Diverse Retrieval-Augmented In-Context Learning for Dialogue State Tracking
conf_acl(2023)
摘要
There has been significant interest in zero and few-shot learning for dialogue state tracking (DST) due to the high cost of collecting and annotating task-oriented dialogues. Recent work has demonstrated that in-context learning requires very little data and zero parameter updates, and even outperforms trained methods in the few-shot setting (Hu et al. 2022). We propose RefPyDST, which advances the state of the art with three advancements to in-context learning for DST. First, we formulate DST as a Python programming task, explicitly modeling language coreference as variable reference in Python. Second, since in-context learning depends highly on the context examples, we propose a method to retrieve a diverse set of relevant examples to improve performance. Finally, we introduce a novel re-weighting method during decoding that takes into account probabilities of competing surface forms, and produces a more accurate dialogue state prediction. We evaluate our approach using MultiWOZ and achieve state-of-the-art multi-domain joint-goal accuracy in zero and few-shot settings.
更多查看译文
关键词
dialogue state
AI 理解论文
溯源树
样例
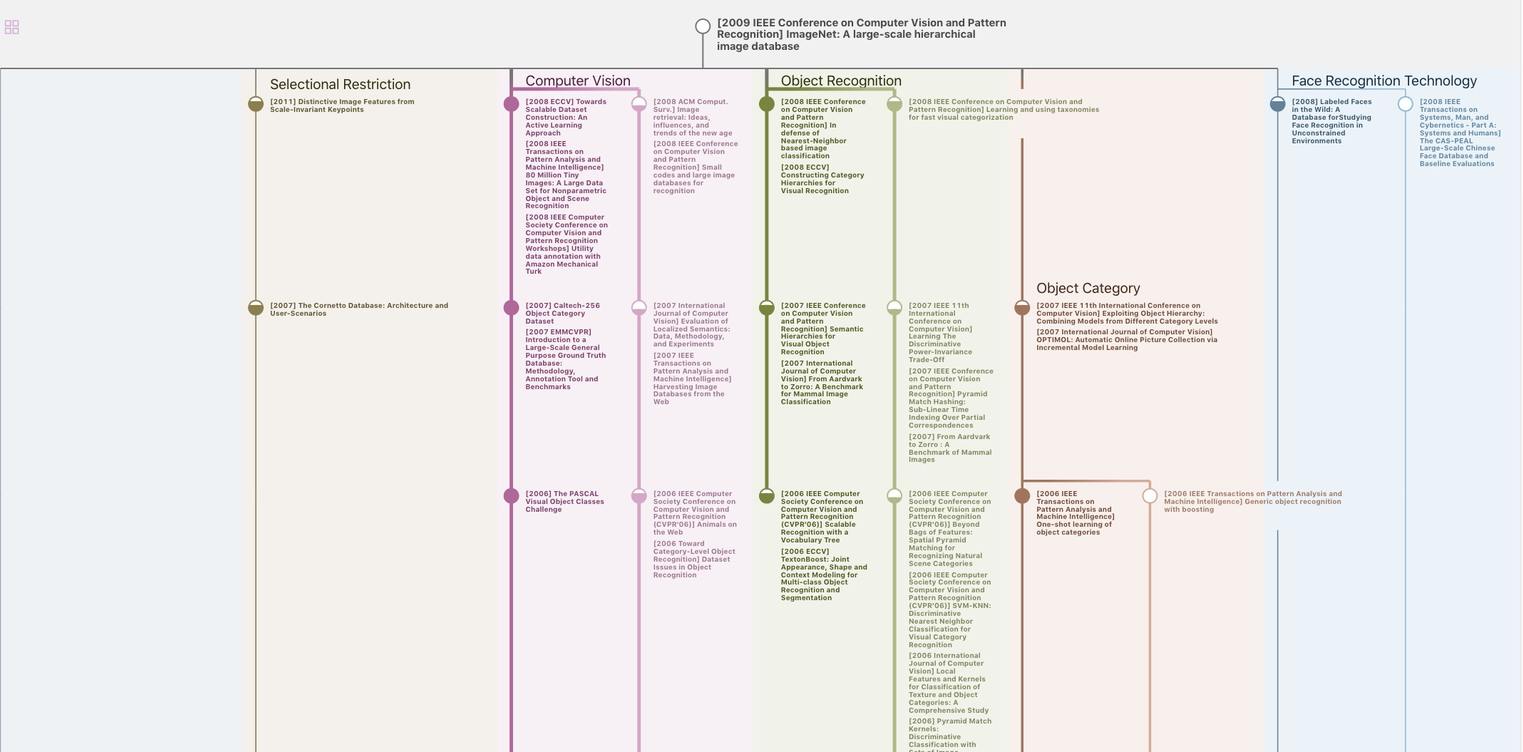
生成溯源树,研究论文发展脉络
Chat Paper
正在生成论文摘要