Improved ReliefF-based feature selection algorithm for cancer histology
Biomedical Signal Processing and Control(2023)
摘要
Global cancer statistics show that breast cancer accounts for 11.6% of mortality, second only to lung cancer, and is the second most deadly disease in the world. Due to the frequent occurrence of cancer and the different clinical presentation of the same cancer, genetic diagnosis has become a key technology for accurate prediction of the disease. Due to the low-sample, high-dimensional and high-noise characteristics of genetic data, which lead to bias in prediction, the algorithm cannot guarantee the efficient and accurate predictive power in practical applications. Therefore, this paper proposes an improved ReliefF-based feature selection algorithm for cancer histology (REDFS), which introduces difference coefficients to improve the stability of feature selection while expanding the differences between similar and dissimilar samples, proposes to calculate the weight of each feature with the weight of Jaccard’s similarity coefficient, and also realizes weight update to retain the most critical feature subsets to achieve the best feature subset selection. To prove the effectiveness of the algorithm, this study conducted a large number of comparative experiments on three cancer datasets, and the experimental results showed that the algorithm has better classification performance. Taking breast cancer as an example, the dataset was typed, and the resulting subtypes were fused with clinical data while correlation analysis was performed to obtain the survival curve of this patient, and finally the enriched ontology (GO) terms and biological pathways were obtained.
更多查看译文
关键词
Breast cancer, Omics data, Deep learning, Feature selection, Survival analysis
AI 理解论文
溯源树
样例
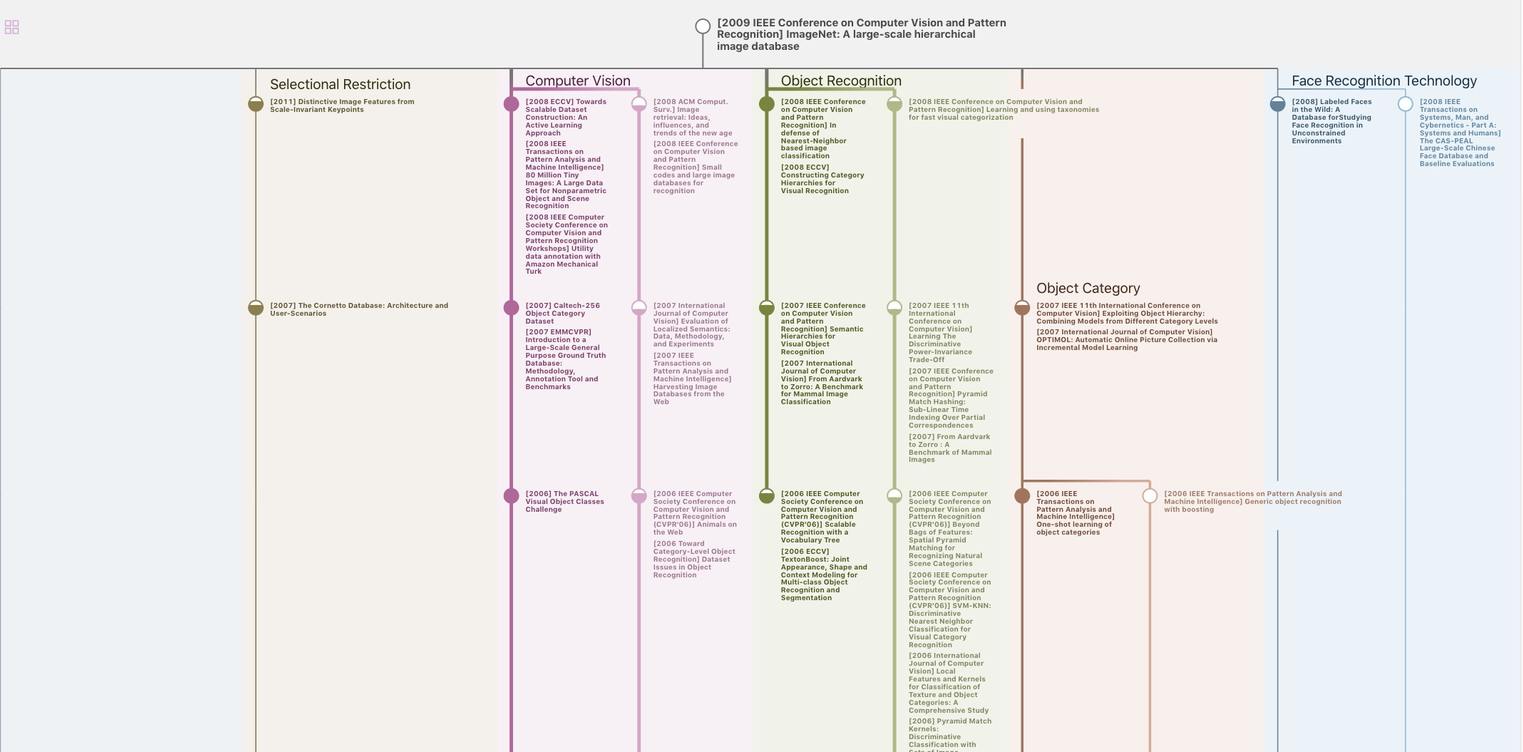
生成溯源树,研究论文发展脉络
Chat Paper
正在生成论文摘要