A Spasticity Assessment Method for Voluntary Movement using Data Fusion and Machine Learning
Research Square (Research Square)(2020)
摘要
Abstract Background: Spasticity is a common complication of stroke. Effective spasticity management can improve patients' recovery efficiency and reduce patients' pain. The present clinical spasticity rating scale exhibits subjectivity and a ceiling effect, which makes it difficult to evaluate spasm objectively and to clinically analyze the pathological mechanism of spasticity. The sensor-based quantitative evaluation method is an effective substitute for the clinical spasm rating scale, but currently, it mainly focuses on the spasm evaluation of passive motion. The study of spasmodic state under active exercise can provide a basis for treatment and rehabilitation training, but the evaluation method of spasmodic state under active exercise has not yet been established. Therefore, we combine inertial measurement unit (IMU) and surface electromyography (sEMG) to test the feasibility of assessing spasticity patterns in stroke patients during voluntary movement. Methods: Nine stroke patients with varying degrees of spasticity and four healthy subjects performed isometric elbow exercises. sEMG and kinematics signals were recorded for all participants. The Empirical Mode Decomposition (EMD) algorithm and double threshold algorithms were used to separate sEMG of involuntary muscle activation from voluntary activation. Then, feature extraction and feature fusion were performed. Four common machine learning algorithms are used to monitor and evaluate spasticity patterns. The validity of the proposed method is verified by comparing the classification accuracy of four machine learning models. Results: Cross-validation yielded high classification accuracies (F1-score>0.88) for all four machine learning classifiers in assessing spasticity patterns. The highest detection performance was obtained using the Random Forest algorithm (average accuracy = 0.979; macro-F1 = 0.976). Conclusions: We present a novel method for assessing post-stroke spasticity based on voluntary movement and machine learning. Good classification performance verifies the feasibility of evaluating spasticity patterns by our method. Reliable classification accuracy achieved by the machine learning algorithms indicated the potential to evaluate spasticity patterns using IMU and sEMG when stroke survivors perform voluntary movements.
更多查看译文
关键词
spasticity assessment method,data fusion,voluntary movement,machine learning
AI 理解论文
溯源树
样例
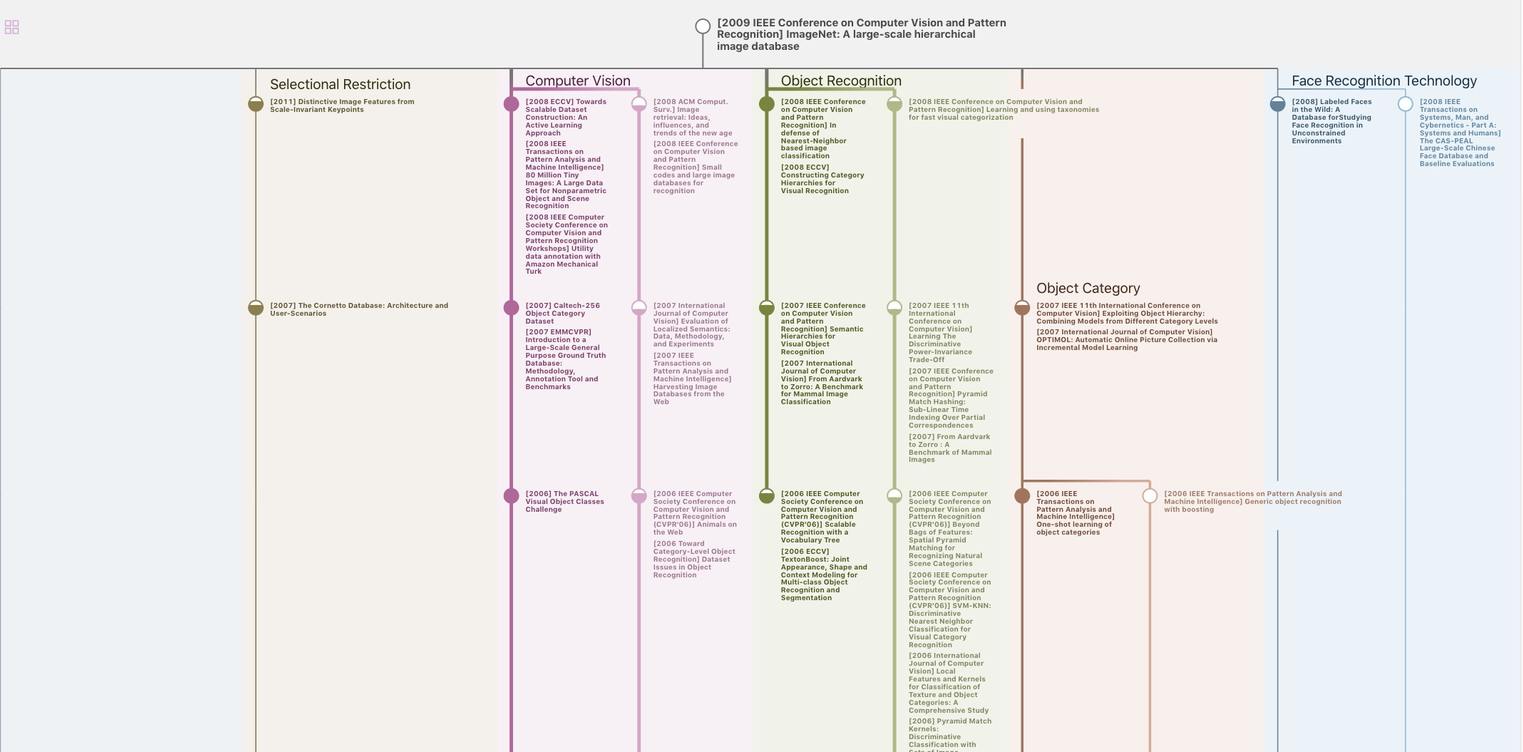
生成溯源树,研究论文发展脉络
Chat Paper
正在生成论文摘要