FGSS: Federated global self-supervised framework for large-scale unlabeled data
Applied Soft Computing(2023)
摘要
Due to the unique advantages of collaborative learning on isolated yet unlabeled data, federated self-supervised learning has received increasing attention from both academic and industrial researchers. Most of the existing federated self-supervised approaches concentrate on the classical scenario, i.e., a large amount of unlabeled data is stored on the clients. However, in many real-world applications, partial labels may be available to the client user, while a large amount of unlabeled data remains on the server side. The existing federated self-supervised methods may usually have difficulty in addressing this scenario. In this paper, we propose a creative federated global self-supervised framework (FGSS) for large-scale unlabeled data that innovatively uses self-supervised learning on the server side, and during every round of communication, we use a small amount of labeled data from the client to facilitate the performance of self-supervised learning. To address the heterogeneity of local data from different clients, we designed an aggregation approach that can adjust the weight of each local model based on the frequency of participation in the communication and the size of its dataset. Experimental results show that our framework outperforms the most existing state-of-the-art methods in both IID and non-IID settings under certain conditions.& COPY; 2023 Elsevier B.V. All rights reserved.
更多查看译文
关键词
Federated learning,Self-supervised learning,non-IID data,Aggregation method
AI 理解论文
溯源树
样例
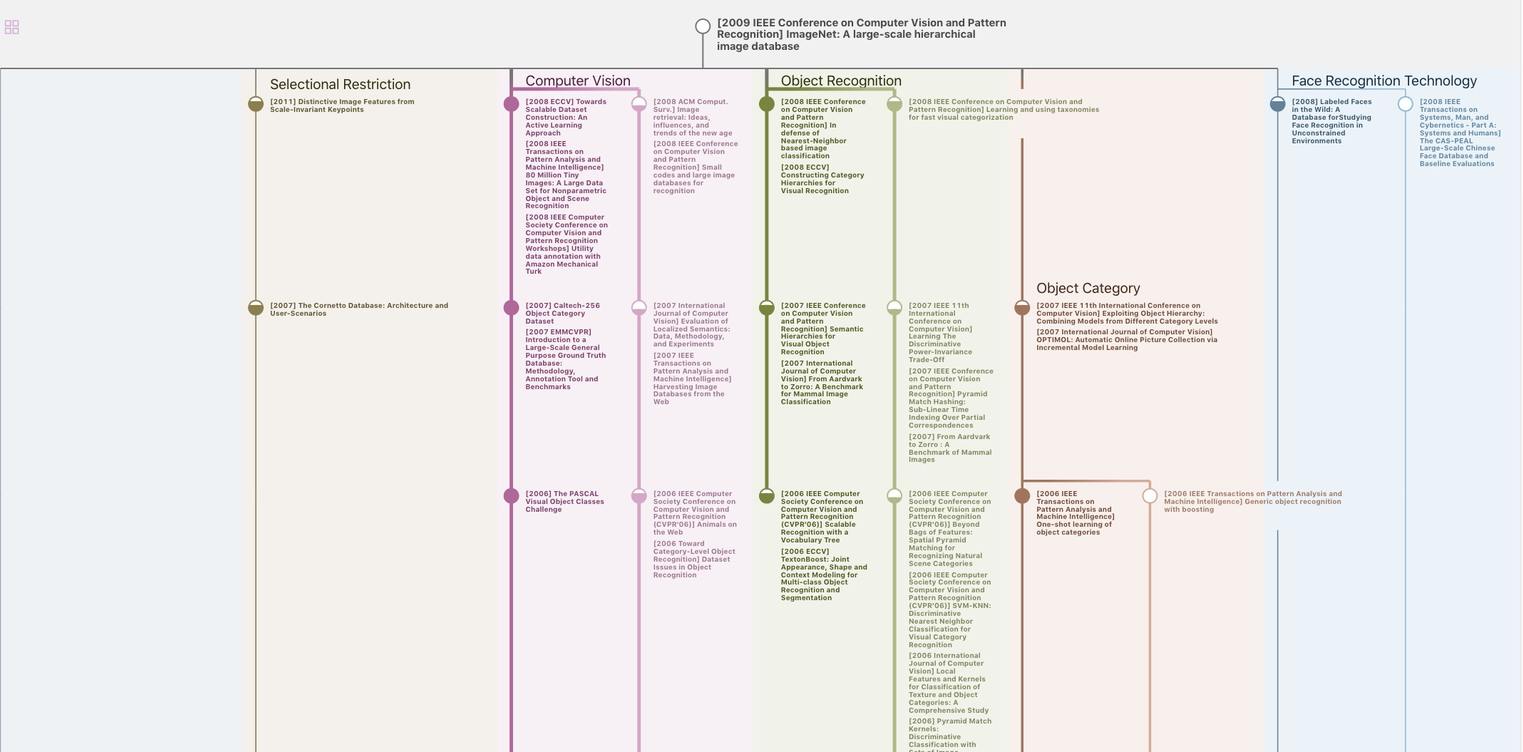
生成溯源树,研究论文发展脉络
Chat Paper
正在生成论文摘要