An explainable machine learning model for superalloys creep life prediction coupling with physical metallurgy models and CALPHAD
Computational Materials Science(2023)
摘要
Data-driven research mode plays an increasingly important role in scientific research. In this study, a dimensionality reduction strategy coupling with physical metallurgy models and CALPHAD method was proposed to established a machine learning model for Ni-based single crystal creep life prediction. SHAP analysis was applied to explain the internal mechanisms and the final results of the model. The results showed that the model was of good prediction accuracy and its prediction results could be reasonably explained. Thus, the model can be applied to predict the creep lives of engineering-applied superalloys and to search for the relationship between microstructures and creep lives of superalloys, which is expected to be applied to the design of new alloy.
更多查看译文
关键词
Creep life prediction,Explainable machine learning,Physical metallurgy models,CALPHAD
AI 理解论文
溯源树
样例
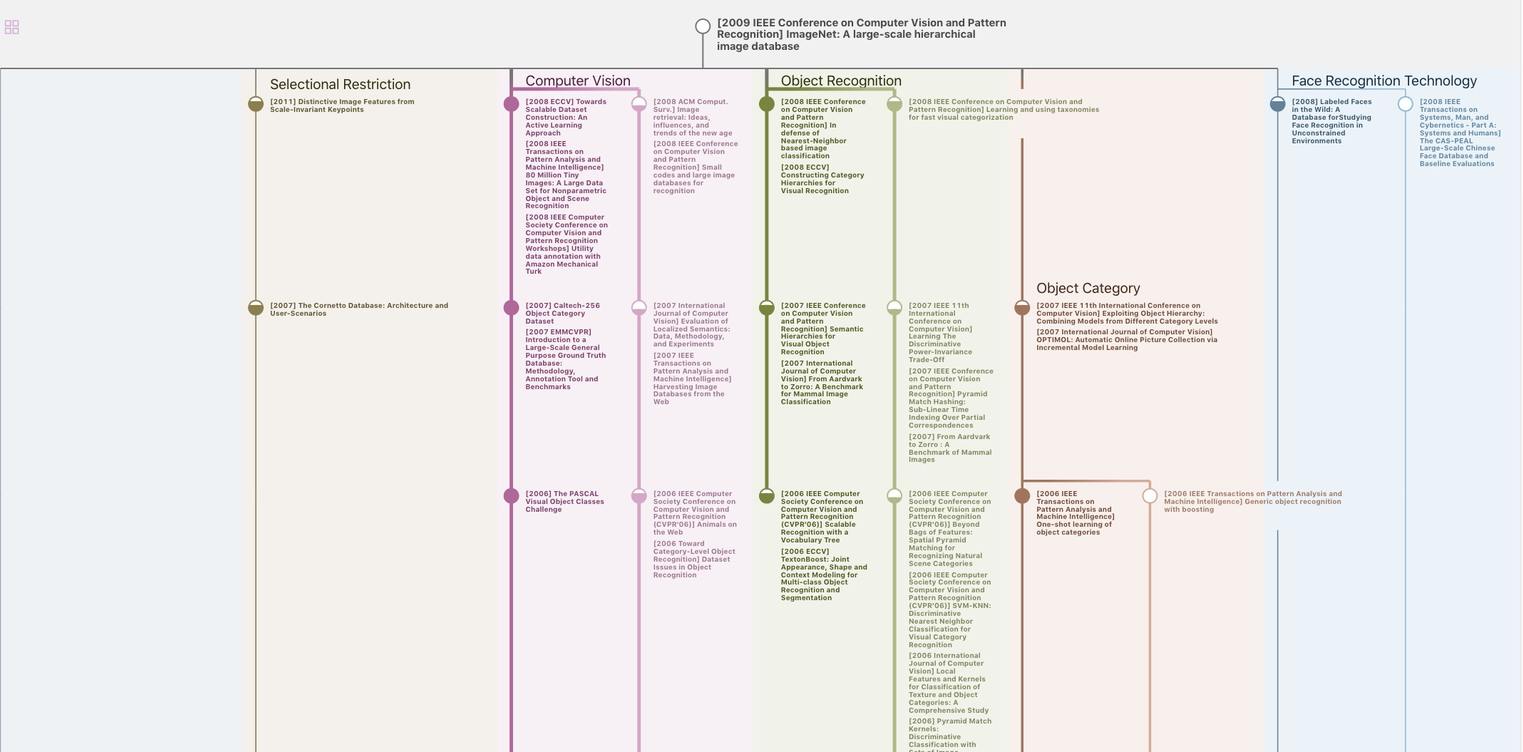
生成溯源树,研究论文发展脉络
Chat Paper
正在生成论文摘要