Using Rewrite Strategies for Efficient Functional Automatic Differentiation
PROCEEDINGS OF THE 25TH ACM INTERNATIONAL WORKSHOP ON FORMAL TECHNIQUES FOR JAVA-LIKE PROGRAMS, FTFJP 2023(2023)
摘要
Automatic Differentiation (AD) has become a dominant technique in ML. AD frameworks have first been implemented for imperative languages using tapes. Meanwhile, functional implementations of AD have been developed, often based on dual numbers, which are close to the formal specification of differentiation and hence easier to prove correct. But these papers have focussed on correctness not efficiency. Recently, it was shown how an approach using dual numbers could be made efficient through the right optimizations. Optimizations are highly dependent on order, as one optimization can enable another. It can therefore be useful to have fine-grained control over the scheduling of optimizations. One method expresses compiler optimizations as rewrite rules, whose application can be combined and controlled using strategy languages. Previous work describes the use of term rewriting and strategies to generate high-performance code in a compiler for a functional language. In this work, we implement dual numbers AD in a functional array programming language using rewrite rules and strategy combinators for optimization. We aim to combine the elegance of differentiation using dual numbers with a succinct expression of the optimization schedule using a strategy language. We give preliminary evidence suggesting the viability of the approach on a micro-benchmark.
更多查看译文
关键词
differentiable programming,domain-specific language,optimization,term rewriting
AI 理解论文
溯源树
样例
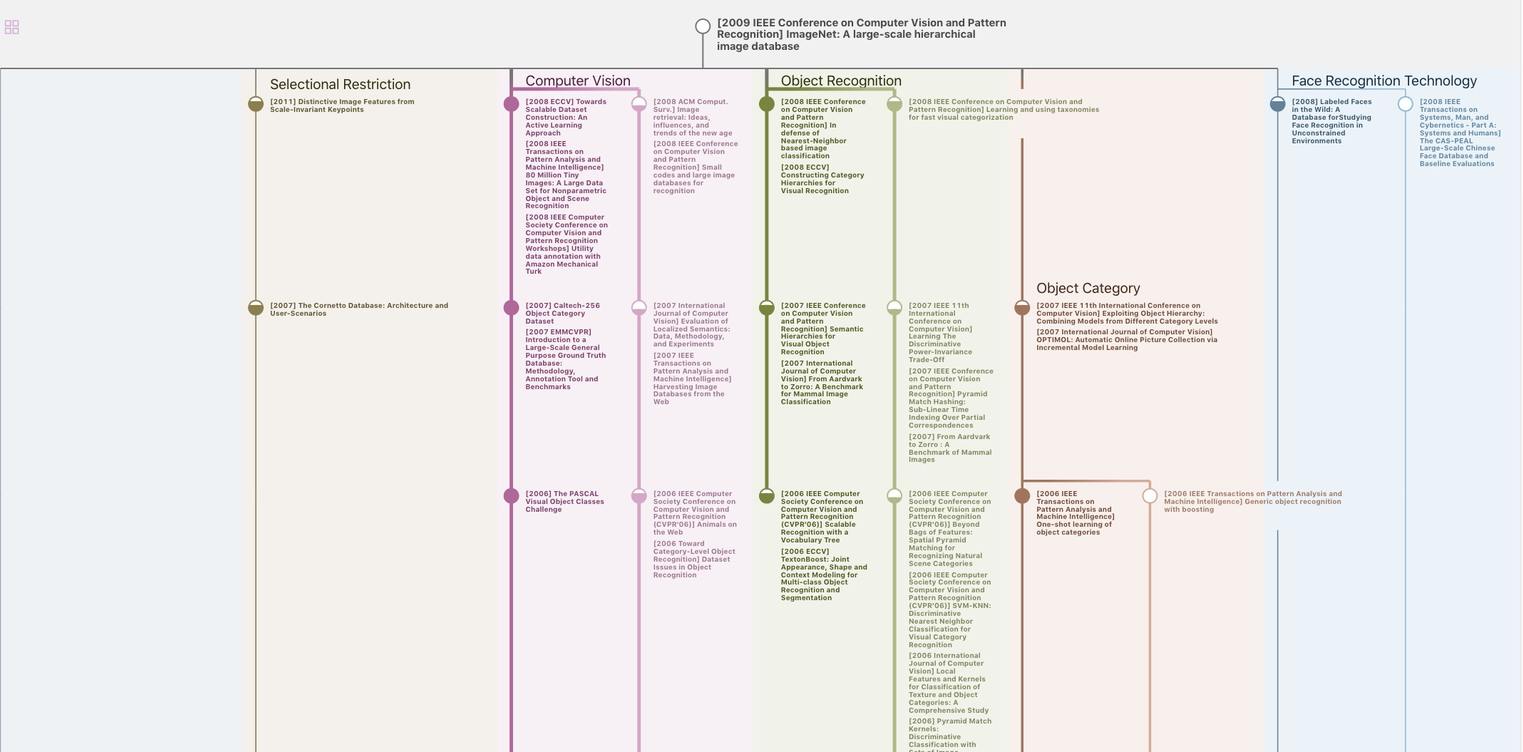
生成溯源树,研究论文发展脉络
Chat Paper
正在生成论文摘要