A novel physics-informed neural networks approach (PINN-MT) to solve mass transfer in plant cells during drying
Biosystems Engineering(2023)
摘要
Predicting microscale mechanisms of plant-based food materials has been an enduring challenge due to the inherent complexity of involved physics and prohibitively-high computational costs. As an alternative to conventional methods of solving corresponding Partial Differential Equations (PDEs), Physics-Informed Neural Networks (PINNs) have recently received significant attention from the research community. This article investigates the feasibility of PINNs' Automatic Differentiation (AD) to solve mass transfer (PINN-MT) and effectively predict cellular-level mass loss and subsequent moisture variations during low-temperature drying. PINN-MT has been developed by incorporating the residuals of convective mass transfer equation, Fick's law of diffusion and relevant initial conditions into the loss function. The integration of Adaptive Activation (AA) and Transfer Learning (TL) leads to improved computational efficiency, prediction accuracy and consistency. To the best of the authors' knowledge, this study is the first of its kind to apply PINNs' AD to solve mass transfer for food-drying. The PDE-solving capacity of the proposed approach has been compared with that of the state-of-the-art Finite Element Analysis (FEA) techniques. Compared to benchmark FEA results, percentage errors for PINN-MT-based mass loss and moisture distribution predictions remain below 0.23% and 0.33%, respectively. In addition, TL enhances the solution-based prediction efficiency of PINN-MT by more than 80%. Further, a novel approach has been proposed to achieve time-efficient predictions by feeding mass transfer coefficient-hm as an independent input to the proposed predictive framework, which has the potential to tackle complex and highly non-linear physics-based models, where conventional computational approaches usually struggle. (c) 2023 IAgrE. Published by Elsevier Ltd. All rights reserved.
更多查看译文
关键词
neural networks,drying,neural networks approach,plant cells,mass transfer,physics-informed
AI 理解论文
溯源树
样例
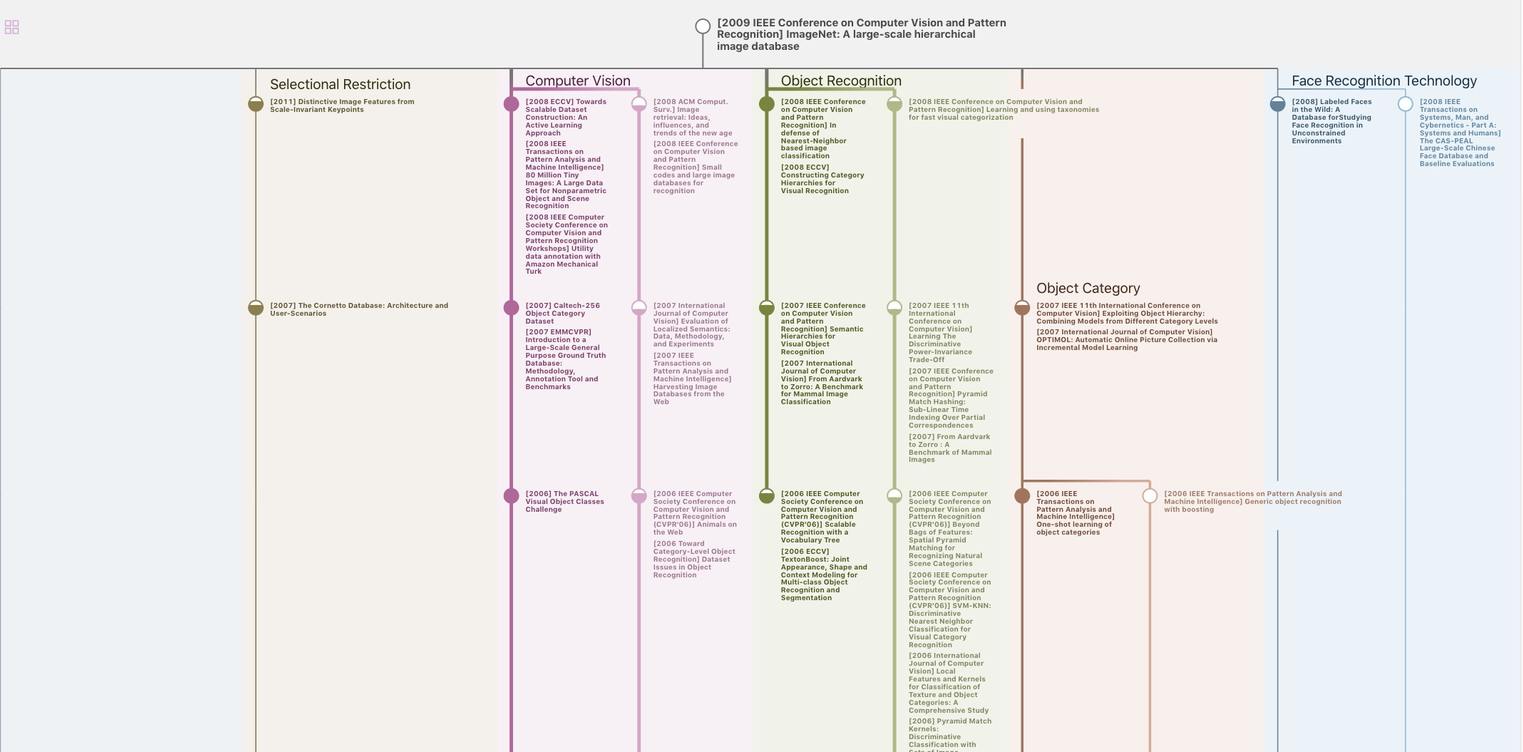
生成溯源树,研究论文发展脉络
Chat Paper
正在生成论文摘要