Local–Global Decompositions for Conditional Microstructure Generation
Acta Materialia(2023)
摘要
Conditional microstructure generation tools offer an important, inexpensive pathway to constructing statistically diverse datasets for Integrated Computational Materials Engineering and Materials Informatics efforts. To provide this utility in practice, an ideal generative framework must be able to efficiently, systematically, and robustly generate microstructures corresponding to selected spatial statistics (e.g., 1- and 2-point statistics) while also producing realistic local features (e.g., shapes and sizes of individual phase constituents). Because of the austerity of these requirements, generative frameworks often target either statistical conditioning or visual realism, but not both. In this paper, we propose to bridge these two approaches by approximating a microstructure’s generating process (i.e., its stochastic microstructure function) using a two layer semi-directed probabilistic graphical model. The first layer – a Gaussian Random Field (GRF) – provides direct control of the 1- and 2-point statistics. The second layer – a Score Based Generative Deep Learning model – postprocesses GRF predictions to refine local features while preserving global patterning. To understand and evaluate our proposed framework, we apply it to generate statistically equivalent N-phase microstructures from experimental references, including a 2-Phase Nickel-based Super Alloy and a 3-phase α−β Titanium alloy. Through these two case studies, we demonstrate that our framework successfully matches both lower-order (1- and 2-point statistics) and several salient higher-order statistics. Additionally, we briefly explore the capacity of these models to extrapolate outside of their training data by varying the input 2-point statistics. We discuss the value of this ability towards systematically generating diverse microstructure datasets.
更多查看译文
关键词
conditional microstructure generation,local–global decompositions
AI 理解论文
溯源树
样例
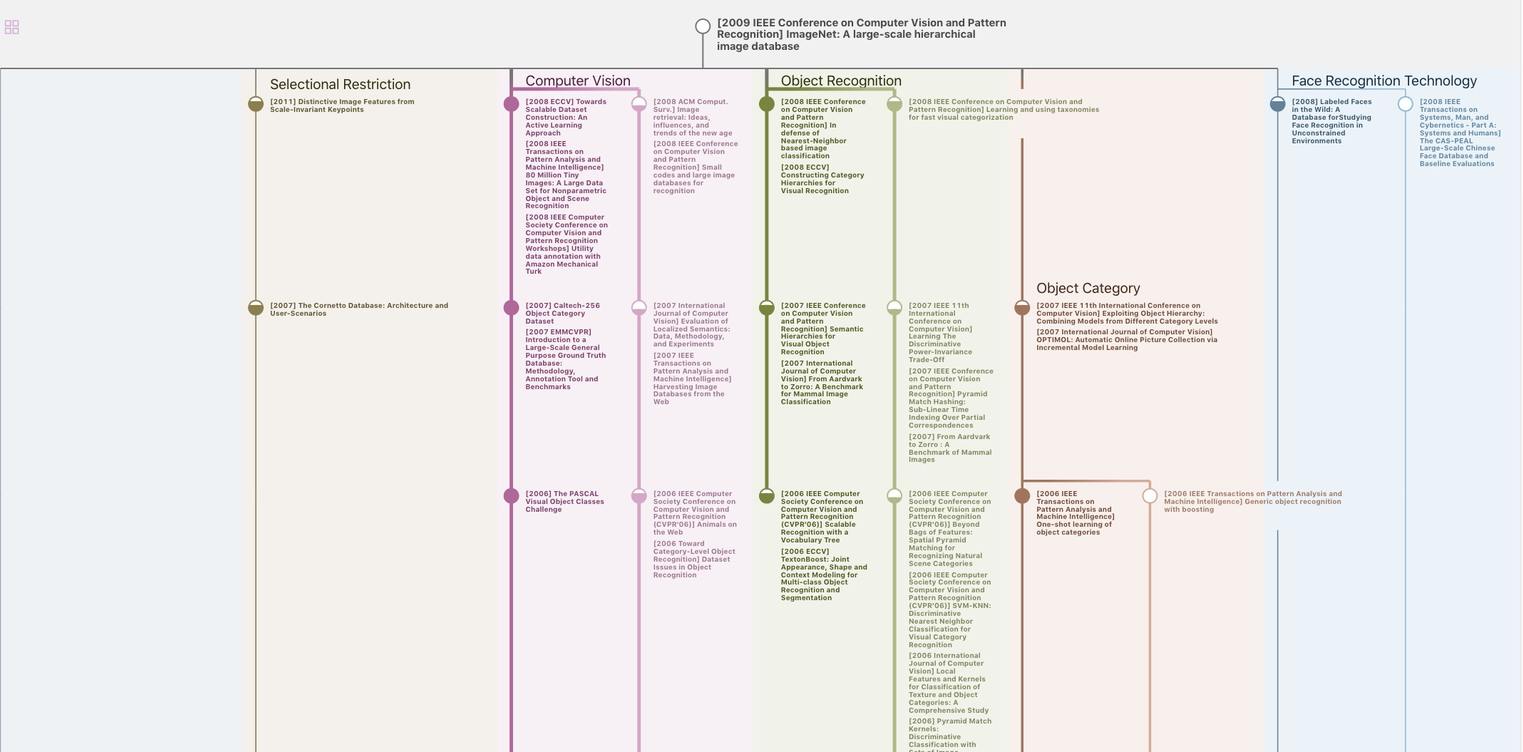
生成溯源树,研究论文发展脉络
Chat Paper
正在生成论文摘要