Fast electrical impedance tomography based on sparse Bayesian learning
Applied Soft Computing(2023)
摘要
Electrical impedance tomography (EIT) is a severely under-determined and ill-posed inverse problem. Therefore, based on the compressed sensing theory and the block sparse Bayesian learning (BSBL) model, a novel accelerated EIT sparse imaging algorithm is presented. The key feature of the proposed algorithm is that the convex–concave procedure (CCP) method is used to optimize the non-convex cost function of the block sparse Bayesian learning model which improves the convergence speed and reduces the time complexity of the algorithm. The proposed method can adaptively explore and utilize the inter-block sparsity and intra-block structural correlation of non-sparse signals without any a priori information, thereby sparse imaging is performed on non-sparse physiological data effectively. The results comparing with other methods show that the proposed algorithm not only reconstruct inclusions with different shapes and conductivity applicably and effectively but also reduce the running time of the algorithm.
更多查看译文
关键词
Electrical impedance tomography,Compressed sensing,Block sparse Bayesian learning,Image reconstruction,Sparse imaging
AI 理解论文
溯源树
样例
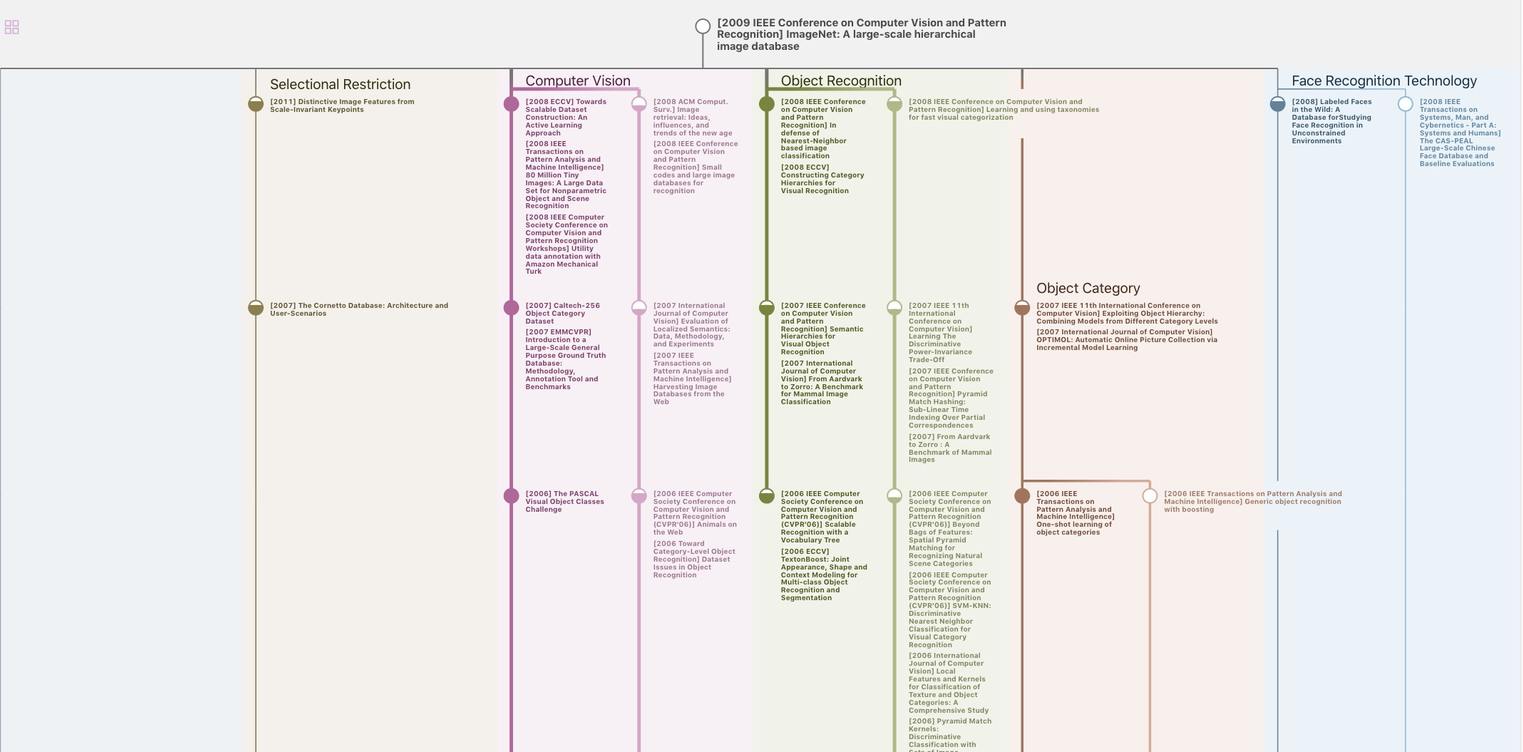
生成溯源树,研究论文发展脉络
Chat Paper
正在生成论文摘要