Convolutional-neural-network-based multi-signals fault diagnosis of induction motor using single and multi-channels datasets
Alexandria Engineering Journal(2023)
摘要
Using deep learning in three-phase induction motor fault diagnosis has gained increasing interest nowadays. This paper proposes a Convolutional Neural Network (CNN) model to diagnose induction motor faults during the starting period. The model is able to detect various faults (locked-rotor, overload, voltage-unbalance, overvoltage, and undervoltage) under three loading levels (Light, Normal, and Heavy loads). The proposed model has high reliability as it depends on image data from multiple motor signals (voltages, currents, torque, and speed). The transient response of the motor starting period, makes it challenging to diagnose faults depending on raw time-domain images only. Therefore, the measured signals are additionally represented as d-q, and Lissajous images to focus on the effect of using these representations on the model performance. With multi-signals data, two forms of data (single- and multi-channel input-shape) can be implemented and evaluated to figure out the form that can capture the related fault features more efficiently. Experiments are performed using simulated machine model, to investigate the best signal representation and the best input shape for the proposed fault diagnosis system. Eight distinct datasets are generated from the motor data to be used in training and testing the CNN model. For the comparison of signals representations, the best accuracy is achieved with datasets containing both voltage and current d-q signals representation. They performed better than the other representations in the range from (0.03%) to (3.57%). For the comparison of input shapes, all multi-channels datasets proved to have better performance than single-channel datasets in the range from (0.77%) to (4.06%).
更多查看译文
关键词
Induction motor, Fault diagnosis, Deep learning, CNN, Multi-signals, Multi-channels
AI 理解论文
溯源树
样例
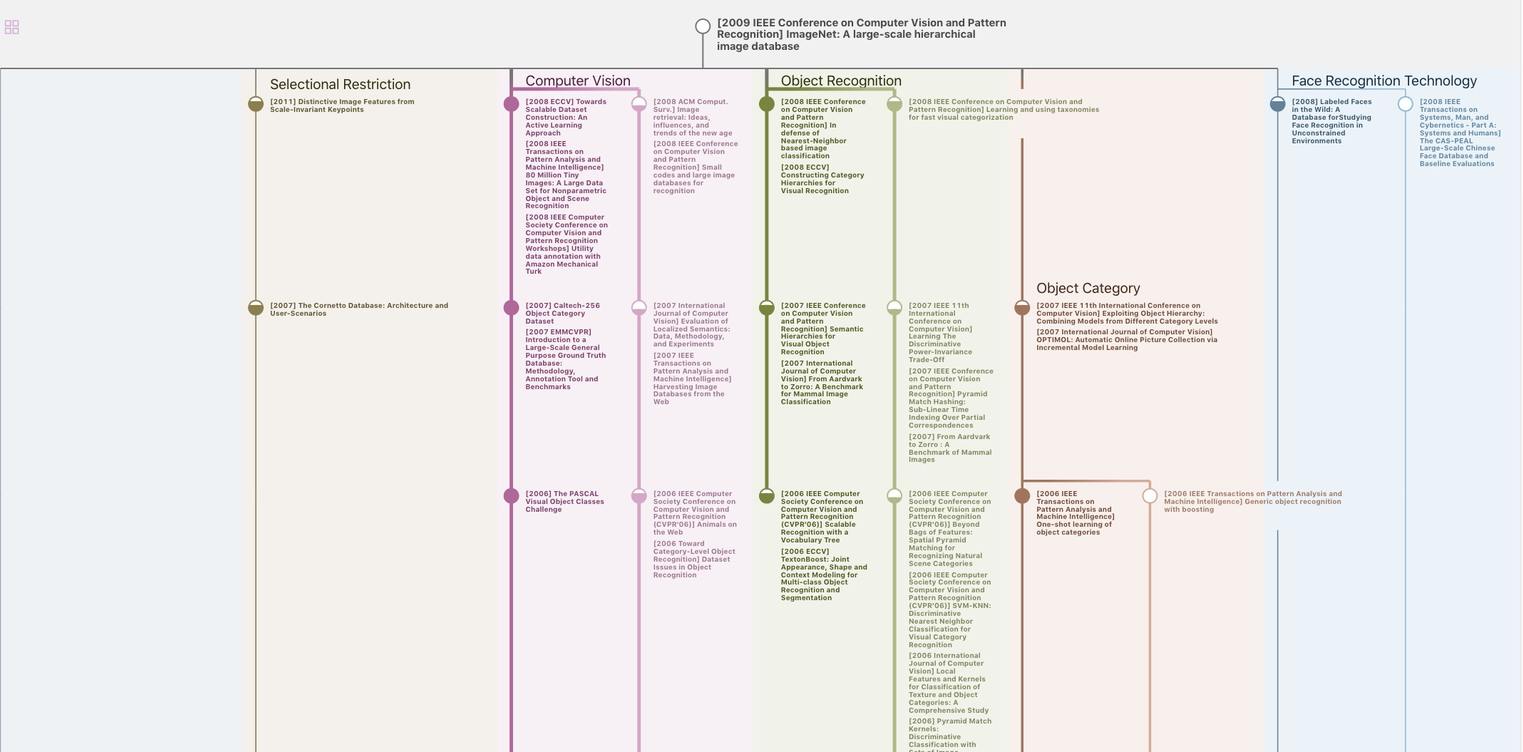
生成溯源树,研究论文发展脉络
Chat Paper
正在生成论文摘要