Multi-modal Multi-class Parkinson Disease Classification Using CNN and Decision Level Fusion
PATTERN RECOGNITION AND MACHINE INTELLIGENCE, PREMI 2023(2023)
摘要
Parkinson's disease (PD) is the second most common neurodegenerative disorder, as reported by theWorld Health Organization (WHO). In this paper, we propose a direct three-Class PD classification using two different modalities, namely, MRI and DTI. The three classes used for classification are PD, Scans Without Evidence of Dopamine Deficit (SWEDD) and Healthy Control (HC). We use white matter (WM) and gray matter (GM) from the MRI and fractional anisotropy (FA) and mean diffusivity (MD) from the DTI to achieve our goal. We train four separate CNNs on the above four types of data. At the decision level, the outputs of the four CNN models are fused with an optimal weighted average fusion technique. We achieve an accuracy of 95.53% for the direct three-class classification of PD, HC and SWEDD on the publicly available PPMI database. Extensive comparisons including a series of ablation studies clearly demonstrate the effectiveness of our proposed solution.
更多查看译文
关键词
Parkinson's disease (PD),Direct three-Class classification,Multi-modal Data,Decision level fusion
AI 理解论文
溯源树
样例
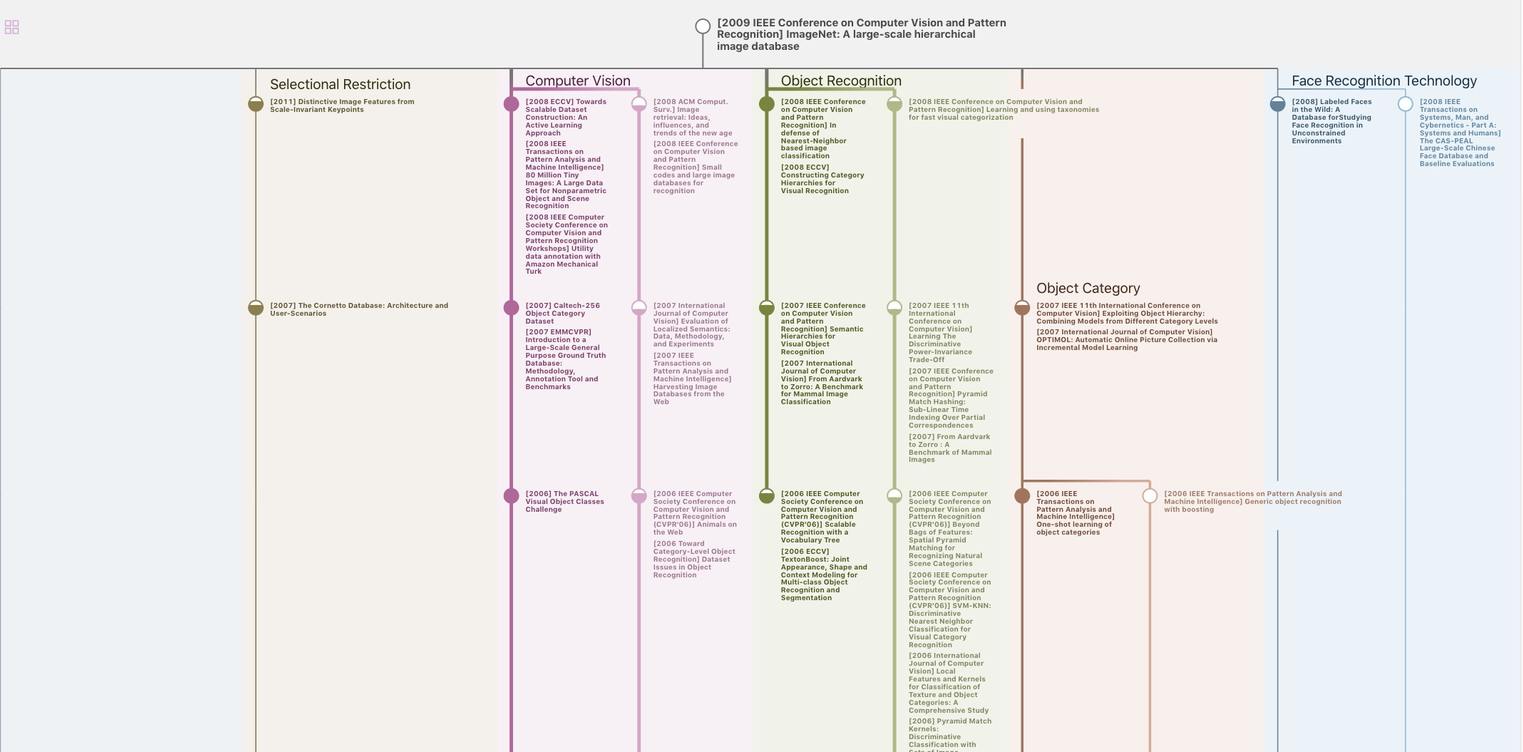
生成溯源树,研究论文发展脉络
Chat Paper
正在生成论文摘要