Hierarchical Empowerment: Towards Tractable Empowerment-Based Skill-Learning
CoRR(2023)
摘要
General purpose agents will require large repertoires of skills. Empowerment -- the maximum mutual information between skills and the states -- provides a pathway for learning large collections of distinct skills, but mutual information is difficult to optimize. We introduce a new framework, Hierarchical Empowerment, that makes computing empowerment more tractable by integrating concepts from Goal-Conditioned Hierarchical Reinforcement Learning. Our framework makes two specific contributions. First, we introduce a new variational lower bound on mutual information that can be used to compute empowerment over short horizons. Second, we introduce a hierarchical architecture for computing empowerment over exponentially longer time scales. We verify the contributions of the framework in a series of simulated robotics tasks. In a popular ant navigation domain, our four level agents are able to learn skills that cover a surface area over two orders of magnitude larger than prior work.
更多查看译文
关键词
learning,skill
AI 理解论文
溯源树
样例
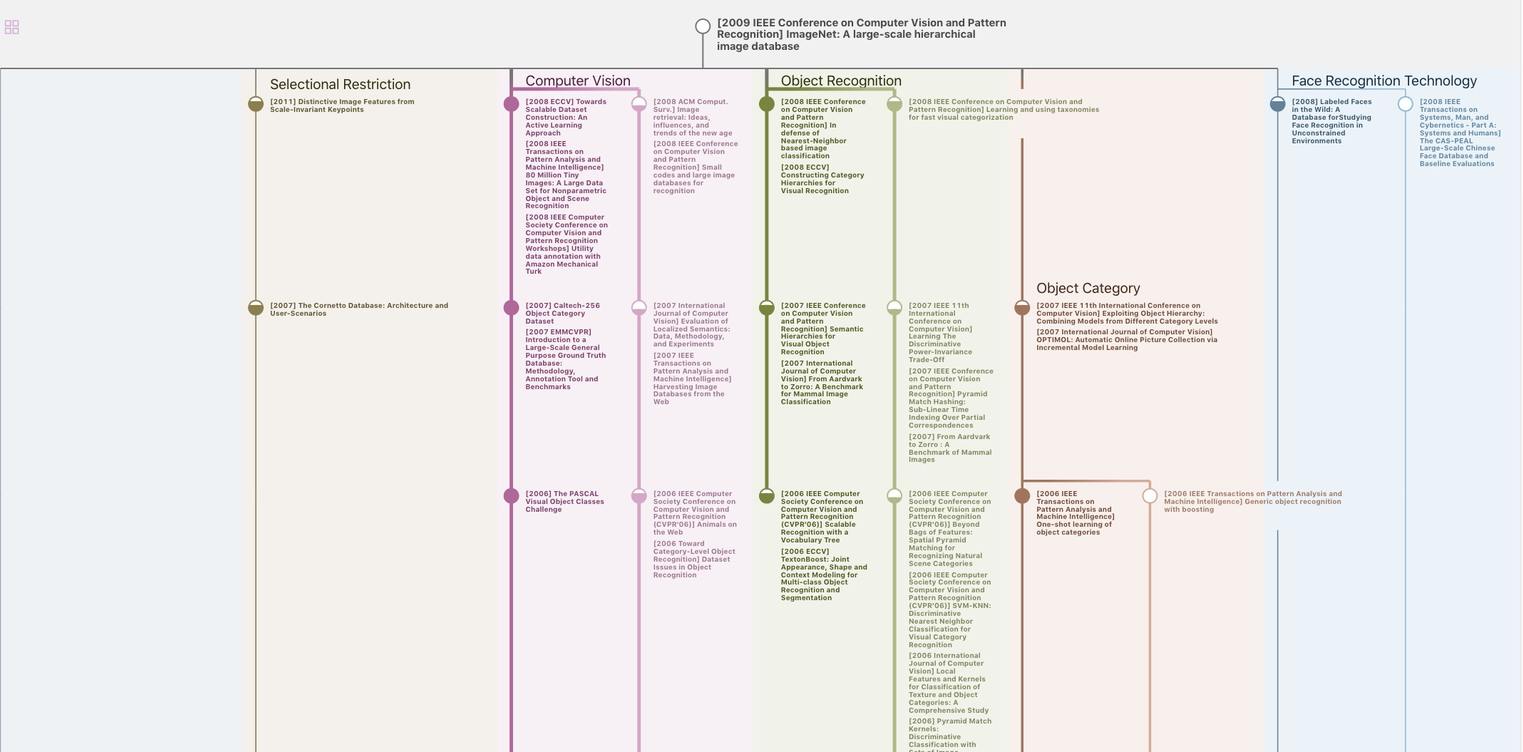
生成溯源树,研究论文发展脉络
Chat Paper
正在生成论文摘要