Machine Learning for Pattern Detection in Cochlear Implant FDA Adverse Event Reports
medRxiv (Cold Spring Harbor Laboratory)(2020)
摘要
ImportanceThe United States Food & Drug Administration (FDA) passively monitors medical device performance and safety through submitted medical device reports (MDRs) in the Manufacturer and User Facility Device Experience (MAUDE) database. These databases can be analyzed for patterns and novel opportunities for improving patient safety and/or device design.ObjectivesThe objective of this analysis was to use supervised machine learning to explore patterns in reported adverse events involving cochlear implants.DesignThe MDRs for the top three CI manufacturers by volume from January 1st 2009 to August 30th 2019 were retained for the analysis. Natural language processing was used to measure the importance of specific words. Four supervised machine learning algorithms were used to predict which adverse event narrative description pattern corresponded with a specific cochlear implant manufacturer and adverse event type - injury, malfunction, or death.SettingU.S. government public database.ParticipantsAdult and pediatric cochlear patients.ExposureSurgical placement of a cochlear implant.Main Outcome MeasureMachine learning model classification prediction accuracy (% correct predictions).Results27,511 adverse events related to cochlear implant devices were submitted to the MAUDE database during the study period. Most adverse events involved patient injury (n = 16,736), followed by device malfunction (n = 10,760), and death (n = 16). Submissions to the database were dominated by Cochlear Corporation (n = 13,897), followed by MedEL (n = 7,125), and Advanced Bionics (n = 6,489). The random forest, linear SVC, naïve Bayes and logistic algorithms were able to predict the specific CI manufacturer based on the adverse event narrative with an average accuracy of 74.8%, 86.0%, 88.5% and 88.6%, respectively.Conclusions & RelevanceUsing supervised machine learning algorithms, our classification models were able to predict the CI manufacturer and event type with high accuracy based on patterns in adverse event text descriptions.Level of evidence3
更多查看译文
关键词
machine learning,pattern detection
AI 理解论文
溯源树
样例
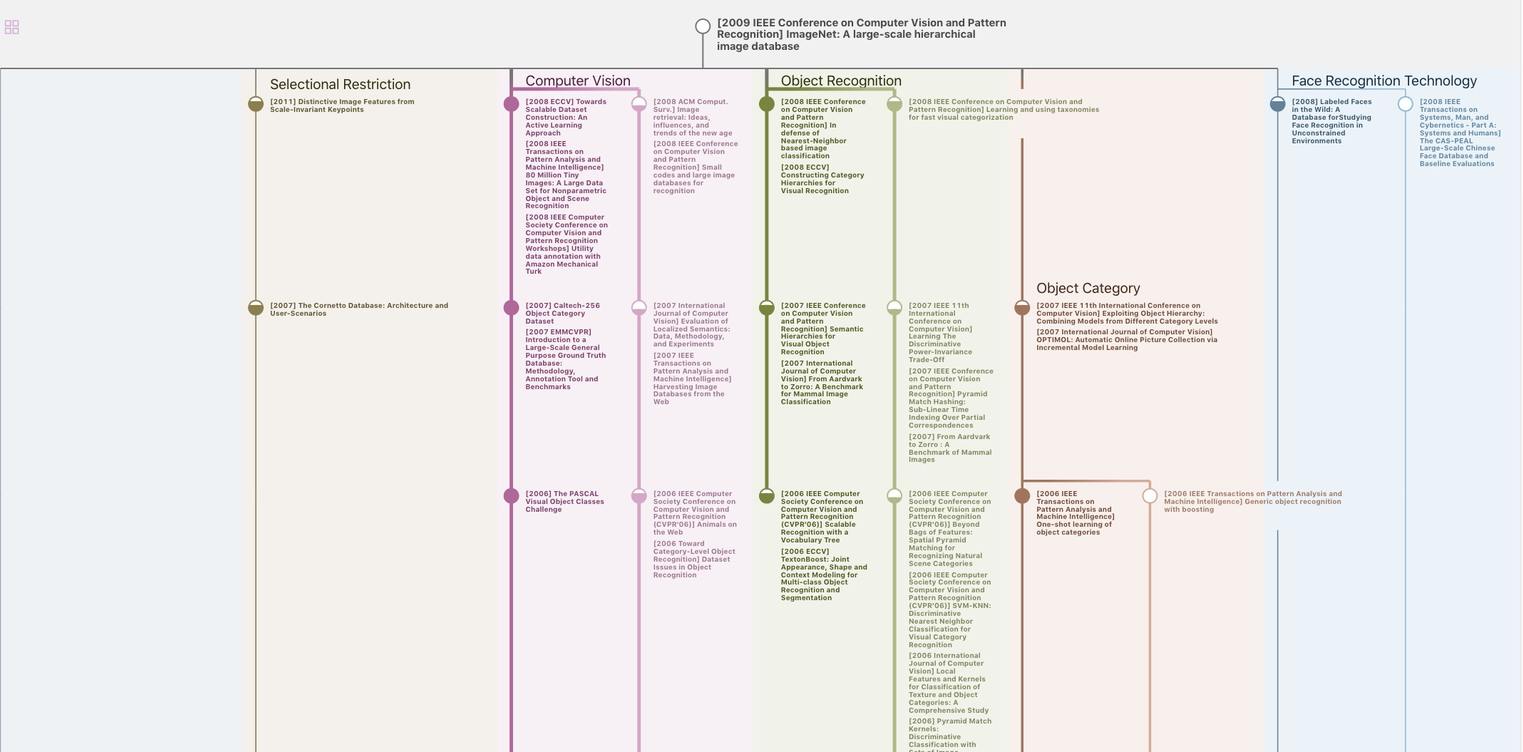
生成溯源树,研究论文发展脉络
Chat Paper
正在生成论文摘要