Data security of machine learning applied in low-carbon smart grid: A formal model for the physics-constrained robustness
Applied Energy(2023)
摘要
Towards the low-carbon goal, a smart grid features remote connection, data sharing, and cyber–physical integration to increase the flexibility of energy supplies, to reduce electricity wastage, and to enhance safety under intermittent renewables. In this context, machine learning (ML) is increasingly employed in smart grids to solve tasks that require deep data analytics. However, it is well recognized that ML models are vulnerable to adversarial examples that might be contained in open shared data, which creates opportunities for potential attackers to launch cyberattacks. In particular, system operating states acting as critical inputs to ML-based smart grid applications (MLsgAPPs) can be manipulated by malicious actors to mislead the system operator into making wrong decisions that in turn may cause major blackouts and other damaging cascading events. It is thus imperative to advance vulnerability assessment for MLsgAPPs.
更多查看译文
关键词
smart grid,robustness,machine learning,security,low-carbon,physics-constrained
AI 理解论文
溯源树
样例
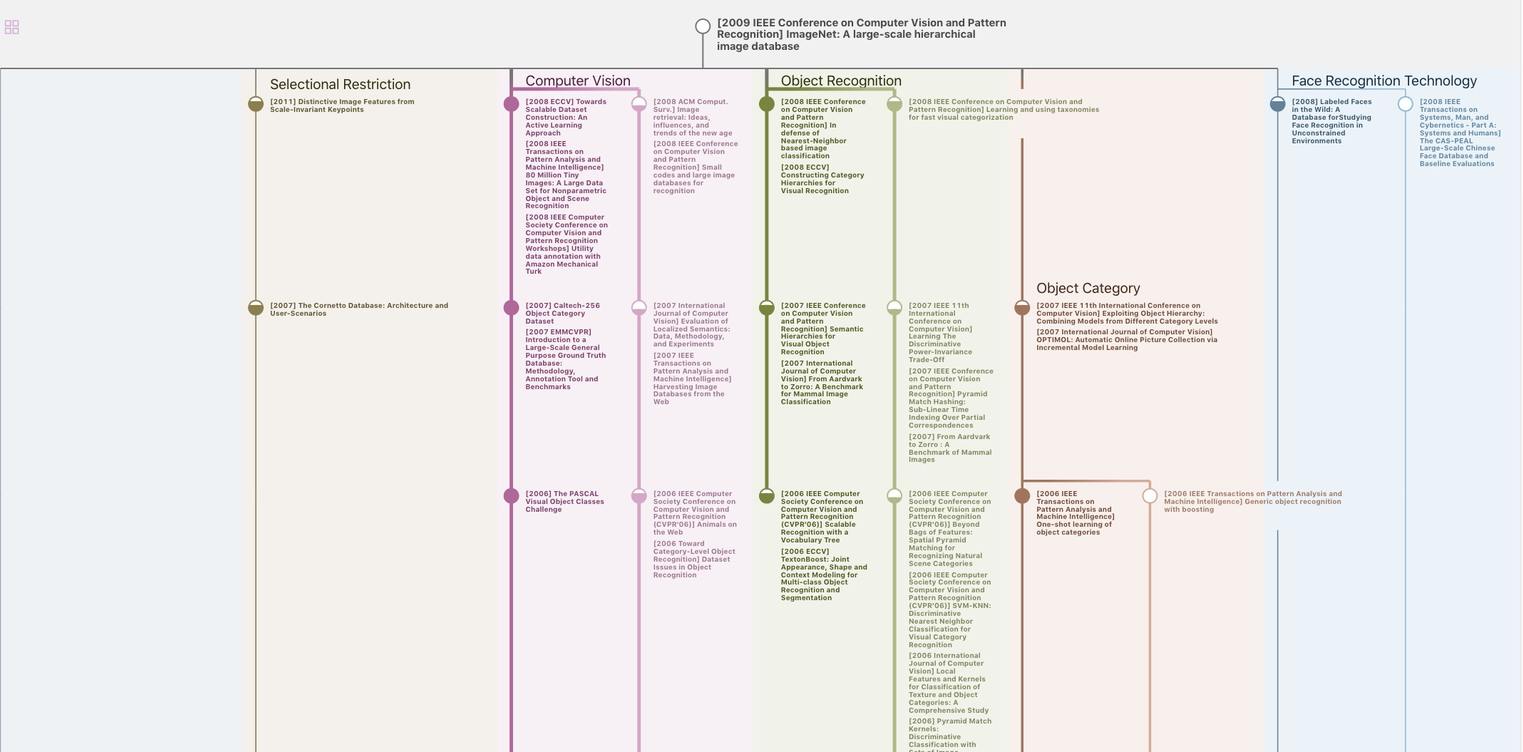
生成溯源树,研究论文发展脉络
Chat Paper
正在生成论文摘要