Machine Learning for Predicting Pathological Complete Response in Patients with Locally Advanced Rectal Cancer after Neoadjuvant Chemoradiotherapy
crossref(2020)
摘要
Abstract BACKGROUND For patients with locally advanced rectal cancer (LARC), achieving pathological complete response (pCR) after neoadjuvant chemoradiotherapy (CRT) results in best prognosis. So far, no reliable prediction model has been available. We aim to evaluate the performance of an artificial neural network (ANN) model in the prediction of pCR in patients with LARC. METHODS Predictive accuracy was compared between the ANN, k-earest neighbor (KNN), support vector machines (SVM), naïve Bayes classifier (NBC), and multiple logistic regression (MLR) models. RESULTS A total of 236 patients with LARC were used to compare the forecasting models. We trained the model with an estimation data set, and evaluated model performance with a validation data set. The ANN model significantly outperformed the KNN, SVM, NBC, and MLR models in predicting pCR. Our results revealed that post-CRT carcinoembryonic antigen was the most influential predictor of pCR, followed by intervals between CRT and surgery, chemotherapy regimens, clinical nodal stage, and clinical tumor stage. CONCLUSIONS Compared with conventional prediction models, the ANN model was more accurate in the prediction of pCR. The predictors of pCR can be used to identify which patients with LARC can benefit from watch-and-wait approaches.
更多查看译文
AI 理解论文
溯源树
样例
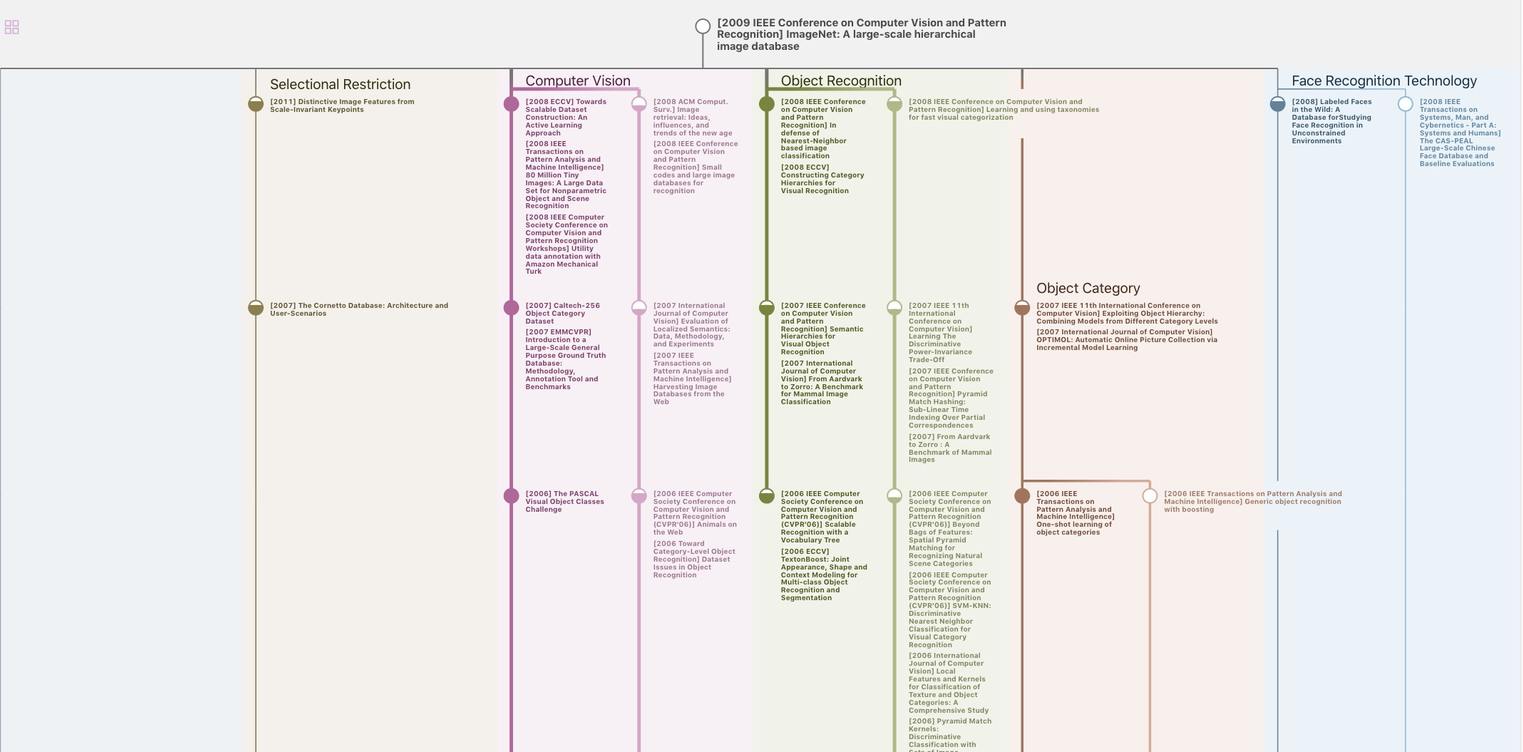
生成溯源树,研究论文发展脉络
Chat Paper
正在生成论文摘要