Sequential sparse autoencoder for dynamic heading representation in ventral intraparietal area
Computers in Biology and Medicine(2023)
摘要
To navigate in space, it is important to predict headings in real-time from neural responses in the brain to vestibular and visual signals, and the ventral intraparietal area (VIP) is one of the critical brain areas. However, it remains unexplored in the population level how the heading perception is represented in VIP. And there are no commonly used methods suitable for decoding the headings from the population responses in VIP, given the large spatiotemporal dynamics and heterogeneity in the neural responses. Here, responses were recorded from 210 VIP neurons in three rhesus monkeys when they were performing a heading perception task. And by specifically and separately modelling the both dynamics with sparse representation, we built a sequential sparse autoencoder (SSAE) to do the population decoding on the recorded dataset and tried to maximize the decoding performance. The SSAE relies on a three-layer sparse autoencoder to extract temporal and spatial heading features in the dataset via unsupervised learning, and a softmax classifier to decode the headings. Compared with other pop-ulation decoding methods, the SSAE achieves a leading accuracy of 96.8% & PLUSMN; 2.1%, and shows the advantages of robustness, low storage and computing burden for real-time prediction. Therefore, our SSAE model performs well in learning neurobiologically plausible features comprising dynamic navigational information.
更多查看译文
关键词
Heading,Neural decoding,Neural dynamics,Sparse autoencoder,Ventral intraparietal area,Vestibular
AI 理解论文
溯源树
样例
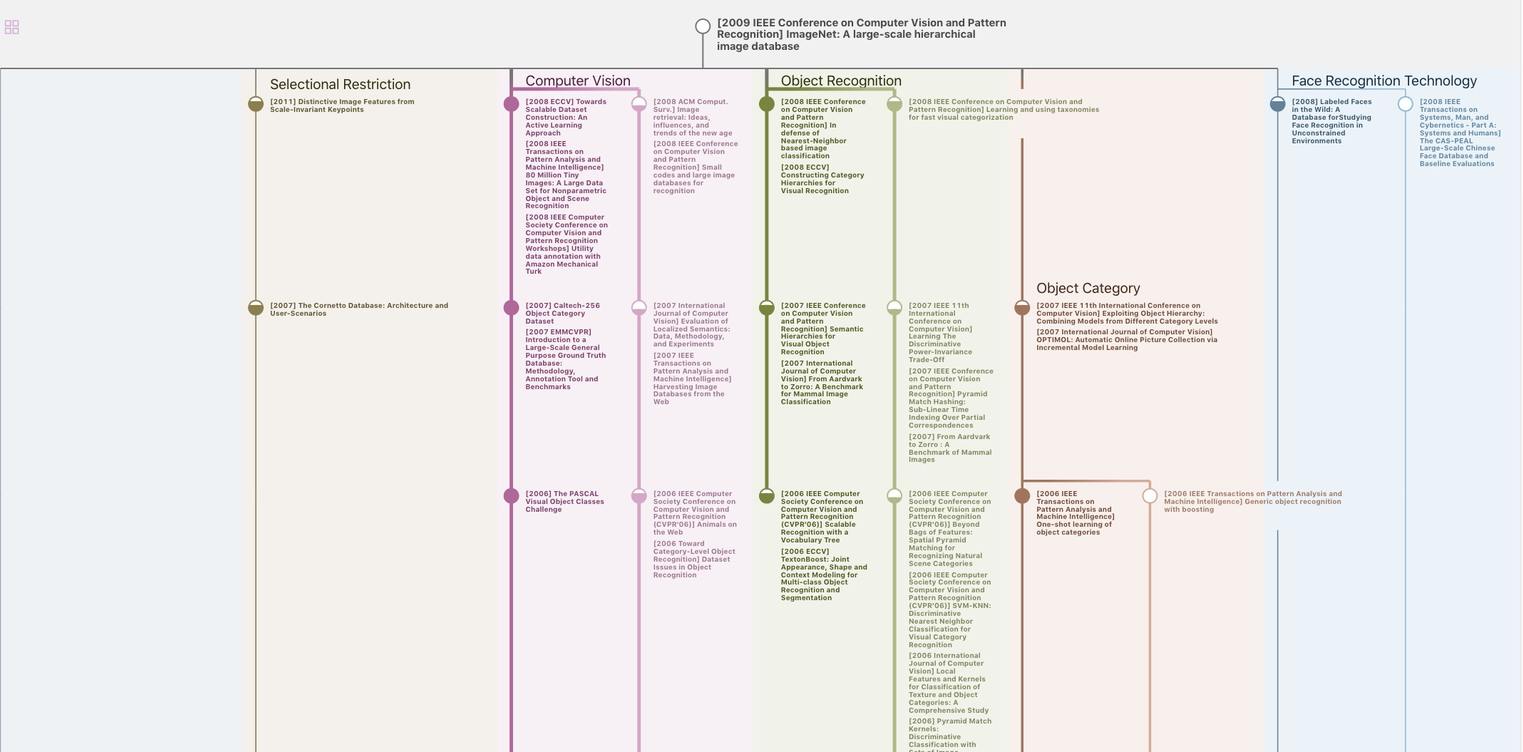
生成溯源树,研究论文发展脉络
Chat Paper
正在生成论文摘要