Development and external validation of machine learning algorithms for postnatal gestational age estimation using clinical data and metabolomic markers
Carolina Digital Repository (University of North Carolina at Chapel Hill)(2020)
摘要
AbstractAccurate estimates of gestational age at birth are important for preterm birth surveillance but can be challenging to reliably acquire in low and middle income countries. Our objective was to develop machine learning models to accurately estimate gestational age shortly after birth using clinical and metabolic data. We derived and internally validated three models using ELASTIC NET multivariable linear regression in heel prick blood samples and clinical data from a retrospective cohort of newborns from Ontario, Canada. We conducted external model validation in heel prick and cord blood sample data collected from prospective birth cohorts in Lusaka, Zambia (N=311) and Matlab, Bangladesh (N=1176). The best-performing model accurately estimated gestational age within about 6 days of early pregnancy ultrasound estimates in both cohorts when applied to heel prick data (MAE (95% CI) = 0.79 weeks (0.69, 0.90) for Zambia; 0.81 weeks (0.75, 0.86) for Bangladesh), and within about 7 days when applied to cord blood data (1.02 weeks (0.90, 1.15) for Zambia; 0.95 weeks (0.90, 0.99) for Bangladesh). Algorithms developed in Canada provided accurate estimates of gestational age when applied to external cohorts from Zambia and Bangladesh. Model performance was superior in heel prick data as compared to cord blood data.
更多查看译文
关键词
postnatal gestational age estimation,machine learning,clinical data
AI 理解论文
溯源树
样例
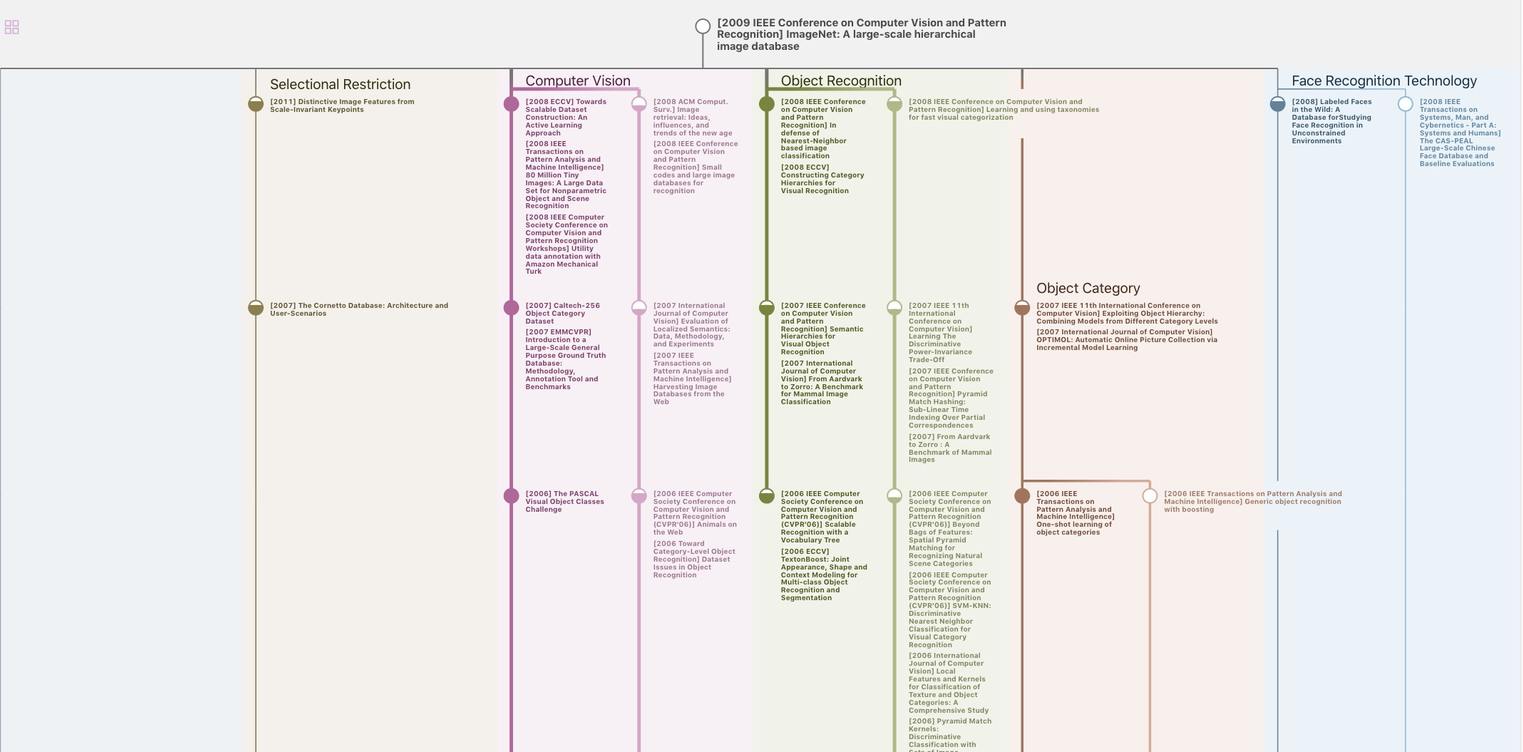
生成溯源树,研究论文发展脉络
Chat Paper
正在生成论文摘要