Fourier-Net+: Leveraging Band-Limited Representation for Efficient 3D Medical Image Registration
CoRR(2023)
摘要
U-Net style networks are commonly utilized in unsupervised image registration to predict dense displacement fields, which for high-resolution volumetric image data is a resource-intensive and time-consuming task. To tackle this challenge, we first propose Fourier-Net, which replaces the costly U-Net style expansive path with a parameter-free model-driven decoder. Instead of directly predicting a full-resolution displacement field, our Fourier-Net learns a low-dimensional representation of the displacement field in the band-limited Fourier domain which our model-driven decoder converts to a full-resolution displacement field in the spatial domain. Expanding upon Fourier-Net, we then introduce Fourier-Net+, which additionally takes the band-limited spatial representation of the images as input and further reduces the number of convolutional layers in the U-Net style network's contracting path. Finally, to enhance the registration performance, we propose a cascaded version of Fourier-Net+. We evaluate our proposed methods on three datasets, on which our proposed Fourier-Net and its variants achieve comparable results with current state-of-the art methods, while exhibiting faster inference speeds, lower memory footprint, and fewer multiply-add operations. With such small computational cost, our Fourier-Net+ enables the efficient training of large-scale 3D registration on low-VRAM GPUs. Our code is publicly available at \url{https://github.com/xi-jia/Fourier-Net}.
更多查看译文
关键词
efficient 3d
AI 理解论文
溯源树
样例
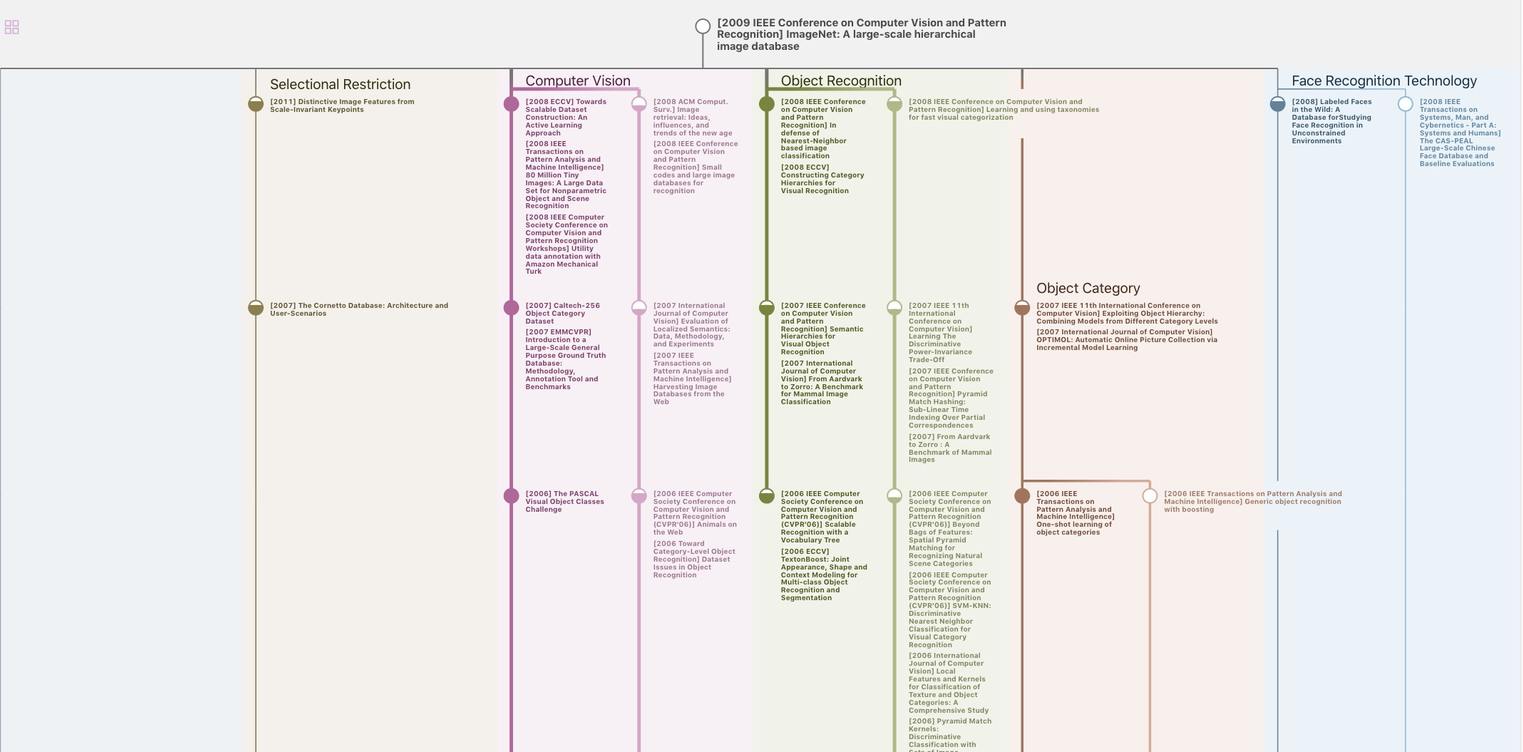
生成溯源树,研究论文发展脉络
Chat Paper
正在生成论文摘要