Exploring new ways: Enforcing representational dissimilarity to learn new features and reduce error consistency
CoRR(2023)
摘要
Independently trained machine learning models tend to learn similar features. Given an ensemble of independently trained models, this results in correlated predictions and common failure modes. Previous attempts focusing on decorrelation of output predictions or logits yielded mixed results, particularly due to their reduction in model accuracy caused by conflicting optimization objectives. In this paper, we propose the novel idea of utilizing methods of the representational similarity field to promote dissimilarity during training instead of measuring similarity of trained models. To this end, we promote intermediate representations to be dissimilar at different depths between architectures, with the goal of learning robust ensembles with disjoint failure modes. We show that highly dissimilar intermediate representations result in less correlated output predictions and slightly lower error consistency, resulting in higher ensemble accuracy. With this, we shine first light on the connection between intermediate representations and their impact on the output predictions.
更多查看译文
关键词
new features,representational dissimilarity,consistency,new ways
AI 理解论文
溯源树
样例
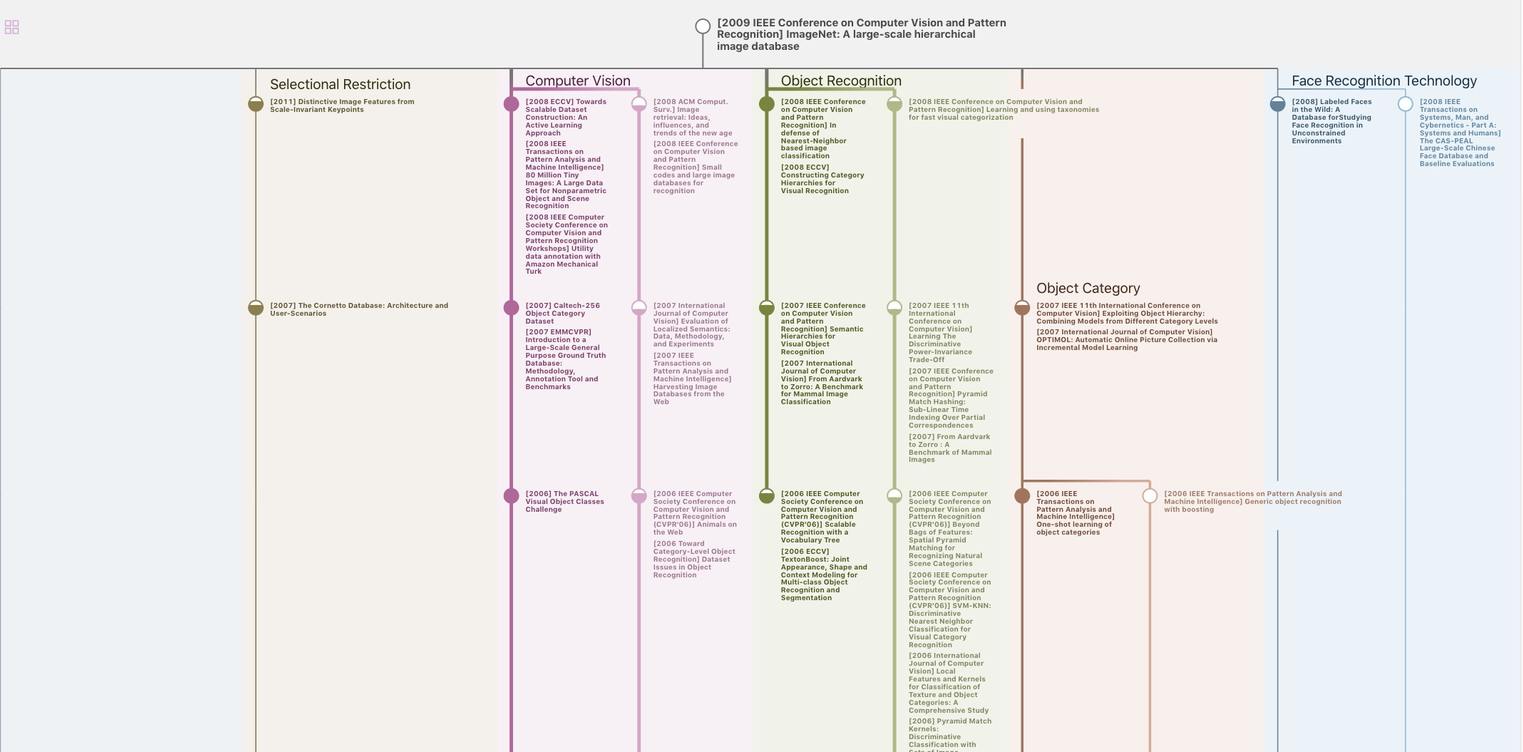
生成溯源树,研究论文发展脉络
Chat Paper
正在生成论文摘要