成溯源树,研究论文发展脉络
Chat Paper
正在生成论文摘要