Stochastic Approximation for Expectation Objective and Expectation Inequality-Constrained Nonconvex Optimization
arXiv (Cornell University)(2023)
摘要
Stochastic Approximation has been a prominent set of tools for solving problems with noise and uncertainty. Increasingly, it becomes important to solve optimization problems wherein there is noise in both a set of constraints that a practitioner requires the system to adhere to, as well as the objective, which typically involves some empirical loss. We present the first stochastic approximation approach for solving this class of problems using the Ghost framework of incorporating penalty functions for analysis of a sequential convex programming approach together with a Monte Carlo estimator of nonlinear maps. We provide almost sure convergence guarantees and demonstrate the performance of the procedure on some representative examples.
更多查看译文
关键词
expectation objective,optimization,inequality-constrained
AI 理解论文
溯源树
样例
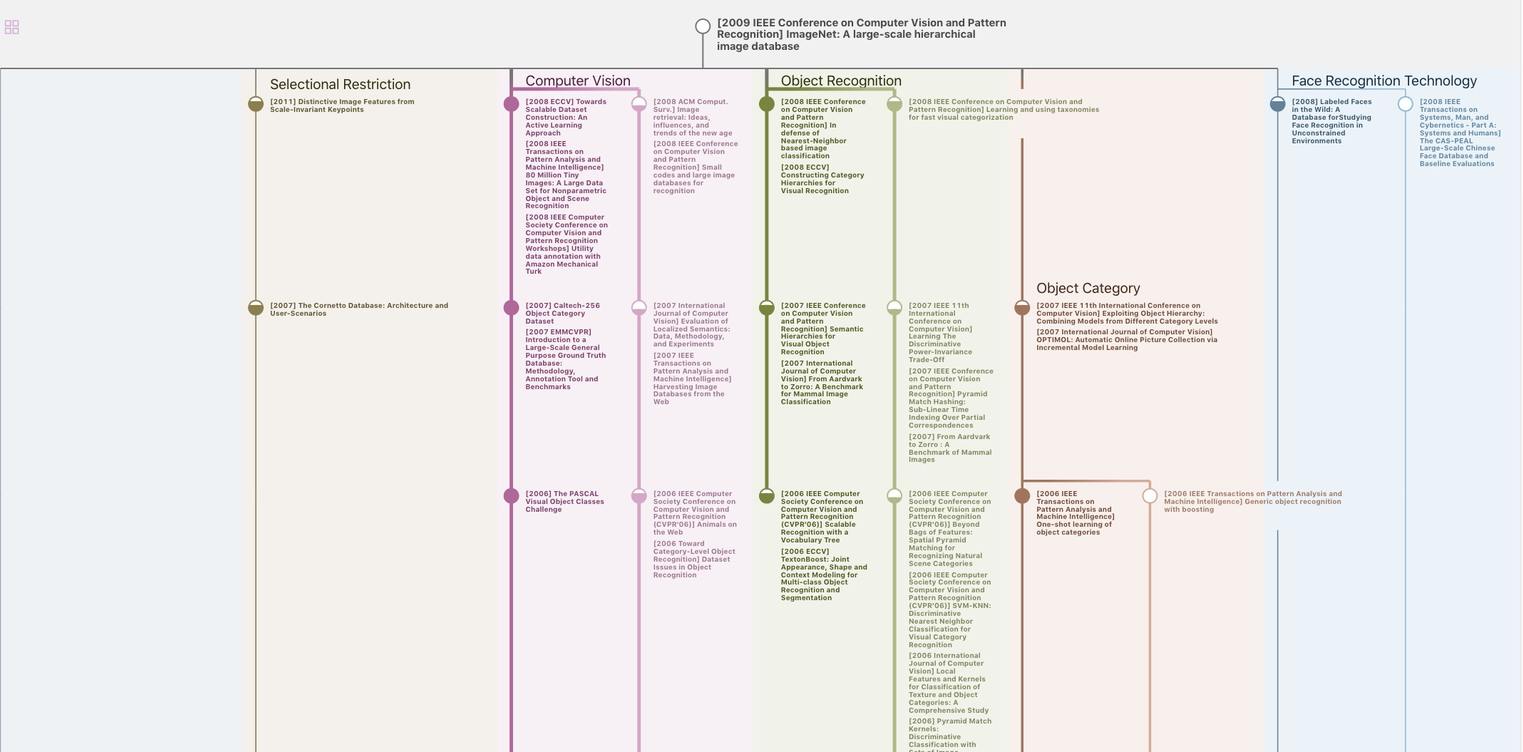
生成溯源树,研究论文发展脉络
Chat Paper
正在生成论文摘要