VertiBench: Advancing Feature Distribution Diversity in Vertical Federated Learning Benchmarks
arXiv (Cornell University)(2023)
摘要
Vertical Federated Learning (VFL) is a crucial paradigm for training machine
learning models on feature-partitioned, distributed data. However, due to
privacy restrictions, few public real-world VFL datasets exist for algorithm
evaluation, and these represent a limited array of feature distributions.
Existing benchmarks often resort to synthetic datasets, derived from arbitrary
feature splits from a global set, which only capture a subset of feature
distributions, leading to inadequate algorithm performance assessment. This
paper addresses these shortcomings by introducing two key factors affecting VFL
performance - feature importance and feature correlation - and proposing
associated evaluation metrics and dataset splitting methods. Additionally, we
introduce a real VFL dataset to address the deficit in image-image VFL
scenarios. Our comprehensive evaluation of cutting-edge VFL algorithms provides
valuable insights for future research in the field.
更多查看译文
关键词
federated learning benchmarks,federated learning,feature distribution diversity,vertibench
AI 理解论文
溯源树
样例
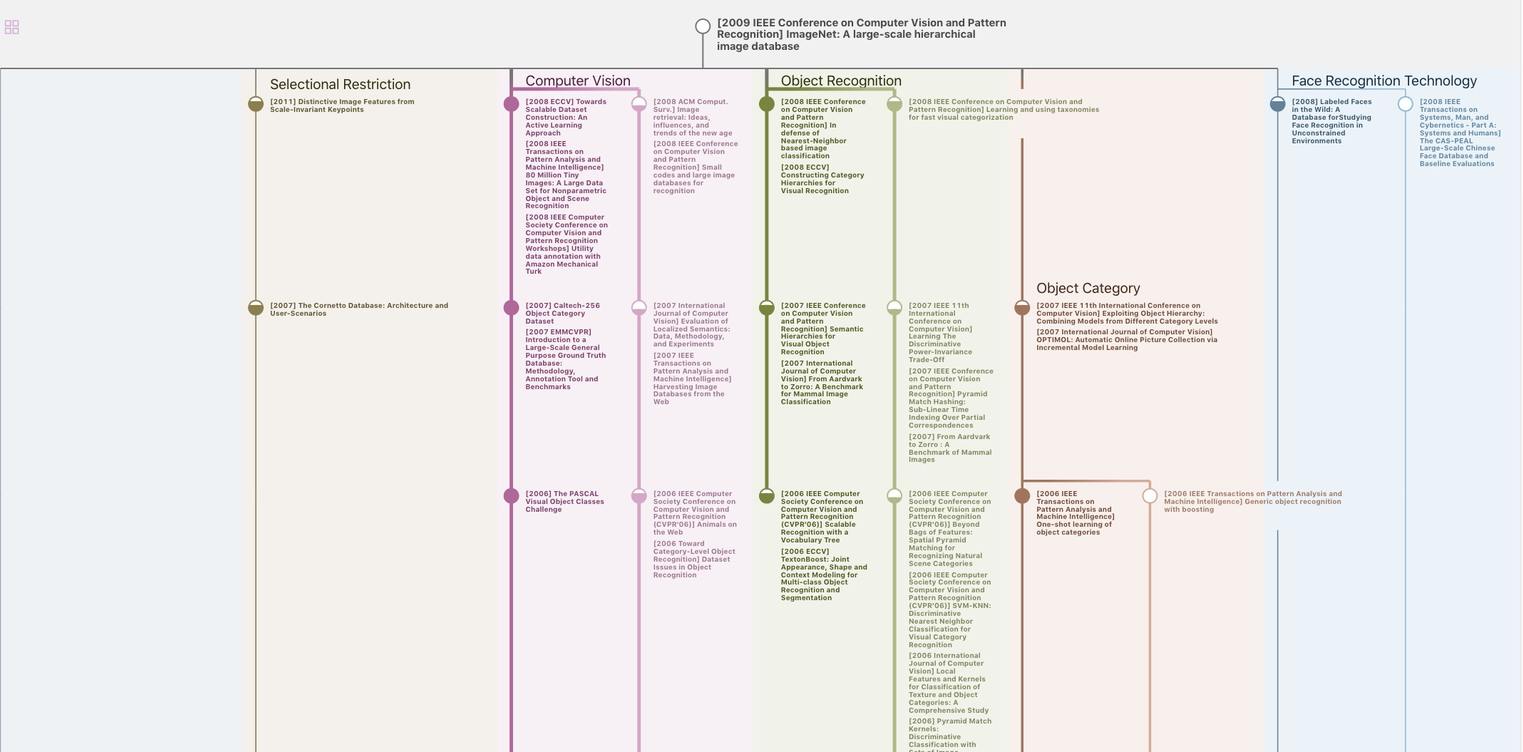
生成溯源树,研究论文发展脉络
Chat Paper
正在生成论文摘要