Improving Hospital Readmission Prediction using Individualized Utility Analysis
crossref(2020)
摘要
Structured AbstractImportanceMachine learning (ML) models for allocating readmission-mitigating interventions are typically selected according to their discriminative ability, which may not necessarily translate into utility in allocation of resources.ObjectiveTo determine whether ML models for allocating readmission-mitigating interventions are ranked differently based on their overall utility and their discriminative ability.DesignA retrospective analysis of ML models using claims data acquired from the Optum Clinformatics Data Mart.SettingHealth plan claims from all 50 states for commercially-insured individuals.Participants513,495 patients who were admitted as inpatients over the period January 2016 through January 2017.Main Outcomes and MeasuresMaximum utility achieved by three machine learning models for allocating readmission-mitigating interventions, determined using cost accrued in the 90 days post-discharge of an index admission and estimated counterfactual cost. Data were analyzed between April 2019 and March 2020.ResultsThe study sample consisted of 513,495 patients (mean [SD] age 69 [19] years; 294,895 [57%] Female) mean 90 day cost of $11,552 for the study period. Allocating readmission-mitigating interventions based on a LightGBM model trained to predict readmissions achieved a maximum utility of $-12,645 per patient, and an AUC of 0.74 (95% CI 0.74, 0.75); allocating interventions based on a model trained to predict cost as a proxy achieved a higher maximum utility of $-12,472 per patient, and an AUC of 0.63 (95% CI 0.62, 0.63). A hybrid model combining both intervention strategies achieved a maximum utility of $-12,472, and an AUC of 0.71 (95% CI 0.71, 0.71), comparable with the best models on either metric.Conclusion and RelevanceWe demonstrate that machine learning models may be ranked differently based on overall utility and discriminative ability. Machine learning models for allocation of limited health resources should consider directly optimizing for utility.Key pointsQuestionDo machine learning models for allocating readmission-mitigating interventions rank differently based on overall utility and discriminative performance?FindingA machine learning model predicting a patient’s future cost of care was able to achieve higher utility than a readmission risk prediction model, even though it had a lower discriminative performance in predicting readmissions.MeaningOur study suggests that machine learning models guiding allocation of limited health resources may consider evaluating and optimizing on utility.
更多查看译文
AI 理解论文
溯源树
样例
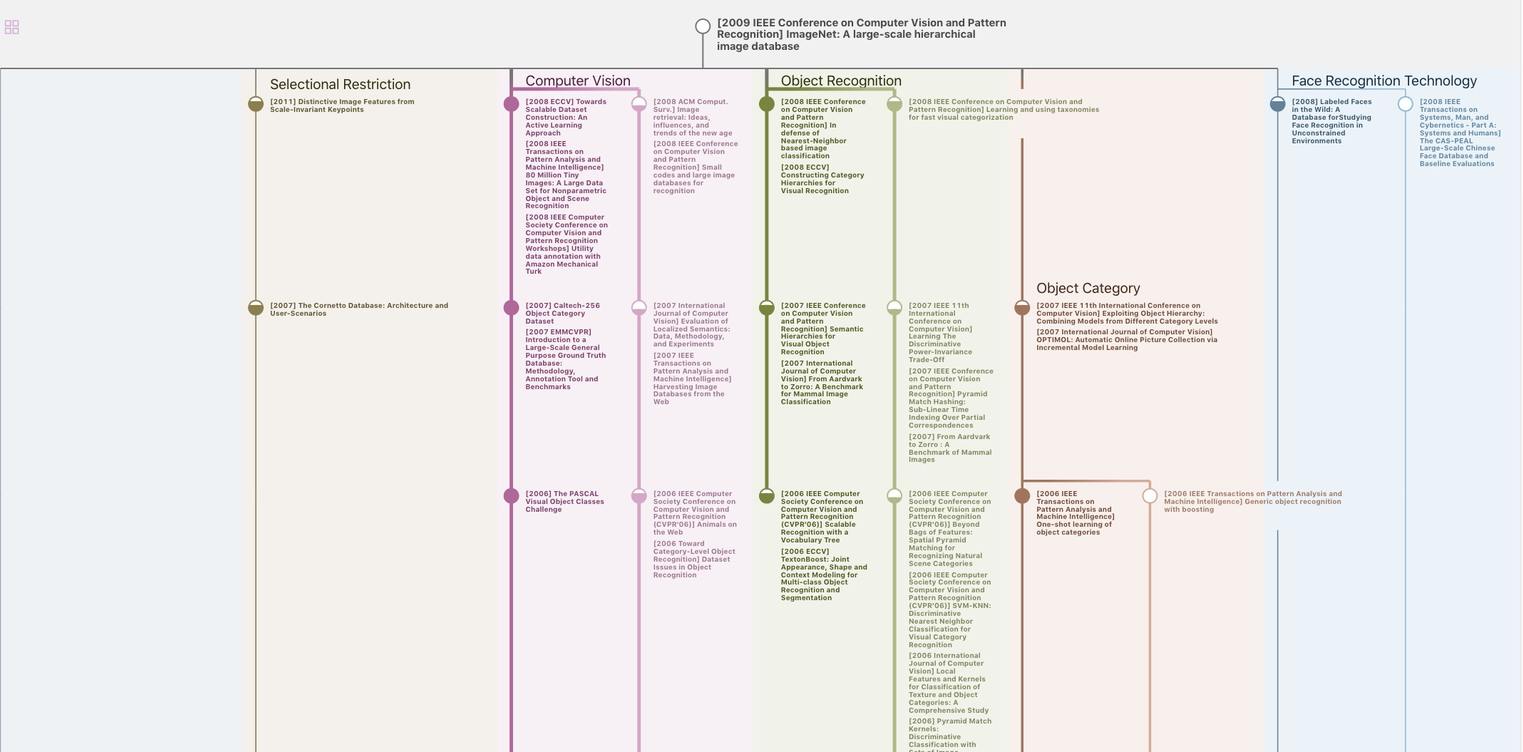
生成溯源树,研究论文发展脉络
Chat Paper
正在生成论文摘要