A class-specific feature selection and classification approach using neighborhood rough set and K-nearest neighbor theories
Applied Soft Computing(2023)
摘要
Rough set theories are utilized in class-specific feature selection to improve the classification performance of continuous data while handling data uncertainty. However, most of those approaches are converting continuous data into discrete or fuzzy data before applying rough set theories. These data conversions can reduce or change the meaning of data, as well as introduce unnecessary complexity to the feature domain. Therefore, in this study, we use neighborhood rough sets in class-specific feature selection to improve the classification performance without data conversions. As the standard classification algorithms are capable of handling a single feature set, we propose a novel classification algorithm based on the K-Nearest Neighbour algorithm to use class-specific feature subsets. Experimental evaluations prove that the proposed approach outperforms most of the baselines, and the selected feature sets are more effective than using the full feature sets in classification. The approach highly reduces the number of selected features and hence, can be used for effective data analysis of continuous data with high performance.
更多查看译文
关键词
Class-specific,Feature selection,Neighborhood rough set,Continuous data,Classification,Data conversion
AI 理解论文
溯源树
样例
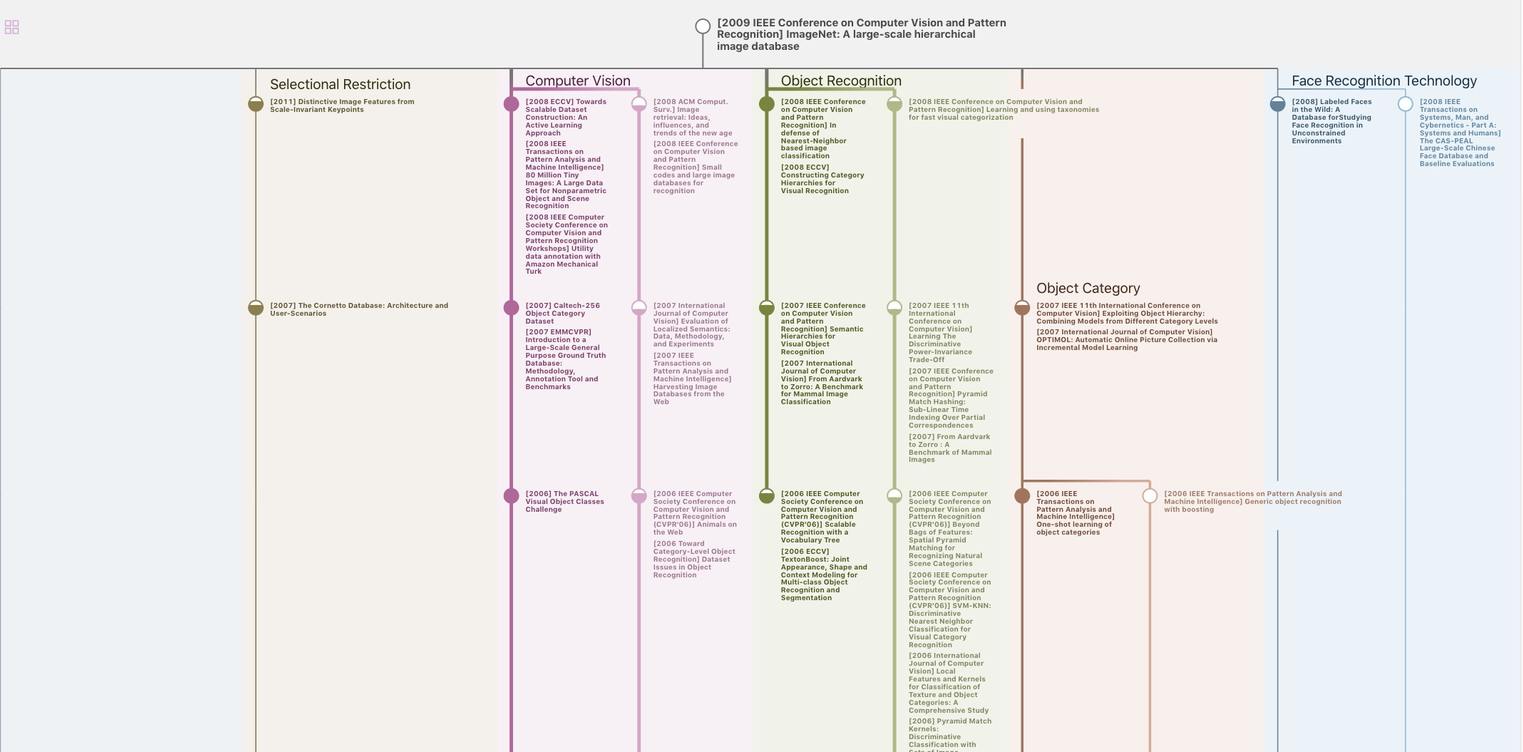
生成溯源树,研究论文发展脉络
Chat Paper
正在生成论文摘要