Diverse classifiers with label dependencies for long-tail relation extraction in big data
Computers and Electrical Engineering(2023)
摘要
Relation extraction is a critical step in knowledge recommendation for big data, but long-tailed distribution in real-world relations presents a significant challenge. Most relations fail to gather enough training instances, which forms a long tail in data distribution and leads to poor performance on these relations. Previous studies have made efforts to improve models for long-tailed relations by sharing knowledge from head classes to the long-tail. Despite proven effectiveness on long tail relations, this line of work lacks control over the knowledge transfer process, which can harm performance on head classes. To address this issue, we propose an approach that enhances the label hierarchical dependencies of a classifier through label-to-sentence attention with multi-granular constraints across different levels of relation. Moreover, We introduce an ensemble mechanism that uses a router module to balance performance between head and tail classes, thereby fixing the long-tail problem on relation extraction data set. Our approach achieves excellent performance on long-tail and all relations on the large-scale benchmark data set New York Times, without sacrificing performance on head relations. The experiment results demonstrate that our approach effectively alleviates long-tail problems and boosts performance on long-tail classes in relation extraction without harming the performance on head classes.
更多查看译文
关键词
Natural language processing,Information extraction,Relation extraction,Long-tail data,Knowledge representation
AI 理解论文
溯源树
样例
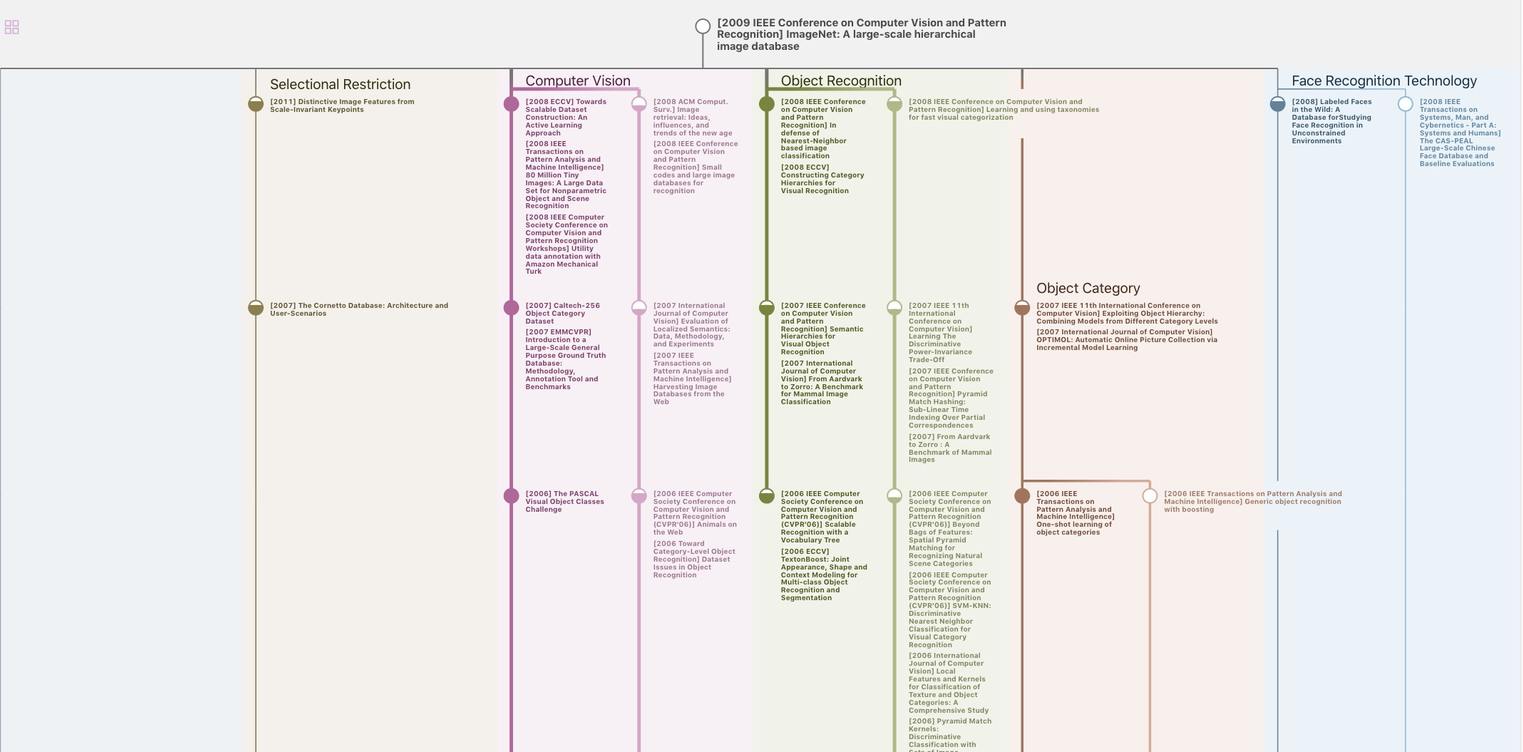
生成溯源树,研究论文发展脉络
Chat Paper
正在生成论文摘要